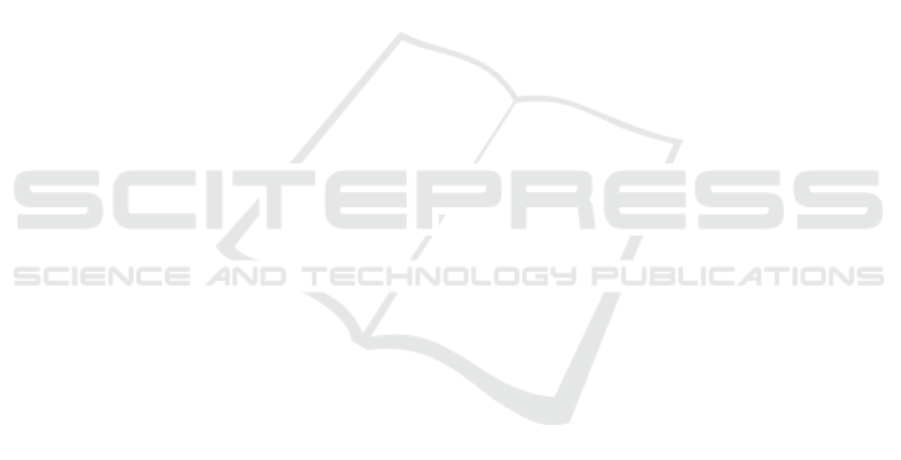
Lin, Z., Xu, P., Winata, G. I., Siddique, F. B., Liu, Z., Shin,
J., and Fung, P. (2020). Caire: An end-to-end empa-
thetic chatbot. In Proceedings of the AAAI Conference
on Artificial Intelligence, volume 34, pages 13622–
13623.
Liu, S., Zheng, C., Demasi, O., Sabour, S., Li, Y., Yu, Z.,
Jiang, Y., and Huang, M. (2021). Towards emotional
support dialog systems. ArXiv, abs/2106.01144.
Lu, X., Tian, Y., Zhao, Y., and Qin, B. (2021). Re-
trieve, discriminate and rewrite: A simple and ef-
fective framework for obtaining affective response in
retrieval-based chatbots. In Findings of the Associ-
ation for Computational Linguistics: EMNLP 2021,
pages 1956–1969.
Ma, Y., Nguyen, K. L., Xing, F. Z., and Cambria, E. (2020).
A survey on empathetic dialogue systems. Informa-
tion Fusion, 64:50–70.
Madotto, A., Lin, Z., Wu, C.-S., and Fung, P. (2019). Per-
sonalizing dialogue agents via meta-learning. In Pro-
ceedings of the 57th Annual Meeting of the Associa-
tion for Computational Linguistics, pages 5454–5459.
Mascarenhas, S., Guimar
˜
aes, M., Santos, P. A., Dias, J.,
Prada, R., and Paiva, A. (2021). Fatima toolkit–toward
an effective and accessible tool for the development
of intelligent virtual agents and social robots. arXiv
preprint arXiv:2103.03020.
Maslowski, I., Lagarde, D., and Clavel, C. (2017). In-the-
wild chatbot corpus: from opinion analysis to interac-
tion problem detection. In ICNLSSP 2017, pages 115–
120, Casablanca, Morocco. ISGA, Institut Sup
´
erieur
d’inG
´
enierie et des Affaires.
Mazar
´
e, P.-E., Humeau, S., Raison, M., and Bordes, A.
(2018). Training millions of personalized dialogue
agents. In Proceedings of the 2018 Conference on
Empirical Methods in Natural Language Processing,
pages 2775–2779, Brussels, Belgium. Association for
Computational Linguistics.
Ochs, M., Sabouret, N., and Corruble, V. (2008). Model-
ing the dynamics of non-player characters’ social re-
lations in video game. In Proceedings of the AAAI
Conference on Artificial Intelligence and Interactive
Digital Entertainment, volume 4, pages 90–95.
Pamungkas, E. W. (2019). Emotionally-aware chatbots: A
survey. arXiv preprint arXiv:1906.09774.
Papineni, K., Roukos, S., Ward, T., and Zhu, W.-J. (2002).
