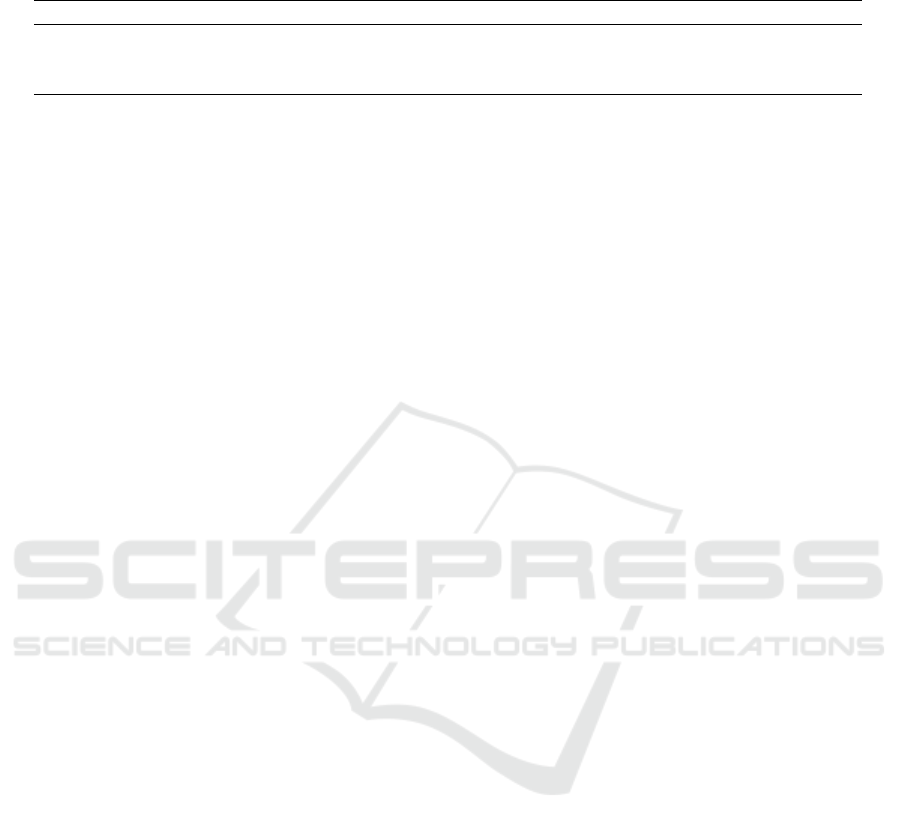
Table 12: Average of the 50 last epochs obtained with the final threshold. These results are presented to comment on how
these changes affect overfitting.
Model Dataset γ Avg. Acc. Std. APCER Avg. BPCER Avg.
FeatherNet A CASIA-SURF 3 85.746 1.003 0.160 0.105
FeatherNet A GRAFTSET - 10% Both 3 87.478 0.401 0.113 0.155
FeatherNet A WMCA 3 88.446 1.012 0 0.504
cases both in presentation attacks and ambient condi-
tions of WMCA. Not only would this dataset be much
closer to what a real day-to-day use of a PAD appli-
cation would encounter, it would also benefit the gen-
eralization of models developed with it. Hence mean-
ing, that with a more diverse dataset, the number of
studies that deviate from the binary approach to live-
ness detection by categorizing each attack individu-
ally could grow with different insights on what dif-
ferent attacks are more challenging with what modal-
ities.
On a final note, and trying to be straightforward
on the best approach regarding the information given
to the model, on a regular application, the conclusion
was moving away from depth or infra red, on both
direct input, or only as a supervision for the model,
as well as sticking with the regular color informa-
tion, proving that the way the model is constructed
is of great importance. The building of a new model
that, like FeatherNets, tries to be as light as possible,
achieving great results and not requiring extra infor-
mation could benefit from some of the considerations
made here. This model would require a new approach
to its construction since many of the choices made
for FeatherNets were taken considering the depth in-
put. Since this new theoretical model would return
to the more common use RGB images but forego the
supervision provided by the extra modalities (depth,
infra red), techniques that were successful for these
types of models might not benefit this one, being per-
haps beneficial to consider the approaches used before
the extra modalities were available while considering
not only the more complex dataset as well as the ap-
proaches demonstrated in this paper.
