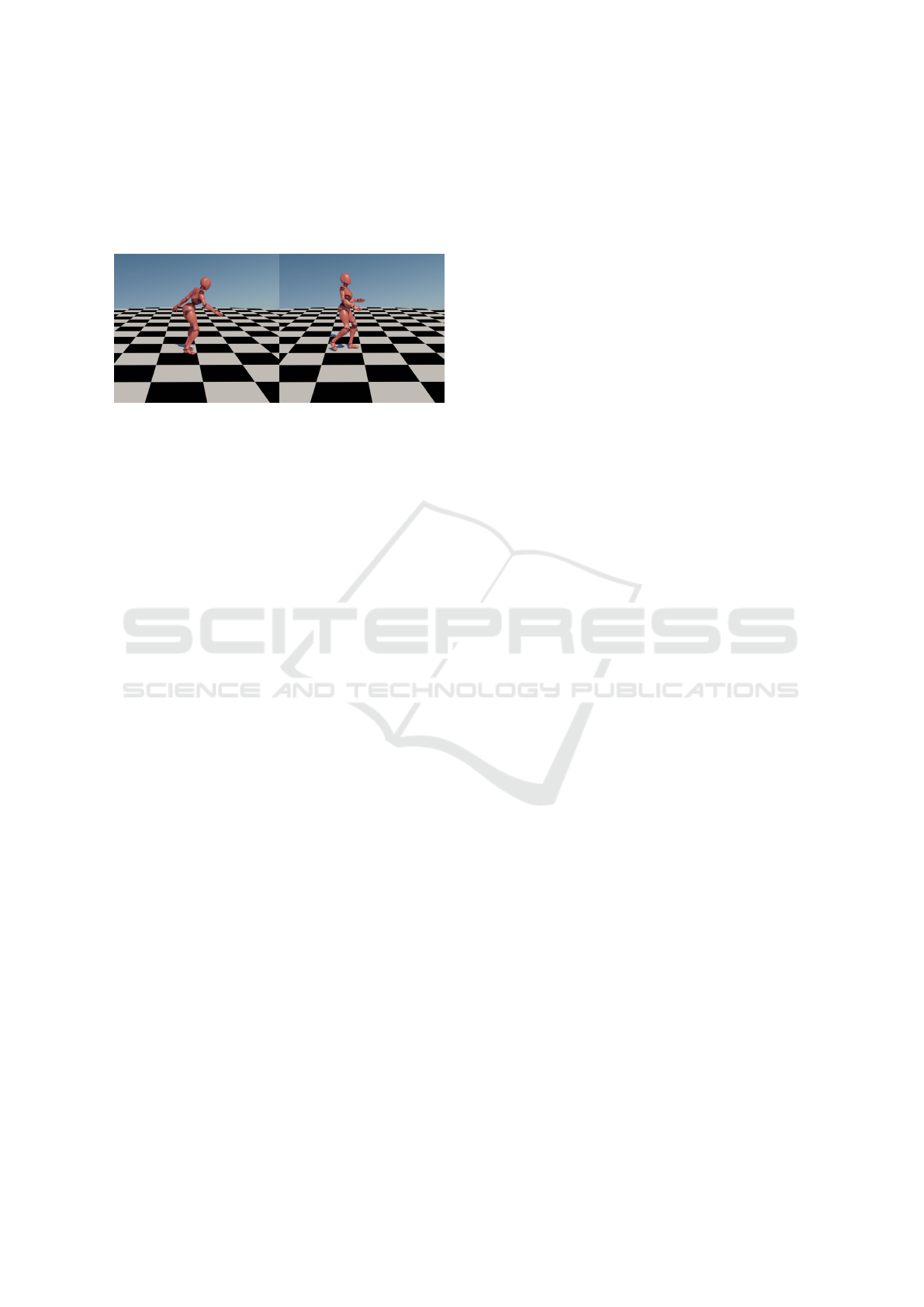
jection. The stochastic property makes the gener-
ation difficult to achieve higher controllingness and
stability. The stochastic system such as DDPM re-
quires further mathematical theories on stochasticity
to achieve higher stability and better controllingness.
Figure 9: Punching is a challenging case.
Another potential direction in future is the control
of limb ends, e.g. feet and hands. Figure 9 shows
a challenging case. In human motions, the ends of
limbs usually have tiny but complicated movements
that DDPM is hard to model. Although we estimate
foot patterns and trajectory of movements, the control
of limb ends still has the potential to be improved by
several ways such as physics-based guidance.
REFERENCES
Aberman, K., Weng, Y., Lischinski, D., Cohen-Or, D., and
Chen, B. (2020). Unpaired motion style transfer from
video to animation. ACM Transactions on Graphics
(TOG), 39(4):64–1.
