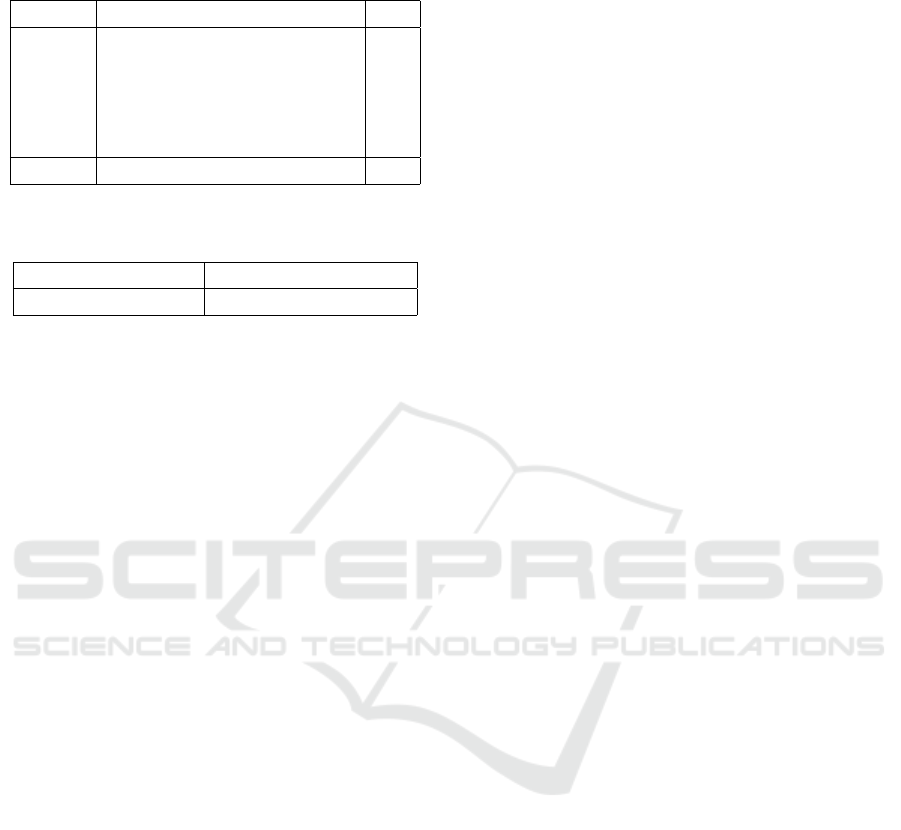
Table 5: Pixel-AUROC comparison on MVTEC AD :
MixedTeacher.
Category CutPaste PatchCore FastFlow Ours
carpet 98.3 98.9 99.1 99.0
tile 90.5 95.6 96.6 95.9
grid 97.5 98.7 99.2 97.5
wood 95.5 95 94.1 94.9
leather 99.5 99.3 99.6 99.4
Mean 96.2 97.5 97.7 97.3
Table 6: Image-AUROC comparison on BTAD:
MixedTeacher.
Category FastFlow Ours
1 (wood from btad) 96.0 97.0
5.4.3 Inference Time Results
In terms of inference speed, our MixedTeacher is 3x
slower than the reduced student since it used two
teacher networks and a more complex student archi-
tecture.
6 CONCLUSION
In this paper, we proposed two methods for effi-
cient unsupervised anomaly detection using the prin-
ciple of knowledge distillation applied to unsuper-
vised anomaly training. Both methods offer differ-
ent benefits. The reduced student proposes a high
speed texture anomaly detector with an AUROC per-
formance close to the state of the art, this method is
to be used in situations where inference time is the
most important priority (mobile device, low computa-
tional power, cost efficiency). The MixedTeacher pro-
pose the highest actual performance on anomaly de-
tection with a localisation close to the state of the art
on the MVTEC AD textures with still a fast inference.
This method is to be used in situations where perfor-
mance is the priority and the computational power is
big enough (monitoring server etc ...)
