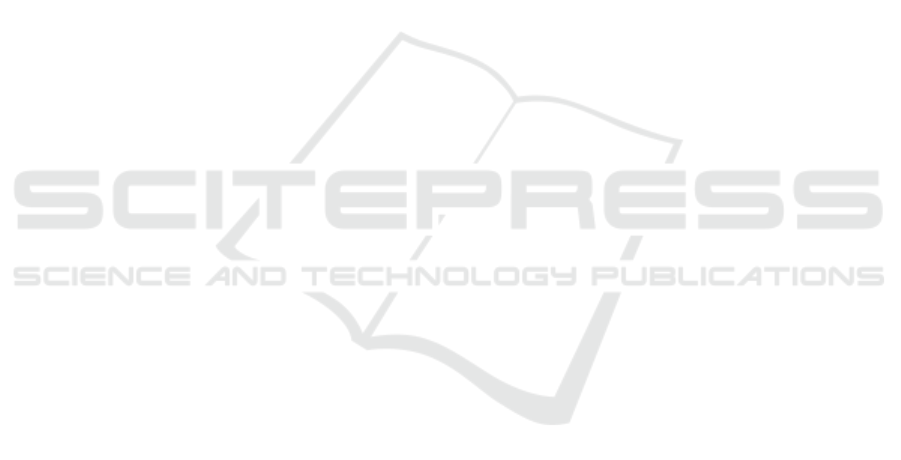
Sun, S., et al. (2019). Mmdetection: Open mm-
lab detection toolbox and benchmark. arXiv preprint
arXiv:1906.07155.
Delange, M., Aljundi, R., Masana, M., Parisot, S., Jia,
X., Leonardis, A., Slabaugh, G., and Tuytelaars, T.
(2021). A continual learning survey: Defying forget-
ting in classification tasks. IEEE Transactions on Pat-
tern Analysis and Machine Intelligence.
Dosovitskiy, A., Beyer, L., Kolesnikov, A., Weissenborn,
D., Zhai, X., Unterthiner, T., Dehghani, M., et al.
(2021). An image is worth 16x16 words: Transform-
ers for image recognition at scale. In International
Conference on Learning Representations.
Farquhar, S. and Gal, Y. (2018). Towards robust
evaluations of continual learning. arXiv preprint
arXiv:1805.09733.
Han, J., Liang, X., Xu, H., Chen, K., Hong, L., Mao, J., Ye,
C., Zhang, W., Li, Z., Liang, X., and Xu, C. (2021).
Soda10m: A large-scale 2d self/semi-supervised ob-
ject detection dataset for autonomous driving.
He, K., Zhang, X., Ren, S., and Sun, J. (2016). Deep resid-
ual learning for image recognition. In Proceedings of
the IEEE Conference on Computer Vision and Pattern
Recognition.
Hsu, Y.-C., Liu, Y.-C., Ramasamy, A., and Kira, Z. (2018).
Re-evaluating continual learning scenarios: A catego-
rization and case for strong baselines. arXiv preprint
arXiv:1810.12488.
Kirkpatrick, J., Pascanu, R., Rabinowitz, N., Veness, J.,
Desjardins, G., Rusu, A., Milan, K., et al. (2017).
Overcoming catastrophic forgetting in neural net-
works. Proceedings of the National Academy of Sci-
ences.
Liu, Z., Lin, Y., Cao, Y., Hu, H., Wei, Y., Zhang, Z., Lin,
S., and Guo, B. (2021). Swin transformer: Hierarchi-
cal vision transformer using shifted windows. In Pro-
ceedings of the IEEE/CVF International Conference
on Computer Vision.
Lomonaco, V., Pellegrini, L., Cossu, A., Carta, A., Graf-
fieti, G., Hayes, T. L., Lange, M. D., et al. (2021).
Avalanche: an end-to-end library for continual learn-
ing. In Proceedings of IEEE Conference on Computer
Vision and Pattern Recognition.
