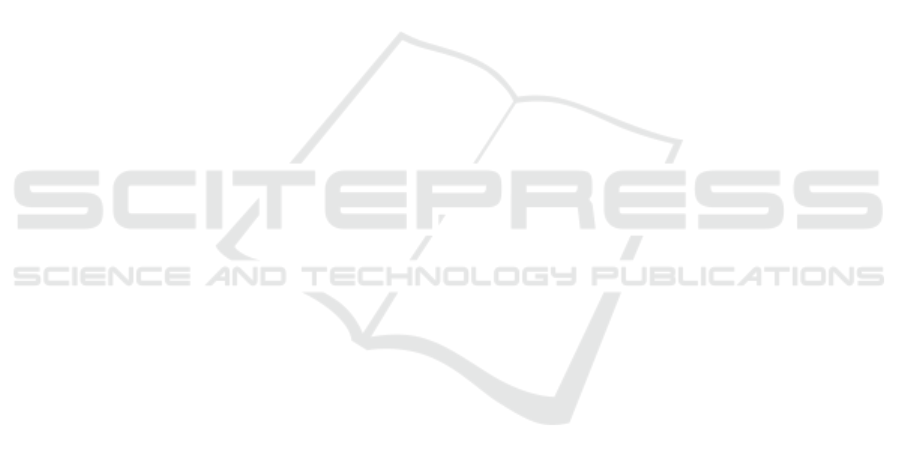
Dubois, F. and Yourassowsky, C. (2015). Off-axis interfer-
ometer.
Higgins, I., Matthey, L., Pal, A., Burgess, C., Glorot, X.,
Botvinick, M., Mohamed, S., and Lerchner, A. (2017).
beta-VAE: Learning Basic Visual Concepts with a
Constrained Variational Framework. In International
Conference on Learning Representations.
Jo, Y., Cho, H., Lee, S. Y., Choi, G., Kim, G., Min, H. S.,
and Park, Y. K. (2019). Quantitative Phase Imaging
and Artificial Intelligence: A Review. IEEE Journal
of Selected Topics in Quantum Electronics, 25(1):1–
14.
Jolliffe, I. T. and Cadima, J. (2016). Principal compo-
nent analysis: a review and recent developments.
Philosophical Transactions of the Royal Society A:
Mathematical, Physical and Engineering Sciences,
374(2065):20150202.
Kingma, D. P., Mohamed, S., Jimenez Rezende, D., and
Welling, M. (2014). Semi-supervised learning with
deep generative models. Advances in neural informa-
tion processing systems, 27.
Kingma, D. P. and Welling, M. (2013). Auto-encoding vari-
ational bayes. arXiv preprint arXiv:1312.6114.
Klenk, C., Heim, D., Ugele, M., and Hayden, O. (2019).
Impact of sample preparation on holographic imaging
of leukocytes. Optical Engineering, 59(10):102403.
Krizhevsky, A., Sutskever, I., and Hinton, G. E. (2012). Im-
agenet classification with deep convolutional neural
networks. In Advances in neural information process-
ing systems, pages 1097–1105.
Kutscher, T., Eder, K., Marzi, A., Barroso, A., Schneken-
burger, J., and Kemper, B. (2021). Cell Detection
and Segmentation in Quantitative Digital Holographic
Phase Contrast Images Utilizing a Mask Region-based
Convolutional Neural Network. In OSA Optical Sen-
sors and Sensing Congress 2021 (AIS, FTS, HISE,
SENSORS, ES), page JTu5A.23. Optica Publishing
Group.
Lam, V. K., Nguyen, T., Phan, T., Chung, B.-M., Nehmetal-
lah, G., and Raub, C. B. (2019). Machine learning
with optical phase signatures for phenotypic profiling
of cell lines. Cytometry Part A, 95(7):757–768.
Larsen, A. B. L., Sønderby, S. K., Larochelle, H., and
Winther, O. (2016). Autoencoding beyond pixels us-
ing a learned similarity metric. In Proceedings of The
33rd International Conference on Machine Learning,
volume 48 of Proceedings of Machine Learning Re-
search, pages 1558–1566. PMLR.
