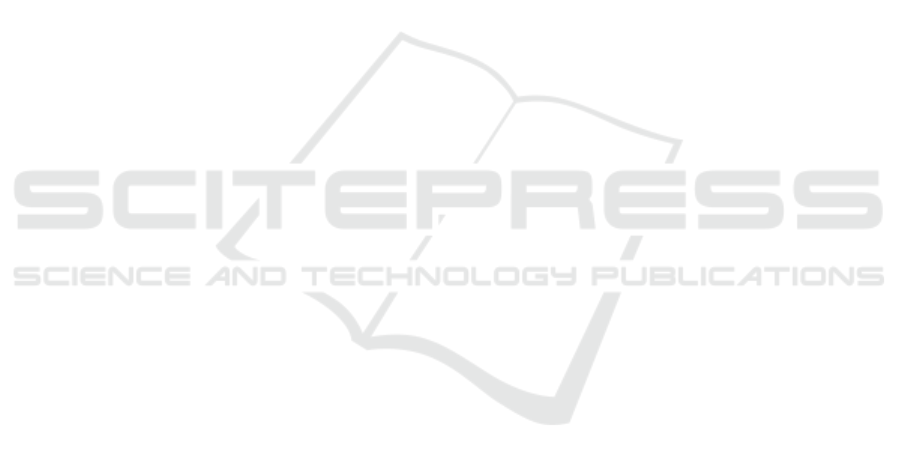
Devlin, J., Chang, M.-W., Lee, K., and Toutanova, K.
(2019). Bert: Pre-training of deep bidirectional trans-
formers for language understanding.
Dong, L., Yang, N., Wang, W., Wei, F., Liu, X., Wang, Y.,
Gao, J., Zhou, M., and Hon, H.-W. (2019). Unified
language model pre-training for natural language un-
derstanding and generation.
Elsas, J. and Carbonell, J. (2009). It pays to be picky: An
evaluation of thread retrieval in online forums. pages
714–715.
Faisal, M. S., Daud, A., Imran, F., and Rho, S. (2016). A
novel framework for social web forums’ thread rank-
ing based on semantics and post quality features. The
Journal of Supercomputing, 72:1–20.
Fleiss, J. (1971). Measuring nominal scale agree-
ment among many raters. Psychological bulletin,
76(5):378—382.
Hern
´
andez-Gonz
´
alez, J., Inza, I., and Lozano, J. (2018). A
note on the behavior of majority voting in multi-class
domains with biased annotators. IEEE Transactions
on Knowledge and Data Engineering, PP:1–1.
Jiao, X., Yin, Y., Shang, L., Jiang, X., Xiao, C., Li, L.,
Wang, F., and Liu, Q. (2020). Tinybert: Distilling bert
for natural language understanding.
Krippendorff, K. (2019). Content analysis : an introduc-
tion to its methodology. SAGE, Los Angeles, fourth
edition. edition.
Liu, Y., Ott, M., Goyal, N., Du, J., Joshi, M., Chen, D.,
Levy, O., Lewis, M., Zettlemoyer, L., and Stoyanov,
V. (2019). Roberta: A robustly optimized bert pre-
training approach.
Mikolov, T., Chen, K., Corrado, G., and Dean, J. (2013).
Efficient estimation of word representations in vector
space.
Office for National Statistics (2020). Leading causes of
death, UK. publisher: GOV.uk.
Porter, M. F. (1980). An algorithm for suffix stripping. Pro-
gram, 40:211–218.
Reimers, N. (2021a). Ms marco cross-encoders —
sentence-transformers documentation.
Reimers, N. (2021b). Pretrained models — sentence-
transformers documentation.
Reimers, N. and Gurevych, I. (2019). Sentence-bert: Sen-
tence embeddings using siamese bert-networks.
Robertson, S., Walker, S., Hancock-Beaulieu, M. M., Gat-
ford, M., and Payne, A. (1996). Okapi at trec-4.
In The Fourth Text REtrieval Conference (TREC-4),
pages 73–96. Gaithersburg, MD: NIST.
Robertson, S., Walker, S., Jones, S., Hancock-Beaulieu,
M. M., and Gatford, M. (1995). Okapi at trec-3.
In Overview of the Third Text REtrieval Conference
(TREC-3), pages 109–126. Gaithersburg, MD: NIST.
Robertson, S. and Zaragoza, H. (2009). The probabilistic
relevance framework: Bm25 and beyond. Founda-
tions and Trends in Information Retrieval, 3(4):333–
389.
Saha, S. K., Prakash, A., and Majumder, M. (2019). “simi-
lar query was answered earlier”: processing of patient
authored text for retrieving relevant contents from
health discussion forum. Health Information Science
and Systems, 7.
Sanh, V., Debut, L., Chaumond, J., and Wolf, T. (2020).
Distilbert, a distilled version of bert: smaller, faster,
cheaper and lighter.
Schuster, M. and Nakajima, K. (2012). Japanese and korean
voice search. In International Conference on Acous-
tics, Speech and Signal Processing, pages 5149–5152.
Thakur, N., Reimers, N., R
¨
uckl
´
e, A., Srivastava, A., and
Gurevych, I. (2021). Beir: A heterogenous bench-
mark for zero-shot evaluation of information retrieval
models.
Trabelsi, M., Chen, Z., Davison, B. D., and Heflin, J.
(2021). Neural ranking models for document retrieval.
Trotman, A., Jia, X., and Crane, M. (2012). Towards an ef-
ficient and effective search engine. In OSIR@ SIGIR,
pages 40–47.
Trotman, A., Puurula, A., and Burgess, B. (2014). Im-
provements to bm25 and language models examined.
In Proceedings of the 2014 Australasian Document
Computing Symposium, ADCS ’14, page 58–65, New
York, NY, USA. Association for Computing Machin-
ery.
University of Manchester (2018). National Confidential In-
quiry into Suicide and Safety in Mental Health.
Vaswani, A., Shazeer, N., Parmar, N., Uszkoreit, J., Jones,
L., Gomez, A. N., Kaiser, L., and Polosukhin, I.
(2017). Attention is all you need.
Wang, A., Singh, A., Michael, J., Hill, F., Levy, O., and
Bowman, S. R. (2019). Glue: A multi-task bench-
mark and analysis platform for natural language un-
derstanding.
Wang, W., Wei, F., Dong, L., Bao, H., Yang, N., and Zhou,
M. (2020). Minilm: Deep self-attention distillation for
task-agnostic compression of pre-trained transform-
ers.
Zapf, A., Castell, S., Morawietz, L., and Karch, A. (2016).
Measuring inter-rater reliability for nominal data -
which coefficients and confidence intervals are appro-
priate? BMC Medical Research Methodology, 16.
Leveraging Out-of-the-Box Retrieval Models to Improve Mental Health Support
73