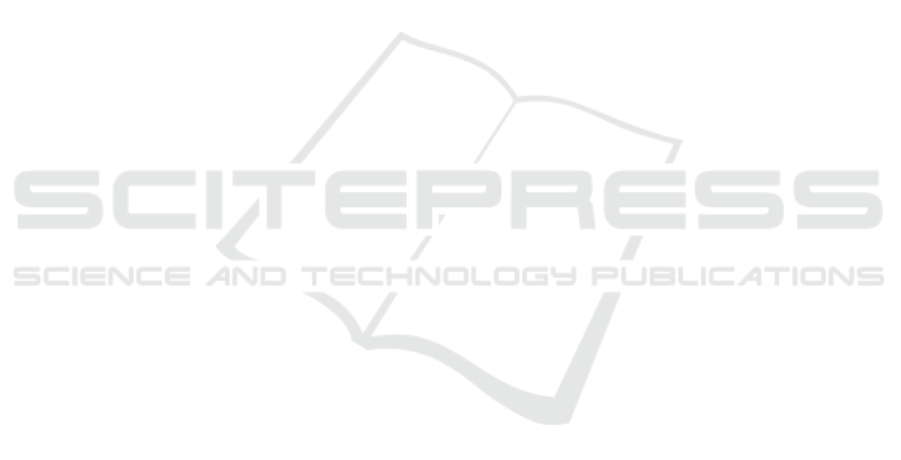
main adaptation techniques, such as based on adver-
sarial networks, to enhance the data representation.
Finally, we can evaluate our proposed approach by
employing different matching algorithms to measure
similarity between embedding features.
REFERENCES
Barakat, B. K., Alasam, R., and El-Sana, J. (2018). Word
spotting using convolutional siamese network. In
2018 13th IAPR International Workshop on Document
Analysis Systems (DAS), pages 229–234. IEEE.
Can, Y. S. and Kabadayı, M. E. (2020). Automatic cnn-
based arabic numeral spotting and handwritten digit
recognition by using deep transfer learning in ottoman
population registers. Applied Sciences, 10(16):5430.
Cui, Y., Song, Y., Sun, C., Howard, A., and Belongie, S.
(2018). Large scale fine-grained categorization and
domain-specific transfer learning. In Proceedings of
the IEEE conference on computer vision and pattern
recognition, pages 4109–4118.
Deng, J., Berg, A. C., and Fei-Fei, L. (2011). Hierarchical
semantic indexing for large scale image retrieval. In
CVPR 2011, pages 785–792. IEEE.
Everingham, M., Van Gool, L., Williams, C. K., Winn, J.,
and Zisserman, A. (2010). The pascal visual object
classes (voc) challenge. International journal of com-
puter vision, 88(2):303–338.
Fathallah, A., Khedher, M. I., El-Yacoubi, M. A., and
Amara, N. E. B. (2020). Evaluation of feature-
embedding methods for word spotting in historical
arabic documents. In 2020 17th International Multi-
Conference on Systems, Signals & Devices (SSD),
pages 34–39. IEEE.
Fathallah, A., Khedher, M. I., El-Yacoubi, M. A., and Es-
soukri Ben Amara, N. (2019). Triplet cnn-based word
spotting of historical arabic documents. 27th Interna-
tional Conference on Neural Information Processing
(ICONIP), 15(2):44–51.
Gurjar, N., Sudholt, S., and Fink, G. A. (2018). Learn-
ing deep representations for word spotting under weak
supervision. In 2018 13th IAPR International Work-
shop on Document Analysis Systems (DAS), pages 7–
12. IEEE.
Kassis, M., Abdalhaleem, A., Droby, A., Alaasam, R., and
El-Sana, J. (2017). Vml-hd: The historical arabic
documents dataset for recognition systems. In Ara-
bic Script Analysis and Recognition (ASAR), 2017 1st
International Workshop on, pages 11–14. IEEE.
Khayyat, M. and Suen, C. Y. (2018). Improving word
spotting system performance using ensemble classi-
fier combination methods. In 2018 16th International
Conference on Frontiers in Handwriting Recognition
(ICFHR), pages 229–234. IEEE.
Khayyat, M. M. and Elrefaei, L. A. (2020). Towards author
recognition of ancient arabic manuscripts using deep
learning: A transfer learning approach. International
Journal of Computing and Digital Systems, 9(5):1–18.
Konidaris, T., Kesidis, A. L., and Gatos, B. (2016). A
segmentation-free word spotting method for historical
printed documents. Pattern analysis and applications,
19(4):963–976.
Mhiri, M., Desrosiers, C., and Cheriet, M. (2019). Word
spotting and recognition via a joint deep embedding
of image and text. Pattern Recognition, 88:312–320.
Mohammed, H. H., Subramanian, N., Al-Maadeed, S., and
Bouridane, A. (2022). Wsnet–convolutional neural
network-based word spotting for arabic and english
handwritten documents. TEM.
Rabaev, I., Barakat, B. K., Churkin, A., and El-Sana, J.
(2020). The hhd dataset. In 2020 17th International
Conference on Frontiers in Handwriting Recognition
(ICFHR), pages 228–233. IEEE.
Rajeswar, S., Rodriguez, P., Singhal, S., Vazquez, D., and
Courville, A. (2022). Multi-label iterated learning for
image classification with label ambiguity. In Proceed-
ings of the IEEE/CVF Conference on Computer Vision
and Pattern Recognition, pages 4783–4793.
Rath, T. M. and Manmatha, R. (2007). Word spotting for
historical documents. International Journal of Docu-
ment Analysis and Recognition (IJDAR), 9(2-4):139–
152.
Schroff, F., Kalenichenko, D., and Philbin, J. (2015).
Facenet: A unified embedding for face recognition
and clustering. In Proceedings of the IEEE conference
on computer vision and pattern recognition, pages
815–823.
Sudholt, S. and Fink, G. A. (2018). Attribute cnns for
word spotting in handwritten documents. Interna-
tional journal on document analysis and recognition
(ijdar), 21(3):199–218.
Westphal, F., Grahn, H., and Lavesson, N. (2020). Repre-
sentative image selection for data efficient word spot-
ting. In International Workshop on Document Analy-
sis Systems, pages 383–397. Springer.
Ye, M. and Shen, J. (2020). Probabilistic structural latent
representation for unsupervised embedding. In Pro-
ceedings of the IEEE/CVF conference on computer vi-
sion and pattern recognition, pages 5457–5466.
Zagoris, K., Pratikakis, I., and Gatos, B. (2014).
Segmentation-based historical handwritten word spot-
ting using document-specific local features. In 2014
14th International Conference on Frontiers in Hand-
writing Recognition, pages 9–14. IEEE.
Transfer Learning for Word Spotting in Historical Arabic Documents Based Triplet-CNN
527