Ahn, H., Ha, T., Choi, Y., Yoo, H., and Oh, S. (2018).
Text2action: Generative adversarial synthesis from
language to action. In 2018 IEEE International Con-
ference on Robotics and Automation (ICRA), pages
5915–5920. IEEE.
Bond-Taylor, S., Leach, A., Long, Y., and Willcocks, C. G.
(2021). Deep generative modelling: A compara-
tive review of vaes, gans, normalizing flows, energy-
based and autoregressive models. arXiv preprint
arXiv:2103.04922.
Dhariwal, P. and Nichol, A. (2021). Diffusion models beat
gans on image synthesis. Advances in Neural Infor-
mation Processing Systems, 34:8780–8794.
Dong, Y., Aristidou, A., Shamir, A., Mahler, M., and Jain,
E. (2020). Adult2child: Motion style transfer using
cyclegans. In Motion, interaction and games, pages
1–11.
Findlay, E. J., Zhang, H., Chang, Z., and Shum, H. P.
(2022). Denoising diffusion probabilistic mod-
els for styled walking synthesis. arXiv preprint
arXiv:2209.14828.
Henter, G. E., Alexanderson, S., and Beskow, J. (2020).
Moglow: Probabilistic and controllable motion syn-
thesis using normalising flows. ACM Transactions on
Graphics (TOG), 39(6):1–14.
Heusel, M., Ramsauer, H., Unterthiner, T., Nessler, B., and
Hochreiter, S. (2017). Gans trained by a two time-
scale update rule converge to a local nash equilibrium.
Advances in neural information processing systems,
30.
Ho, J., Jain, A., and Abbeel, P. (2020). Denoising diffusion
probabilistic models. Advances in Neural Information
Processing Systems, 33:6840–6851.
Holden, D., Komura, T., and Saito, J. (2017). Phase-
functioned neural networks for character control.
ACM Transactions on Graphics (TOG), 36(4):1–13.
Kawar, B., Elad, M., Ermon, S., and Song, J. (2022). De-
noising diffusion restoration models. arXiv preprint
arXiv:2201.11793.
Ling, H. Y., Zinno, F., Cheng, G., and Van De Panne, M.
(2020). Character controllers using motion vaes. ACM
Transactions on Graphics (TOG), 39(4):40–1.
Martinez, J., Black, M. J., and Romero, J. (2017). On
human motion prediction using recurrent neural net-
works. In Proceedings of the IEEE conference on
computer vision and pattern recognition, pages 2891–
2900.
Mourot, L., Hoyet, L., Le Clerc, F., Schnitzler, F., and Hel-
lier, P. (2022). A survey on deep learning for skeleton-
based human animation. In Computer Graphics Fo-
rum, volume 41, pages 122–157. Wiley Online Li-
brary.
Nichol, A., Dhariwal, P., Ramesh, A., Shyam, P., Mishkin,
P., McGrew, B., Sutskever, I., and Chen, M. (2021).
Glide: Towards photorealistic image generation and
editing with text-guided diffusion models. arXiv
preprint arXiv:2112.10741.
Nichol, A. Q. and Dhariwal, P. (2021). Improved denois-
ing diffusion probabilistic models. In International
Conference on Machine Learning, pages 8162–8171.
PMLR.
Petrovich, M., Black, M. J., and Varol, G. (2021). Action-
conditioned 3d human motion synthesis with trans-
former vae. In Proceedings of the IEEE/CVF Interna-
tional Conference on Computer Vision, pages 10985–
10995.
Ramesh, A., Dhariwal, P., Nichol, A., Chu, C., and
Chen, M. (2022). Hierarchical text-conditional im-
age generation with clip latents. arXiv preprint
arXiv:2204.06125.
Saharia, C., Chan, W., Saxena, S., Li, L., Whang, J., Den-
ton, E., Ghasemipour, S. K. S., Ayan, B. K., Mahdavi,
S. S., Lopes, R. G., et al. (2022). Photorealistic text-
to-image diffusion models with deep language under-
standing. arXiv preprint arXiv:2205.11487.
Sohl-Dickstein, J., Weiss, E., Maheswaranathan, N., and
Ganguli, S. (2015). Deep unsupervised learning us-
ing nonequilibrium thermodynamics. In International
Conference on Machine Learning, pages 2256–2265.
PMLR.
Song, J., Meng, C., and Ermon, S. (2020). De-
noising diffusion implicit models. arXiv preprint
arXiv:2010.02502.
Vahdat, A., Kreis, K., and Kautz, J. (2021). Score-based
generative modeling in latent space. Advances in
Unifying Human Motion Synthesis and Style Transfer with Denoising Diffusion Probabilistic Models
73