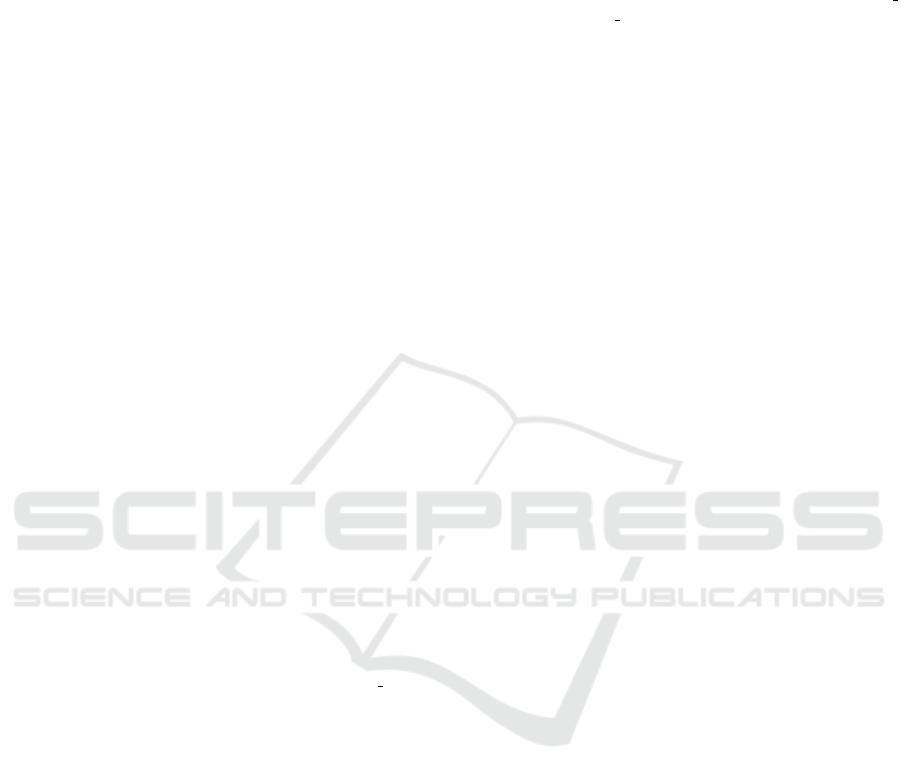
Fari
˜
na, J., Segura, M., G
´
omez, V., Vila, D., and Veiga,
C. (2021). Using explainable machine learning to
improve intensive care unit alarm systems. Sensors
(Basel, Switzerland), 21(21).
Harutyunyan, H., Khachatrian, H., Kale, D. C., Ver Steeg,
G., and Galstyan, A. (2019). Multitask learning and
benchmarking with clinical time series data. Scientific
Data, 6(1):96.
Johnson, A., Bulgarelli, L., Pollard, T., Horng, S., Celi,
L. A., and Mark, R. (2021). Mimic-iv. https://doi.org/
10.13026/S6N6-XD98.
Ke, G., Meng, Q., Finley, T., Wang, T., Chen, W., Ma, W.,
Ye, Q., and Liu, T.-Y. (2017). Lightgbm: A highly
efficient gradient boosting decision tree. Advances
in neural information processing systems, 30:3146–
3154.
Kong, S. H., Ahn, D., Kim, B. R., Srinivasan, K., Ram, S.,
Kim, H., Hong, A. R., Kim, J. H., Cho, N. H., and
Shin, C. S. (2020). A novel fracture prediction model
using machine learning in a community-based cohort.
JBMR plus, 4(3):e10337.
Lundberg, S. M. and Lee, S.-I. (2017). A unified approach
to interpreting model predictions. In I. Guyon, U. Von
Luxburg, S. Bengio, H. Wallach, R. Fergus, S. Vish-
wanathan, and R. Garnett, editors, Advances in Neural
Information Processing Systems, volume 30. Curran
Associates, Inc.
McIlwraith, D., Marmanis, H., and Babenko, D. (2016). Al-
gorithms of the intelligent web. Manning Publications
Co, Shelter Island NY, second edition edition.
