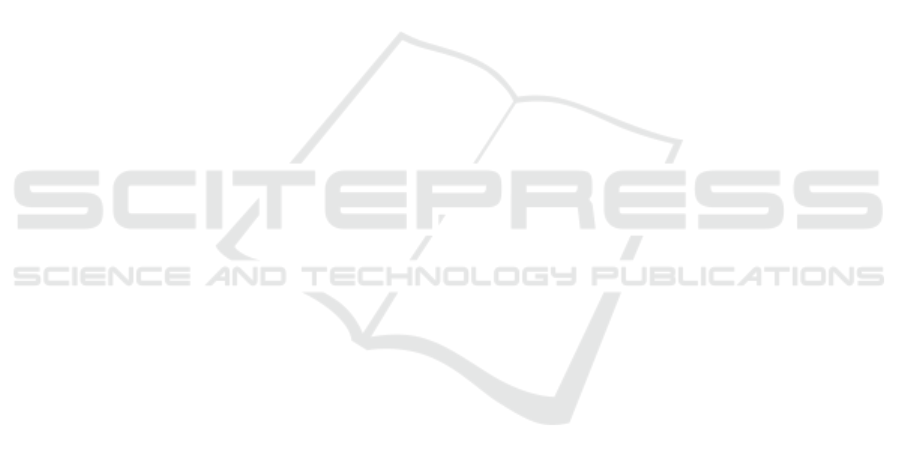
Domain generalisation for mitosis detection exploting
preprocessing homogenizers. In International Con-
ference on Medical Image Computing and Computer-
Assisted Intervention, pages 77–80. Springer.
Aubreville, M., Bertram, C., Veta, M., Klopfleisch, R.,
Stathonikos, N., Breininger, K., ter Hoeve, N.,
Ciompi, F., and Maier, A. (2021). Mitosis domain
generalization challenge. In 24th International Con-
ference on Medical Image Computing and Computer
Assisted Intervention (MICCAI 2021), pages 1–15.
Breen, J., Zucker, K., Orsi, N. M., and Ravikumar, N.
(2021). Assessing domain adaptation techniques
for mitosis detection in multi-scanner breast cancer
histopathology images. In International Conference
on Medical Image Computing and Computer-Assisted
Intervention, pages 14–22. Springer.
Choi, Y., Choi, M., Kim, M., Ha, J.-W., Kim, S., and Choo,
J. (2018). Stargan: Unified generative adversarial net-
works for multi-domain image-to-image translation.
In Proceedings of the IEEE conference on computer
vision and pattern recognition, pages 8789–8797.
Chung, Y., Cho, J., and Park, J. (2021). Domain-robust
mitotic figure detection with style transfer. In In-
ternational Conference on Medical Image Comput-
ing and Computer-Assisted Intervention, pages 23–
31. Springer.
Dexl, J., Benz, M., Bruns, V., Kuritcyn, P., and Wittenberg,
T. (2021). Mitodet: Simple and robust mitosis de-
tection. In International Conference on Medical Im-
age Computing and Computer-Assisted Intervention,
pages 53–57. Springer.
Everingham, M., Van Gool, L., Williams, C.
K. I., Winn, J., and Zisserman, A. The
PASCAL Visual Object Classes Challenge
2012 (VOC2012) Results. http://www.pascal-
network.org/challenges/VOC/voc2012/workshop/index.html.
Fick, R. H., Moshayedi, A., Roy, G., Dedieu, J., Petit,
S., and Hadj, S. B. (2021). Domain-specific cycle-
gan augmentation improves domain generalizability
for mitosis detection. In International Conference on
Medical Image Computing and Computer-Assisted In-
tervention, pages 40–47. Springer.
Ganin, Y. and Lempitsky, V. (2015). Unsupervised do-
main adaptation by backpropagation. In International
conference on machine learning, pages 1180–1189.
PMLR.
