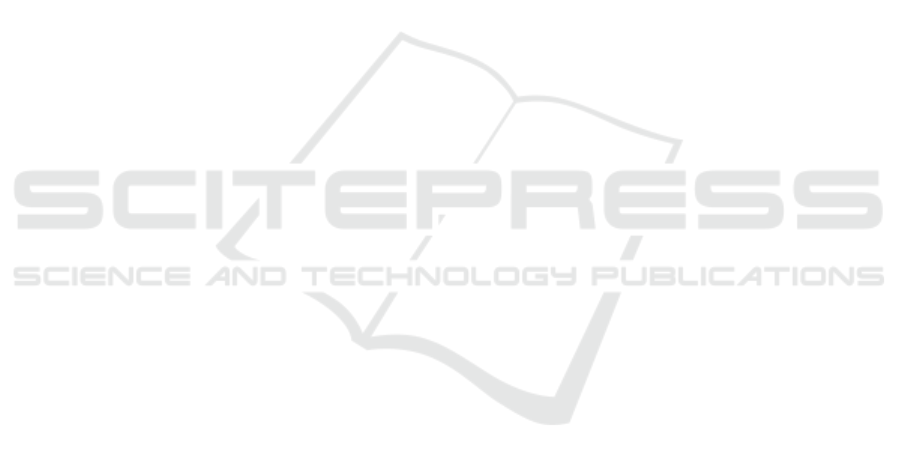
Kautz, J. (2018). Domain stylization: A strong, sim-
ple baseline for synthetic to real image domain adap-
tation. arXiv:1807.09384.
Goodfellow, I. J., Pouget-Abadie, J., Mirza, M., Xu, B.,
Warde-Farley, D., Ozair, S., Courville, A., and Ben-
gio, Y. (2014). Generative adversarial nets. In
Proceedings of the 27th International Conference on
Neural Information Processing Systems - Volume 2,
NIPS’14, pages 2672–2680, Cambridge, MA, USA.
MIT Press.
Grigoryev, T., Voynov, A., and Babenko, A. (2022).
When, Why, and Which Pretrained GANs Are Use-
ful? arXiv:2202.08937.
Gulrajani, I., Ahmed, F., Arjovsky, M., Dumoulin, V., and
Courville, A. (2017). Improved Training of Wasser-
stein GANs. arXiv:1704.00028.
He, K., Zhang, X., Ren, S., and Sun, J. (2016). Deep Resid-
ual Learning for Image Recognition. In Proceedings
of the IEEE Conference on Computer Vision and Pat-
tern Recognition, pages 770–778.
Hoffman, J., Tzeng, E., Park, T., Zhu, J.-Y., Isola, P.,
Saenko, K., Efros, A., and Darrell, T. (2018). Cy-
CADA: Cycle-Consistent Adversarial Domain Adap-
tation. In International Conference on Machine
Learning, pages 1989–1998. PMLR.
Hoffman, J., Wang, D., Yu, F., and Darrell, T. (2016). FCNs
in the Wild: Pixel-level Adversarial and Constraint-
based Adaptation. arXiv:1612.02649.
Isola, P., Zhu, J.-Y., Zhou, T., and Efros, A. A. (2017).
Image-to-Image Translation with Conditional Adver-
sarial Networks. In 2017 IEEE Conference on Com-
puter Vision and Pattern Recognition, pages 5967–
5976.
Jaccard, P. (1912). The Distribution of the Flora in the
Alpine Zone. New Phytologist, 11(2):37–50.
Jiang, P., Wu, A., Han, Y., Shao, Y., Qi, M., and Li, B.
(2020). Bidirectional Adversarial Training for Semi-
Supervised Domain Adaptation. In Twenty-Ninth In-
ternational Joint Conference on Artificial Intelligence,
volume 1, pages 934–940.
J
¨
ulich Supercomputing Centre (2019). JUWELS: Modu-
lar Tier-0/1 Supercomputer at the J
¨
ulich Supercom-
puting Centre. Journal of large-scale research facili-
ties, 5(A135).