Lopez-Paz, D. and Ranzato, M. (2017). Gradient episodic
memory for continual learning. Advances in Neural
Information Processing Systems.
McCloskey, M. and Cohen, N. J. (1989). Catastrophic in-
terference in connectionist networks: The sequential
learning problem. In Psychology of Learning and Mo-
tivation.
Mirzadeh, S. I., Chaudhry, A., Yin, D., Nguyen, T., Pas-
canu, R., Gorur, D., and Farajtabar, M. (2022). Archi-
tecture matters in continual learning. arXiv preprint
arXiv:2202.00275.
Pan, S. J. and Yang, Q. (2009). A survey on transfer learn-
ing. IEEE Transactions on Knowledge and Data En-
gineering.
Peng, C., Zhao, K., and Lovell, B. C. (2020). Faster ilod: In-
cremental learning for object detectors based on faster
rcnn. Pattern Recognition Letters.
Peng, C., Zhao, K., Maksoud, S., Li, M., and Lovell, B. C.
(2021). Sid: Incremental learning for anchor-free ob-
ject detection via selective and inter-related distilla-
tion. Computer Vision and Image Understanding.
Pham, Q., Liu, C., and Hoi, S. (2022). Continual normaliza-
tion: Rethinking batch normalization for online con-
tinual learning. arXiv preprint arXiv:2203.16102.
Ramasesh, V. V., Lewkowycz, A., and Dyer, E. (2021). Ef-
fect of scale on catastrophic forgetting in neural net-
works. In International Conference on Learning Rep-
resentations.
Rebuffi, S.-A., Kolesnikov, A., Sperl, G., and Lampert,
C. H. (2017). icarl: Incremental classifier and rep-
resentation learning. In Proceedings of the IEEE Con-
ference on Computer Vision and Pattern Recognition.
Ren, S., He, K., Girshick, R., and Sun, J. (2015). Faster
r-cnn: Towards real-time object detection with region
proposal networks. Advances in Neural Information
Processing Systems.
Rusu, A. A., Rabinowitz, N. C., Desjardins, G., Soyer,
H., Kirkpatrick, J., Kavukcuoglu, K., Pascanu, R.,
and Hadsell, R. (2016). Progressive neural networks.
arXiv preprint arXiv:1606.04671.
Serra, J., Suris, D., Miron, M., and Karatzoglou, A. (2018).
Overcoming catastrophic forgetting with hard atten-
tion to the task. In International Conference on Ma-
chine Learning. PMLR.
Shin, H., Lee, J. K., Kim, J., and Kim, J. (2017). Contin-
ual learning with deep generative replay. Advances in
Neural Information Processing Systems.
Shmelkov, K., Schmid, C., and Alahari, K. (2017). In-
cremental learning of object detectors without catas-
trophic forgetting. In Proceedings of the IEEE/CVF
International Conference on Computer Vision.
Tian, Z., Shen, C., Chen, H., and He, T. (2019). Fcos: Fully
convolutional one-stage object detection. In Proceed-
ings of the IEEE/CVF International Conference on
Computer Vision.
Van de Ven, G. M. and Tolias, A. S. (2019). Three
scenarios for continual learning. arXiv preprint
arXiv:1904.07734.
Yu, F., Chen, H., Wang, X., Xian, W., Chen, Y., Liu, F.,
Madhavan, V., and Darrell, T. (2020). Bdd100k: A
diverse driving dataset for heterogeneous multitask
learning. In Proceedings of the IEEE Conference on
Computer Vision and Pattern Recognition.
Zenke, F., Poole, B., and Ganguli, S. (2017). Continual
learning through synaptic intelligence. In Interna-
tional Conference on Machine Learning.
Zhu, X., Su, W., Lu, L., Li, B., Wang, X., and Dai, J. (2021).
Deformable detr: Deformable transformers for end-
to-end object detection. International Conference on
Learning Representations.
Severity of Catastrophic Forgetting in Object Detection for Autonomous Driving
269