NBER (2022). Icd-9-cm to and from icd-10-cm and icd-
10-pcs crosswalk or general equivalence mappings.
https://www.nber.org/research/data/icd-9-cm-and-
icd-10-cm-and-icd-10-pcs-crosswalk-or-general-
equivalence-mappings. Last checked on Jun 14,
2022.
NIH (2022). Overview of snomed ct. https:
//www.nlm.nih.gov/healthit/snomedct/snomed
overview.html. Last checked on Mai 31, 2022.
Pelter, M. M., Suba, S., Sandoval, C., Z
`
egre-Hemsey, J. K.,
Berger, S., Larsen, A., Badilini, F., and Hu, X. (2020).
Actionable ventricular tachycardia during in-hospital
ecg monitoring and its impact on alarm fatigue. Crit-
ical Pathways in Cardiology: A Journal of Evidence-
Based Medicine, 19(2):79–86.
Pollard, T. J., Johnson, A. E. W., Raffa, J. D., Celi, L. A.,
Mark, R. G., and Badawi, O. (2018). The eicu col-
laborative research database, a freely available multi-
center database for critical care research. Scientific
Data, 5:180178.
Prokhorenkova, L., Gusev, G., Vorobev, A., Dorogush,
A. V., and Gulin, A. (2017). Catboost: unbiased
boosting with categorical features. https://arxiv.org/
pdf/1706.09516.
Schmid, F., Goepfert, M. S., Kuhnt, D., Eichhorn, V.,
Diedrichs, S., Reichenspurner, H., Goetz, A. E., and
Reuter, D. A. (2011). The wolf is crying in the operat-
ing room: patient monitor and anesthesia workstation
alarming patterns during cardiac surgery. Anesthesia
and analgesia, 112(1):78–83.
Sendelbach, S. and Funk, M. (2013). Alarm fatigue: a pa-
tient safety concern. AACN advanced critical care,
24(4):378–86; quiz 387–8.
SHAP (2022). beeswarm plot — shap latest documen-
tation. https://shap.readthedocs.io/en/latest/example
notebooks/api examples/plots/beeswarm.html. Last
checked on Sep 16, 2022.
Shapley, L. S. (1953). 17. a value for n-person games. In
Kuhn, H. W. and Tucker, A. W., editors, Contributions
to the Theory of Games (AM-28), Annals of Mathe-
matics Studies, pages 307–318. Princeton University
Press, Princeton, NJ.
SNOMED International (2022). Snomed
ct - clinical finding (finding). https:
//browser.ihtsdotools.org/?perspective=
full&conceptId1=404684003&edition=MAIN/
2022-05-31&release=&languages=en. Last checked
on Mai 31, 2022.
Suba, S., Sandoval, C. P., Z
`
egre-Hemsey, J. K., Hu, X.,
and Pelter, M. M. (2019). Contribution of electrocar-
diographic accelerated ventricular rhythm alarms to
alarm fatigue. American journal of critical care : an
official publication, American Association of Critical-
Care Nurses, 28(3):222–229.
Torres, F. (2022). Laboratory for computational physiology.
https://lcp.mit.edu/mimic. Last checked on Mai 28,
2022.
WHO (2022). International classification of diseases
(icd). https://www.who.int/standards/classifications/
classification-of-diseases. Last checked on Mai 31,
2022.
Yu, G., Li, Z., Li, S., Liu, J., Sun, M., Liu, X., Sun, F.,
Zheng, J., Li, Y., Yu, Y., Shu, Q., and Wang, Y. (2020).
The role of artificial intelligence in identifying asthma
in pediatric inpatient setting. Annals of translational
medicine, 8(21):1367.
Zhao, Q.-Y., Liu, L.-P., Luo, J.-C., Luo, Y.-W., Wang, H.,
Zhang, Y.-J., Gui, R., Tu, G.-W., and Luo, Z. (2020).
A machine-learning approach for dynamic prediction
of sepsis-induced coagulopathy in critically ill pa-
tients with sepsis. Frontiers in medicine, 7:637434.
Recommender System for Alarm Thresholds in Medical Patient Monitors
85