REFERENCES
Bergmann, P., Fauser, M., Sattlegger, D., and Steger, C.
MVTec AD — a comprehensive real-world dataset for
unsupervised anomaly detection. In 2019 IEEE/CVF
Conference on Computer Vision and Pattern Recogni-
tion (CVPR), pages 9584–9592. IEEE.
Deng, H. and Li, X. Anomaly detection via reverse distilla-
tion from one-class embedding.
Goodfellow, I. J., Pouget-Abadie, J., Mirza, M., Xu, B.,
Warde-Farley, D., Ozair, S., Courville, A., and Ben-
gio, Y. Generative adversarial networks.
He, K., Zhang, X., Ren, S., and Sun, J. Deep residual learn-
ing for image recognition.
Huang, J., Li, C., Lin, Y., Lian, S., and Innovation, A. Unsu-
pervised industrial anomaly detection via pattern gen-
erative and contrastive networks.
Iglesias, F. and Zseby, T. Analysis of network traffic fea-
tures for anomaly detection. 101(1):59–84.
K
¨
ahler, F., Schmedemann, O., and Sch
¨
uppstuhl, T.
Anomaly detection for industrial surface inspection:
application in maintenance of aircraft components.
107:246–251.
Lee, S., Lee, S., and Song, B. C. CFA: Coupled-
hypersphere-based feature adaptation for target-
oriented anomaly localization.
Li, C.-L., Sohn, K., Yoon, J., and Pfister, T. CutPaste: Self-
supervised learning for anomaly detection and local-
ization.
Liang, Y., Zhang, J., Zhao, S., Wu, R., Liu, Y., and Pan, S.
Omni-frequency channel-selection representations for
unsupervised anomaly detection.
Mei, S., Wang, Y., and Wen, G. Automatic fabric defect
detection with a multi-scale convolutional denoising
autoencoder network model. 18(4):1064.
Minhas, M. S. and Zelek, J. AnoNet: Weakly supervised
anomaly detection in textured surfaces.
Mishra, P., Verk, R., Fornasier, D., Piciarelli, C., and
Foresti, G. L. VT-ADL: A vision transformer net-
work for image anomaly detection and localization.
In 2021 IEEE 30th International Symposium on In-
dustrial Electronics (ISIE), pages 01–06.
Nguyen, Q. P., Lim, K. W., Divakaran, D. M., Low, K. H.,
and Chan, M. C. GEE: A gradient-based explainable
variational autoencoder for network anomaly detec-
tion.
Pourreza, M., Mohammadi, B., Khaki, M., Bouindour, S.,
Snoussi, H., and Sabokrou, M. G2d: Generate to de-
tect anomaly. In 2021 IEEE Winter Conference on Ap-
plications of Computer Vision (WACV), pages 2002–
2011. IEEE. event-place: Waikoloa, HI, USA.
Roth, K., Pemula, L., Zepeda, J., Sch
¨
olkopf, B., Brox,
T., and Gehler, P. Towards total recall in industrial
anomaly detection.
Rudolph, M., Wandt, B., and Rosenhahn, B. Same same
but DifferNet: Semi-supervised defect detection with
normalizing flows. In 2021 IEEE Winter Conference
on Applications of Computer Vision (WACV), pages
1906–1915. IEEE. event-place: Waikoloa, HI, USA.
Rudolph, M., Wehrbein, T., Rosenhahn, B., and Wandt, B.
Fully convolutional cross-scale-flows for image-based
defect detection.
Schlegl, T., Seeb
¨
ock, P., Waldstein, S. M., Langs, G.,
and Schmidt-Erfurth, U. f-AnoGAN: Fast unsuper-
vised anomaly detection with generative adversarial
networks. 54:30–44.
Tan, M. and Le, Q. V. EfficientNet: Rethinking model scal-
ing for convolutional neural networks.
Wang, G., Han, S., Ding, E., and Huang, D. Student-teacher
feature pyramid matching for anomaly detection.
MixedTeacher: Knowledge Distillation for Fast Inference Textural Anomaly Detection
493