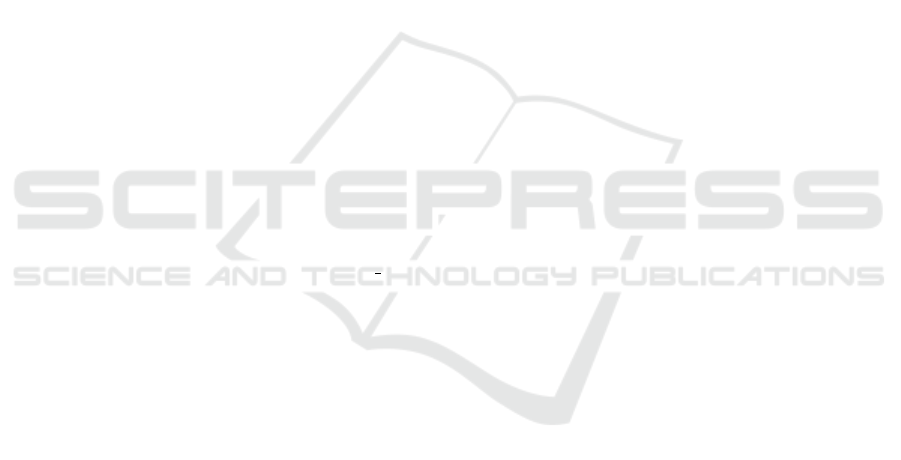
(2021). Effects of cognitive workload on heart and
locomotor rhythms coupling. Neuroscience Letters,
762:136140.
Feehan, L. M., Geldman, J., Sayre, E. C., Park, C., Ez-
zat, A. M., Yoo, J. Y., Hamilton, C. B., and Li,
L. C. (2018). Accuracy of Fitbit Devices: System-
atic Review and Narrative Syntheses of Quantitative
Data. JMIR mHealth and uHealth, 6(8):e10527. Com-
pany: JMIR mHealth and uHealth Distributor: JMIR
mHealth and uHealth Institution: JMIR mHealth and
uHealth Label: JMIR mHealth and uHealth Publisher:
JMIR Publications Inc., Toronto, Canada.
Hausdorff, J. M., Forman, D. E., Pilgrim, D. M., Rigney,
D. R., and Wei, J. Y. (1992). A new technique
for simultaneous monitoring of electrocardiogram and
walking cadence. The American Journal of Cardiol-
ogy, 70(11):1064–1071.
Jett
´
e, M., Sidney, K., and Bl
¨
umchen, G. (1990). Metabolic
equivalents (METS) in exercise testing, exercise pre-
scription, and evaluation of functional capacity. Clin-
ical Cardiology, 13(8):555–565.
Karami, Z., Hines, A., and Jahromi, H. Z. (2021). Leverag-
ing IoT Lifelog Data to Analyse Performance of Phys-
ical Activities. In 2021 32nd Irish Signals and Systems
Conference (ISSC), pages 1–6. ISSN: 2688-1454.
Kirby, R. L., Nugent, S. T., Marlow, R. W., Macli$od, D. A.,
Alan, Marble, E., Lee, R., Nugent, S. T., Mar, R. W.,
and Macleod, D. A. (1989). Coupling of cardiac and
locomotor rhythms. J. Appl. Physiol, pages 323–329.
Murphy, M. N., Mizuno, M., Mitchell, J. H., and
Smith, S. A. (2011). Cardiovascular regulation
by skeletal muscle reflexes in health and disease.
American Journal of Physiology-Heart and Circu-
latory Physiology, 301(4):H1191–H1204. eprint:
https://doi.org/10.1152/ajpheart.00208.2011.
Niizeki, K. and Miyamoto, Y. (1999). Phase-dependent
heartbeat modulation by muscle contractions dur-
ing dynamic handgrip in humans. American Jour-
nal of Physiology-Heart and Circulatory Physiology,
276(4):H1331–H1338. Publisher: American Physio-
logical Society.
Niizeki, K. and Saitoh, T. (2014). Cardiolocomotor phase
synchronization during rhythmic exercise. The Jour-
nal of Physical Fitness and Sports Medicine, 3(1):11–
20.