Bleu: a method for automatic evaluation of machine
translation. In Proceedings of the 40th Annual Meet-
ing of the Association for Computational Linguistics,
pages 311–318, Philadelphia, Pennsylvania, USA.
Association for Computational Linguistics.
Plutchik, R. (1984). Emotions : a general psychoevolution-
ary theory.
Radford, A., Wu, J., Child, R., Luan, D., Amodei, D., and
Sutskever, I. (2019). Language models are unsuper-
vised multitask learners.
Rashkin, H., Smith, E. M., Li, M., and Boureau, Y.-L.
(2018). Towards empathetic open-domain conversa-
tion models: A new benchmark and dataset. arXiv
preprint arXiv:1811.00207.
Santos Teixeira, M. and Dragoni, M. (2022). A review
of plan-based approaches for dialogue management.
Cognitive Computation, pages 1–20.
Shu, C., Zhang, Y., Dong, X., Shi, P., Yu, T., and Zhang,
R. (2021). Logic-consistency text generation from
semantic parses. In Findings of the Association
for Computational Linguistics: ACL-IJCNLP 2021,
pages 4414–4426, Online. Association for Computa-
tional Linguistics.
Skerry, A. E., Saxe, R., Skerry, A. E., and Saxe, R.
(2015). Neural representations of emotion are orga-
nized around abstract event features. Curr. Biol.
Soni, M., Cowan, B., and Wade, V. (2021). Enhancing
self-disclosure in neural dialog models by candidate
re-ranking. arXiv preprint arXiv:2109.05090.
Sun, K., Moon, S., Crook, P., Roller, S., Silvert, B., Liu,
B., Wang, Z., Liu, H., Cho, E., and Cardie, C. (2020).
Adding chit-chat to enhance task-oriented dialogues.
Wang, Y.-H., Hsu, J.-H., Wu, C.-H., and Yang, T.-H.
(2021). Transformer-based empathetic response gen-
eration using dialogue situation and advanced-level
definition of empathy. In 2021 12th International
Symposium on Chinese Spoken Language Processing
(ISCSLP), pages 1–5.
Wardhana, A. K., Ferdiana, R., and Hidayah, I. (2021).
Empathetic chatbot enhancement and development: A
literature review. In 2021 International Conference
on Artificial Intelligence and Mechatronics Systems
(AIMS), pages 1–6.
Welivita, A., Xie, Y., and Pu, P. (2021). A large-scale
dataset for empathetic response generation. In Pro-
ceedings of the 2021 Conference on Empirical Meth-
ods in Natural Language Processing, pages 1251–
1264.
Wolf, T., Sanh, V., Chaumond, J., and Delangue, C. (2019).
Transfertransfo: A transfer learning approach for
neural network based conversational agents. arXiv
preprint arXiv:1901.08149.
Wu, B., Li, M., Wang, Z., Chen, Y., Wong, D., Feng, Q.,
Huang, J., and Wang, B. (2019). Guiding variational
response generator to exploit persona.
Yacoubi, A. and Sabouret, N. (2018). Teatime: A formal
model of action tendencies in conversational agents.
In ICAART (2), pages 143–153.
Zhang, S., Dinan, E., Urbanek, J., Szlam, A., Kiela, D.,
and Weston, J. (2018). Personalizing dialogue agents:
I have a dog, do you have pets too? In Proceed-
ings of the 56th Annual Meeting of the Association for
Computational Linguistics (Volume 1: Long Papers),
pages 2204–2213, Melbourne, Australia. Association
for Computational Linguistics.
Zheng, Y., Zhang, R., Huang, M., and Mao, X. (2020).
A pre-training based personalized dialogue generation
model with persona-sparse data. In Proceedings of
the AAAI Conferencezh on Artificial Intelligence, vol-
ume 34, pages 9693–9700.
Zhong, P., Zhang, C., Wang, H., Liu, Y., and Miao, C.
(2020). Towards persona-based empathetic conversa-
tional models. arXiv preprint arXiv:2004.12316.
ICAART 2023 - 15th International Conference on Agents and Artificial Intelligence
192