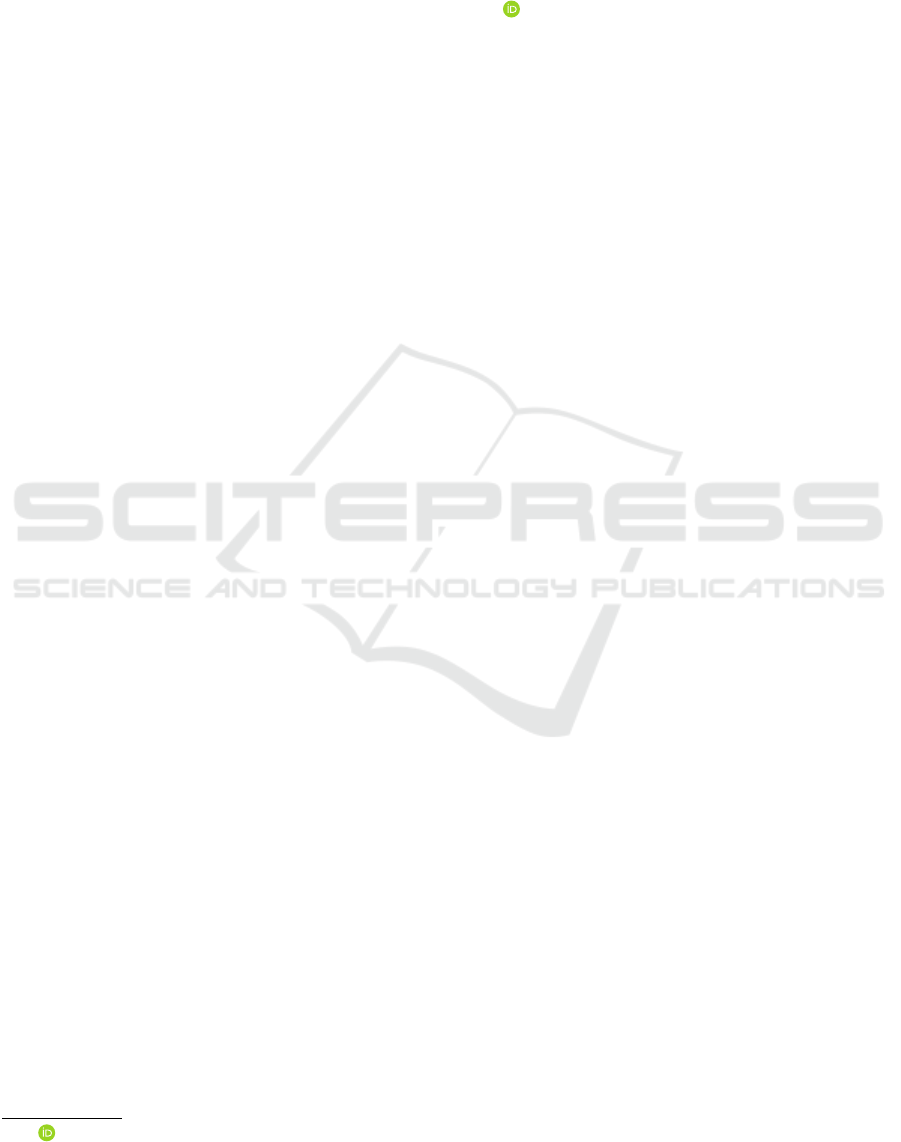
On the Prediction of a Nonstationary Exponential Distribution Based on
Bayes Decision Theory
Daiki Koizumi
a
Otaru University of Commerce, 3–5–21, Midori, Otaru-city, Hokkaido, 045–8501, Japan
Keywords:
Probability Model, Bayes Decision Theory, Nonstationary Exponential Distribution, Hierarchical Bayesian
Model.