Nomura, K., Takei, Y., and Yanagida, Y. (2003). Compar-
ison of cardio-locomotor synchronization during run-
ning and cycling. European Journal of Applied Phys-
iology, 89(3):221–229.
Novak, V., Hu, K., Vyas, M., and Lipsitz, L. A. (2007).
Cardiolocomotor Coupling in Young and Elderly Peo-
ple. The Journals of Gerontology Series A: Biological
Sciences and Medical Sciences, 62(1):86–92.
Sj
¨
oberg, V., Westergren, J., Monnier, A., Martire, R. L.,
Hagstr
¨
omer, M.,
¨
Ang, B. O., and Vixner, L. (2021).
Wrist-Worn Activity Trackers in Laboratory and Free-
Living Settings for Patients With Chronic Pain: Cri-
terion Validity Study. JMIR mHealth and uHealth,
9(1):e24806. Company: JMIR mHealth and uHealth
Distributor: JMIR mHealth and uHealth Institution:
JMIR mHealth and uHealth Label: JMIR mHealth and
uHealth Publisher: JMIR Publications Inc., Toronto,
Canada.
Takeuchi, S., Nishida, Y., and Mizushima, T. (2014). Ef-
fects of Synchronization between Cardiac and Loco-
motor Rhythms on Oxy- gen Pulse during Walking.
page 7.
Thambawita, V., Hicks, S. A., Borgli, H., Stensland, H. K.,
Jha, D., Svensen, M. K., Pettersen, S.-A., Johansen,
D., Johansen, H. D., Pettersen, S. D., Nordvang, S.,
Pedersen, S., Gjerdrum, A., Grønli, T.-M., Fredriksen,
P. M., Eg, R., Hansen, K., Fagernes, S., Claudi, C.,
Biørn-Hansen, A., Nguyen, D. T. D., Kupka, T., Ham-
mer, H. L., Jain, R., Riegler, M. A., and Halvorsen, P.
(2020). PMData: a sports logging dataset. In Proceed-
ings of the 11th ACM Multimedia Systems Conference,
pages 231–236, Istanbul Turkey. ACM.
Tudor-Locke, C., Aguiar, E. J., Han, H., Ducharme, S. W.,
Schuna, J. M., Barreira, T. V., Moore, C. C., Busa,
M. A., Lim, J., Sirard, J. R., Chipkin, S. R., and Stau-
denmayer, J. (2019). Walking cadence (steps/min)
and intensity in 21–40 year olds: CADENCE-adults.
The International Journal of Behavioral Nutrition and
Physical Activity, 16:8.
Tudor-Locke, C., Camhi, S. M., Leonardi, C., Johnson,
W. D., Katzmarzyk, P. T., Earnest, C. P., and Church,
T. S. (2011). Patterns of adult stepping cadence
in the 2005-2006 NHANES. Preventive Medicine,
53(3):178–181.
Tudor-Locke, C., Ducharme, S. W., Aguiar, E. J., Schuna,
J. M., Barreira, T. V., Moore, C. C., Chase,
C. J., Gould, Z. R., Amalbert-Birriel, M. A., Mora-
Gonzalez, J., Chipkin, S. R., and Staudenmayer, J.
(2020). Walking cadence (steps/min) and intensity in
41 to 60-year-old adults: the CADENCE-adults study.
The International Journal of Behavioral Nutrition and
Physical Activity, 17:137.
Tudor-Locke, C., Mora-Gonzalez, J., Ducharme, S. W.,
Aguiar, E. J., Schuna, J. M., Barreira, T. V., Moore,
C. C., Chase, C. J., Gould, Z. R., Amalbert-Birriel,
M. A., Chipkin, S. R., and Staudenmayer, J. (2021).
Walking cadence (steps/min) and intensity in 61-85-
year-old adults: the CADENCE-Adults study. The In-
ternational Journal of Behavioral Nutrition and Phys-
ical Activity, 18(1):129.
Spontaneous Cardiac-Locomotor Coupling in Healthy Individuals During Daily Activities
177