Maaløe, L., Sønderby, C. K., Sønderby, S. K., and Winther,
O. (2016). Auxiliary deep generative models. In In-
ternational Conference on Machine Learning, pages
1445–1453. PMLR.
Midtvedt, B., Helgadottir, S., Argun, A., Pineda, J.,
Midtvedt, D., and Volpe, G. (2021). Quantitative dig-
ital microscopy with deep learning. Applied Physics
Reviews, 8(1):011310.
Mishra, A., Krishna Reddy, S., Mittal, A., and Murthy,
H. A. (2018). A generative model for zero shot learn-
ing using conditional variational autoencoders. In
Proceedings of the IEEE Conference on Computer Vi-
sion and Pattern Recognition Workshops, pages 2188–
2196.
Nguyen, T. H., Sridharan, S., Macias, V., Kajdacsy-Balla,
A., Melamed, J., Do, M. N., and Popescu, G. (2017).
Automatic Gleason grading of prostate cancer us-
ing quantitative phase imaging and machine learning.
Journal of Biomedical Optics, 22(3):036015.
Nguyen, T. L., Pradeep, S., Judson-Torres, R. L., Reed, J.,
Teitell, M. A., and Zangle, T. A. (2022). Quantita-
tive phase imaging: Recent advances and expanding
potential in biomedicine. American Chemical Society
Nano, 16(8):11516–11544.
Paidi, S. K., Raj, P., Bordett, R., Zhang, C., Karandikar,
S. H., Pandey, R., and Barman, I. (2021). Raman and
quantitative phase imaging allow morpho-molecular
recognition of malignancy and stages of B-cell acute
lymphoblastic leukemia. Biosensors and Bioelectron-
ics, 190:113403.
Paine, S. W. and Fienup, J. R. (2018). Machine learning
for improved image-based wavefront sensing. Optics
Letters, 43(6):1235–1238.
Perez-Cruz, F. (2008). Kullback-Leibler divergence esti-
mation of continuous distributions. In 2008 IEEE In-
ternational Symposium on Information Theory, pages
1666–1670.
Sahoo, H. (2012). Fluorescent labeling techniques in
biomolecules: A flashback. Royal Society of Chem-
istry Advances, 2(18):7017–7029.
Schmidhuber, J. (2015). Deep learning in neural networks:
An overview. Neural Networks, 61:85–117.
Sender, R., Fuchs, S., and Milo, R. (2016). Revised esti-
mates for the number of human and bacteria cells in
the body. PLoS biology, 14(8):e1002533.
Suzuki, S. and Abe, K. (1985). Topological structural anal-
ysis of digitized binary images by border following.
Computer Vision, Graphics and Image Processing,
30(1):32–46.
Ugele, M. (2019). High-throughput hematology analy-
sis with digital holographic microscopy. PhD thesis,
Friedrich-Alexander-Universit
¨
at Erlangen-N
¨
urnberg
(FAU).
Ugele, M., Weniger, M., Stanzel, M., Bassler, M., Krause,
S. W., Friedrich, O., Hayden, O., and Richter, L.
(2018). Label-Free High-Throughput Leukemia De-
tection by Holographic Microscopy. Advanced Sci-
ence, 5(12).
Vuorte, J., Jansson, S.-E., and Repo, H. (2001). Evaluation
of red blood cell lysing solutions in the study of neu-
trophil oxidative burst by the DCFH assay. Cytometry,
43(4):290–296.
Yan, X., Yang, J., Sohn, K., and Lee, H. (2016). At-
tribute2image: Conditional image generation from vi-
sual attributes. In European Conference on Computer
Vision, pages 776–791. Springer.
Young, B., Woodford, P., and O’Dowd, G. (2013).
Wheater’s functional histology E-Book: a text and
colour atlas. Elsevier Health Sciences.
Explainable Feature Learning with Variational Autoencoders for Holographic Image Analysis
77