Abstract:
A prediction problem with a nonstationary exponential distribution based on the Bayes decision theory was
considered in this paper. The proposed predictive algorithm is based on both posterior and predictive dis-
tributions in a Bayesian context. The predictive estimator satisfies the Bayes optimality, which guarantees
a minimum average error rate with a nonstationary probability model, a squared loss function, and a prior
distribution of parameter. Finally, the predictive performance of the proposed algorithm was evaluated via
comparison with the stationary exponential distribution using real meteorological data.
1 INTRODUCTION
The exponential distribution (Johnson et al., 1994;
Bernardo and Smith, 2000) is a continuous prob-
ability distribution that has applications in various
fields such as queuing theory (Kleinrock, 1975; Allen,
1990; Ross, 1997), reliability engineering (Gnedenko
et al., 1969; Trivedi, 1982; Ross, 1997), and Bayesian
statistics (Bernardo and Smith, 2000; Press, 2003).
The stationary exponential distribution can be de-
fined by both a nonnegative continuous random vari-
able and a nonnegative parameter. The stationary ex-
ponential distribution has the so-called memoryless
property, which leads to an independent distribution
of service time in queuing theory (Allen, 1990, p. 123,
3.2.2), and a constant failure rate of lifetime distri-
bution in reliability theory (Trivedi, 1982, pp. 122–
123). Furthermore, the maximum likelihood estima-
tor of the stationary exponential distribution can be
obtained via simple arithmetic calculations (Trivedi,
1982, p. 482, Example 10.8); this implies that it is
tractable for parameter estimation with real data.
Nevertheless, in the field of Bayesian statistics,
parameter estimation or prediction problems with the
Bayesian approach often become intractable prob-
lems. This is because these problems require inte-
gral calculations in the denominator of the Bayes the-
orem depending on a known prior distribution of pa-
rameter. However, if the specific distribution of pa-
rameter is assumed to be the prior, complex integral
calculations can be avoided. In Bayesian statistics,
a
https://orcid.org/0000-0002-5302-5346
this specific class of prior is called a conjugate fam-
ily (Berger, 1985, pp. 130–132) (Bernardo and Smith,
2000, pp. 265–267). The gamma distribution is the
natural conjugate prior of the stationary exponential
distribution (Bernardo and Smith, 2000, p. 438).
The aforementioned results are limited within the
stationary probability distributions. If nonstationary
probability distributions are assumed, the Bayesian
estimation problems become more difficult and more
intractable. In such cases, there is no guarantee of the
existence of a natural conjugate prior. In this regard,
an interesting class of nonstationary probability mod-
els has been proposed, referred to as the Simple Power
Steady Model (SPSM) (Smith, 1979). The SPSM is
a time-series model with a specific class of nonsta-
tionary parameters. Under SPSM, they have shown
certain illustrative probability distributions called lin-
ear expanding families in which natural conjugate pri-
ors exist (Smith, 1979). With regard to probability
distributions in linear expanding families, the author
proposed the same nonstationary parameter classes
as in SPSM, approximated maximum likelihood es-
timation method of hyperparameters, and similar up-
dating rules for the posterior distribution of parame-
ters (Koizumi, 2020; Koizumi, 2021). Furthermore,
a Bayesian problem in the Bayes decision theory was
considered. Using this approach, the predictive esti-
mator satisfies Bayes optimality, which guarantees a
minimum average error rate for predictions. This ap-
proach has been applied to both a nonstationary Pois-
son distribution (Koizumi, 2020) and nonstationary
Bernoulli distribution (Koizumi, 2021). The former
concerns not only the Bayes optimal point prediction
Koizumi, D.
On the Prediction of a Nonstationary Exponential Distribution Based on Bayes Decision Theory.
DOI: 10.5220/0011632500003393
In Proceedings of the 15th International Conference on Agents and Artificial Intelligence (ICAART 2023) - Volume 3, pages 193-201
ISBN: 978-989-758-623-1; ISSN: 2184-433X
Copyright
c
2023 by SCITEPRESS – Science and Technology Publications, Lda. Under CC license (CC BY-NC-ND 4.0)
193