Kang, G., Wei, Y., Yang, Y., Zhuang, Y., and Hauptmann,
A. (2020). Pixel-Level Cycle Association: A New
Perspective for Domain Adaptive Semantic Segmen-
tation. In Advances in Neural Information Processing
Systems, volume 33, pages 3569–3580. Curran Asso-
ciates, Inc.
Kim, M. and Byun, H. (2020). Learning Texture Invari-
ant Representation for Domain Adaptation of Seman-
tic Segmentation. In Proceedings of the IEEE/CVF
conference on computer vision and pattern recogni-
tion, pages 12975–12984.
Kim, T. and Kim, C. (2020). Attract, Perturb, and Ex-
plore: Learning a Feature Alignment Network for
Semi-supervised Domain Adaptation. In European
conference on computer vision – ECCV 2020, pages
591–607, Cham. Springer International Publishing.
Kingma, D. P. and Ba, J. (2014). Adam: A method for
stochastic optimization. arXiv:1412.6980.
Liu, Z., Lin, Y., Cao, Y., Hu, H., Wei, Y., Zhang, Z., Lin,
S., and Guo, B. (2021). Swin transformer: Hierarchi-
cal vision transformer using shifted windows. In Pro-
ceedings of the IEEE/CVF International Conference
on Computer Vision, pages 10012–10022.
Mabu, S., Miyake, M., Kuremoto, T., and Kido, S. (2021).
Semi-supervised CycleGAN for domain transforma-
tion of chest CT images and its application to opacity
classification of diffuse lung diseases. International
Journal of Computer Assisted Radiology and Surgery,
16(11):1925–1935.
Mao, X., Li, Q., Xie, H., Lau, R. Y. K., Wang, Z., and
Paul Smolley, S. (2017). Least Squares Generative
Adversarial Networks. In Proceedings of the IEEE
International Conference on Computer Vision, pages
2794–2802.
Mei, K., Zhu, C., Zou, J., and Zhang, S. (2020). In-
stance Adaptive Self-Training for Unsupervised Do-
main Adaptation. arXiv:2008.12197.
Minaee, S., Boykov, Y. Y., Porikli, F., Plaza, A. J., Kehtar-
navaz, N., and Terzopoulos, D. (2021). Image Seg-
mentation Using Deep Learning: A Survey. IEEE
Transactions on Pattern Analysis and Machine Intel-
ligence.
Pang, Y., Lin, J., Qin, T., and Chen, Z. (2021). Image-to-
Image Translation: Methods and Applications. IEEE
Transactions on Multimedia.
Ros, G., Sellart, L., Materzynska, J., V
´
azquez, D., and
L
´
opez, A. M. (2016). The SYNTHIA Dataset: A
Large Collection of Synthetic Images for Semantic
Segmentation of Urban Scenes. In The IEEE Con-
ference on Computer Vision and Pattern Recognition,
pages 3234–3243.
Rottmann, M. and Reese, M. (2022). Automated Detection
of Label Errors in Semantic Segmentation Datasets
via Deep Learning and Uncertainty Quantification.
arXiv:2207.06104.
Saito, K., Kim, D., Sclaroff, S., Darrell, T., and Saenko,
K. (2019). Semi-supervised domain adaptation via
minimax entropy. In Proceedings of the IEEE/CVF
International Conference on Computer Vision, pages
8050–8058.
Sangkloy, P., Burnell, N., Ham, C., and Hays, J. (2016).
The sketchy database: learning to retrieve badly
drawn bunnies. ACM Transactions on Graphics,
35(4):119:1–119:12.
Shukla, S., Van Gool, L., and Timofte, R. (2019). Extremely
Weak Supervised Image-to-Image Translation for Se-
mantic Segmentation. In 2019 IEEE/CVF Interna-
tional Conference on Computer Vision Workshop (IC-
CVW), pages 3368–3377. ISSN: 2473-9944.
Tao, A., Sapra, K., and Catanzaro, B. (2020). Hierarchi-
cal multi-scale attention for semantic segmentation.
arXiv:2005.10821.
Toldo, M., Maracani, A., Michieli, U., and Zanuttigh, P.
(2020). Unsupervised Domain Adaptation in Seman-
tic Segmentation: A Review. Technologies, 8(2):35.
Semi-Supervised Domain Adaptation with CycleGAN Guided by Downstream Task Awareness
89