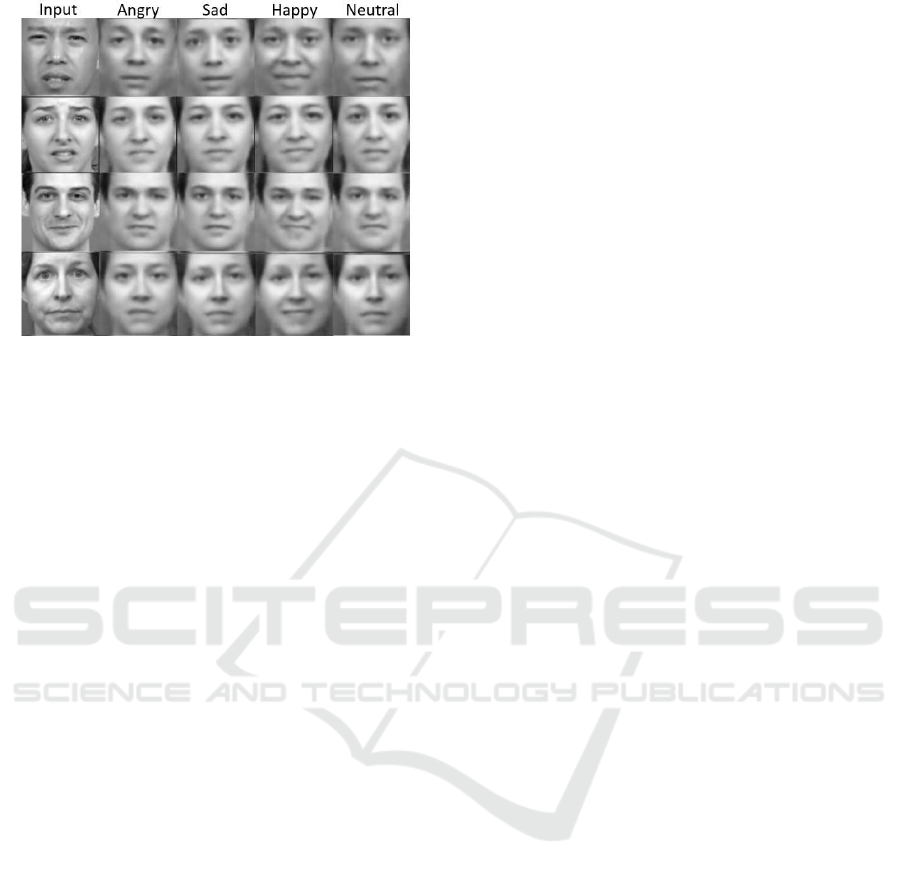
Figure 7: Result of replacing labels.
ferences were not as clear as for the “happy”.
The reason for this is assumed to be that there is
a wide range of emotions in “angry” and “sad”. For
example, there are many types of facial expressions
that are expressed depending on the type of angery,
such as “cross,” “annoyed,” and “irritated,” and it is
possible that sad and neutral faces were included in
the angery-labeled images, which may have nega-
tively affected learning. On the contrary, “happy” is
the only positive emotion, and although there are dif-
ferent types such as “smile,” “laugh,” and “grin,” it
has the common feature of raising the corners of the
mouth, and we assume that it was learned successfully
with low learning difficulty.
5.3 Voice Encoder Training and
Generation Experiment
First, from Figure 6, it can be confirmed that the voice
encoder was successfully trained with the ImageEn-
coder features of VAE. Next, the trained voice en-
coder is integrated into VAE as shown in Figure 3,
and the results of face image generation from voice
are confirmed. Figure 7 shows that the general char-
acteristics of the face, such as gender, were captured.
Although there are some differences in details such
as the size of the eyes and the depth of the moat, the
accuracy is sufficient for one of the purposes of this
study, which is “to understand the face of an unknown
person from voice alone”. As in 5.2, significant re-
sults were obtained for the facial expression “happi-
ness”, but for the other emotions, the accuracy was
only as good as the identification of positive and neg-
ative emotions. We assume that this is also due to the
fact that a wide range of emotions are included, as
was the case in the VAE training.
6 CONCLUSION
In this paper, we proposed a method for generating
face images that reflect the attributes and emotions of
the speaker based on voice input for the purpose of
conducting a simulated video call using only voice.
As a result, the proposed method was not able to learn
facial expressions well when negative emotions such
as “angry” and “sad” had similar facial expressions,
but it was able to reflect emotional information well
for emotions with monotonous facial expression fea-
tures such as “happy”. In addition, we were able to
generate a face image from the input speech that re-
flected the attributes of the speaker’s face image.
7 FUTURE WORK
This paper does not quantitatively evaluate the gen-
eration results using specific indicators. Therefore,
it is necessary to quantitatively evaluate the gener-
ation results of this model using specific numerical
values in the future. In addition, since the purpose
of this research is to understand the face and facial
expression of an unknown speaker during telephone
communication, it is more important to generate the
most plausible face image for the input voice than to
generate the exact face of the speaker. Therefore, in
addition to quantitative evaluation, qualitative evalu-
ation is also an important evaluation item and should
be conducted. As a future challenge, we would like
to expand the training data to a larger dataset to im-
prove the robustness of the model to all types of input
speech, since it cannot be said that we have acquired
sufficient speaker diversity with the dataset used in
this study.
ACKNOWLEDGEMENTS
This work was supported by JSPS KAKENHI Grant
Numbers JP21H03496, JP22K12157.
REFERENCES
Cao, H., Cooper, D. G., Keutmann, M. K., Gur, R. C.,
Nenkova, A., and Verma, R. (2014). Crema-d: Crowd-
sourced emotional multimodal actors dataset. IEEE
transactions on affective computing, 5(4):377–390.
Chowdhury, A., Ross, A., and David, P. (2021). Deeptalk:
Vocal style encoding for speaker recognition and
speech synthesis. In ICASSP 2021-2021 IEEE Inter-
national Conference on Acoustics, Speech and Signal
Processing (ICASSP), pages 6189–6193. IEEE.
ICAART 2023 - 15th International Conference on Agents and Artificial Intelligence
104