REFERENCES
Atoum, Y., Liu, Y., Jourabloo, A., and Liu, X. (2017). Face
anti-spoofing using patch and depth-based cnns. 2017
IEEE International Joint Conference on Biometrics
(IJCB), pages 319–328.
Chingovska, I., Anjos, A. R. d., and Marcel, S. (2014).
Biometrics evaluation under spoofing attacks. IEEE
Transactions on Information Forensics and Security,
9(12):2264–2276.
George, A., Mostaani, Z., Geissenbuhler, D., Nikisins, O.,
Anjos, A., and Marcel, S. (2020). Biometric face pre-
sentation attack detection with multi-channel convo-
lutional neural network. IEEE Transactions on Infor-
mation Forensics and Security, 15.
Hao, H., Pei, M., and Zhao, M. (2019). Face liveness detec-
tion based on client identity using siamese network.
Pattern Recognition and Computer Vision: Second
Chinese Conference, PRCV 2019, pages 172–180.
He, K., Zhang, X., Ren, S., and Sun, J. (2016). Deep resid-
ual learning for image recognition. 2016 IEEE Con-
ference on Computer Vision and Pattern Recognition
(CVPR), pages 770–778.
Janocha, K. and Czarnecki, W. M. (2017). On loss func-
tions for deep neural networks in classification. Pro-
ceedings of the Theoretical Foundations of Machine
Learning 2017 (TFML 2017).
Kim, T., Kim, Y., Kim, I., and Kim, D. (2019). Basn: En-
riching feature representation using bipartite auxiliary
supervisions for face anti-spoofing. 2019 IEEE/CVF
International Conference on Computer Vision Work-
shop (ICCVW), pages 494–503.
Lin, T. Y., Goyal, P., Girshick, R., He, K., and Dollar, P.
(2020). Focal loss for dense object detection. IEEE
Transactions on Pattern Analysis and Machine Intel-
ligence, 42.
Liu, Y., Stehouwer, J., Jourabloo, A., and Liu, X. (2019).
Deep tree learning for zero-shot face anti-spoofing.
Proceedings of the IEEE Computer Society Confer-
ence on Computer Vision and Pattern Recognition,
2019-June.
Menotti, D., Chiachia, G., Pinto, A., Schwartz, W. R.,
Pedrini, H., Falcao, A. X., and Rocha, A. (2015).
Deep representations for iris, face, and fingerprint
spoofing detection. IEEE Transactions on Informa-
tion Forensics and Security, 10:864–879.
Sandler, M., Howard, A., Zhu, M., Zhmoginov, A., and
Chen, L.-C. (2018). Mobilenetv2: Inverted residuals
and linear bottlenecks. 2018 IEEE/CVF Conference
on Computer Vision and Pattern Recognition, pages
4510–4520.
Sun, W., Song, Y., Chen, C., Huang, J., and Kot, A. C.
(2020). Face spoofing detection based on local ternary
label supervision in fully convolutional networks.
IEEE Transactions on Information Forensics and Se-
curity, 15:3181–3196.
Wayman, J. L. (2007). 10 - the scientific development of
biometrics over the last 40 years. In Leeuw, K. D.
and Bergstra, J., editors, The History of Information
Security, pages 263–274. Elsevier Science B.V., Am-
sterdam.
Xu, X., Xiong, Y., and Xia, W. (2020). On improving tem-
poral consistency for online face liveness detection.
arXiv preprints arXiv:2006.06756.
ICPRAM 2023 - 12th International Conference on Pattern Recognition Applications and Methods
72