Girshick, R. (2015). Fast r-cnn. In Proceedings of the IEEE
international conference on computer vision, pages
1440–1448.
He, K., Gkioxari, G., Doll
´
ar, P., and Girshick, R. (2017).
Mask r-cnn. In Proceedings of the IEEE international
conference on computer vision, pages 2961–2969.
Howard, J., Thomas, R., and Gugger, S. (2018). fastai. Aval-
ableat: https://github. com/fastai/fastai.
Jahanifar, M., Shepard, A., Zamanitajeddin, N., Bashir, R.,
Bilal, M., Khurram, S. A., Minhas, F., and Rajpoot,
N. (2021). Stain-robust mitotic figure detection for
the mitosis domain generalization challenge. In In-
ternational Conference on Medical Image Comput-
ing and Computer-Assisted Intervention, pages 48–
52. Springer.
Johnson, J., Alahi, A., and Fei-Fei, L. (2016). Perceptual
losses for real-time style transfer and super-resolution.
In European conference on computer vision, pages
694–711. Springer.
Lin, T., Goyal, P., Girshick, R., He, K., and Dollar,
P. (2017a). Focal loss for dense object detection
2017 ieee international conference on computer vision
(iccv).
Lin, T.-Y., Goyal, P., Girshick, R., He, K., and Doll
´
ar, P.
(2017b). Focal loss for dense object detection. In 2017
IEEE International Conference on Computer Vision
(ICCV), pages 2999–3007.
Long, X., Cheng, Y., Mu, X., Liu, L., and Liu, J.
(2021). Domain adaptive cascade r-cnn for mito-
sis domain generalization (midog) challenge. In In-
ternational Conference on Medical Image Comput-
ing and Computer-Assisted Intervention, pages 73–
76. Springer.
Nateghi, R. and Pourakpour, F. (2021). Two-step domain
adaptation for mitotic cell detection in histopathology
images. In International Conference on Medical Im-
age Computing and Computer-Assisted Intervention,
pages 32–39. Springer.
Paszke, A., Gross, S., Massa, F., Lerer, A., Bradbury, J.,
Chanan, G., Killeen, T., Lin, Z., Gimelshein, N.,
Antiga, L., et al. (2019). Pytorch: An imperative style,
high-performance deep learning library. Advances in
neural information processing systems, 32.
Peter Byfield, a. T. G. and Gamper, J. (Accessed
in 03 Aug 2022). Staintools homepage. In
https://github.com/Peter554/StainTools.
Reinhard, E., Adhikhmin, M., Gooch, B., and Shirley, P.
(2001). Color transfer between images. IEEE Com-
puter graphics and applications, 21(5):34–41.
Vahadane, A., Peng, T., Albarqouni, S., Baust, M., Steiger,
K., Schlitter, A. M., Sethi, A., Esposito, I., and Navab,
N. (2015). Structure-preserved color normalization
for histological images. In 2015 IEEE 12th Inter-
national Symposium on Biomedical Imaging (ISBI),
pages 1012–1015. IEEE.
Vahadane, A., Peng, T., Sethi, A., Albarqouni, S., Wang,
L., Baust, M., Steiger, K., Schlitter, A. M., Esposito,
I., and Navab, N. (2016). Structure-preserving color
normalization and sparse stain separation for histolog-
ical images. IEEE transactions on medical imaging,
35(8):1962–1971.
Wilm, F., Marzahl, C., Breininger, K., and Aubreville, M.
(2021). Domain adversarial retinanet as a reference
algorithm for the mitosis domain generalization chal-
lenge. In International Conference on Medical Im-
age Computing and Computer-Assisted Intervention,
pages 5–13. Springer.
Zhu, J.-Y., Park, T., Isola, P., and Efros, A. A. (2017).
Unpaired image-to-image translation using cycle-
consistent adversarial networks. In Proceedings of
the IEEE international conference on computer vi-
sion, pages 2223–2232.
BIOIMAGING 2023 - 10th International Conference on Bioimaging
56