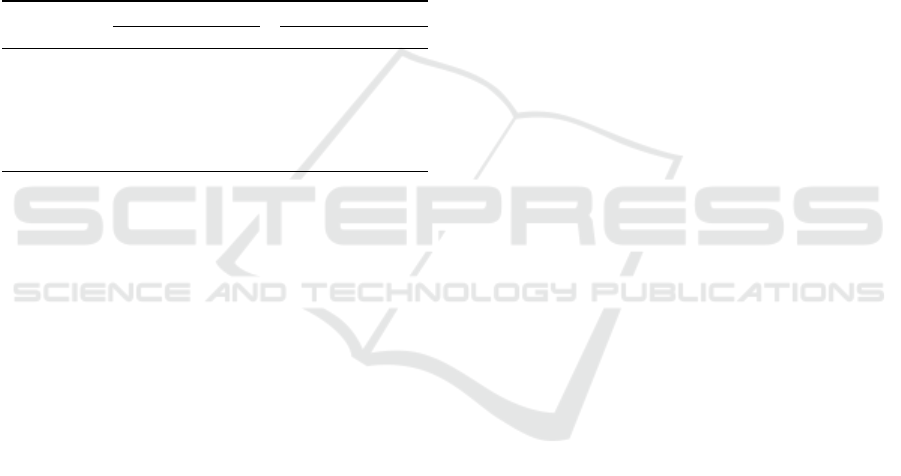
5.2 All Diagnoses Classification
This section deals with the multi-label all diagnoses
classification scenario. The results are summarised in
Table 4. We tested the same models as in the single-
label scenario. The only modification is using the sig-
moid activation function in the classification layer and
binary cross-entropy loss function. In this scenario,
HA-GRU is the best-performing model in terms of
both micro- and macro-averaged values. The results
indicate that the attention mechanism used in this net-
work is the most suitable for the task and outperforms
the more complex Electra model as well as the CNN
networks.
Table 4: Macro- and micro-averaged precision, recall and
F-measure for the all diagnoses classification scenario in
[%].
Model
Macro Micro
F1 P R F1 P R
MLP (base.) 31.6 43.8 26.9 68.7 78.3 61.2
1-8 CNN 512 35.6 46.8 31.7 71.8 80.5 64.8
CNN 1024 34.2 43.9 31.3 72.0 81.3 64.4
ELECTRA 20.0 27.1 17.5 70.6 83.3 61.3
DocChar 33.9 47.1 29.6 65.2 80.5 54.8
HA-GRU 41.8 50.3 38.3 75.1 79.7 71.1
6 CONCLUSIONS AND FUTURE
WORK
In this study, we have performed a comparative eval-
uation of several state-of-the-art models for the task
of medical report classification in Czech. To the best
of our knowledge, it is the first attempt at automatic
diagnosis coding on Czech data.
The results for the main diagnosis scenario indi-
cate that the models perform comparably and slightly
outperform the baseline which proved to be relatively
strong.
In the second scenario, the more sophisticated
models obtained better results compared to the base-
line. The HA-GRU model proved to be the best one
in this scenario.
We can also conclude that the results of the best
HA-GRU model are good enough to be integrated into
the target system which will significantly reduce the
workload of the operators and thus also saves the time
and money.
In the future work, we would like to improve the
architecture of the HA-GRU model and adjust it for
utilisation of other types of clinical reports such as
epicrisis etc. and improve the performance.
ACKNOWLEDGEMENTS
This work has been partly supported by Grant No.
SGS-2022-016 Advanced methods of data processing
and analysis.
REFERENCES
Baumel, T., Nassour-Kassis, J., Cohen, R., Elhadad, M.,
and Elhadad, N. (2018). Multi-label classification of
patient notes: case study on icd code assignment. In
Workshops at the thirty-second AAAI conference on
artificial intelligence.
Chalkidis, I., Fergadiotis, M., Malakasiotis, P., Aletras, N.,
and Androutsopoulos, I. (2019). Extreme multi-label
legal text classification: A case study in eu legislation.
arXiv preprint arXiv:1905.10892.
Cho, K., Van Merri
¨
enboer, B., Bahdanau, D., and Bengio,
Y. (2014). On the properties of neural machine trans-
lation: Encoder-decoder approaches. arXiv preprint
arXiv:1409.1259.
Clark, K., Luong, M.-T., Le, Q. V., and Manning, C. D.
(2020). ELECTRA: Pre-training text encoders as dis-
criminators rather than generators. In ICLR.
El Boukkouri, H., Ferret, O., Lavergne, T., Noji, H.,
Zweigenbaum, P., and Tsujii, J. (2020). Charac-
terBERT: Reconciling ELMo and BERT for word-
level open-vocabulary representations from charac-
ters. In Proceedings of the 28th International Confer-
ence on Computational Linguistics, pages 6903–6915,
Barcelona, Spain (Online). International Committee
on Computational Linguistics.
Gu, P., Yang, S., Li, Q., and Wang, J. (2021). Disease cor-
relation enhanced attention network for icd coding. In
2021 IEEE International Conference on Bioinformat-
ics and Biomedicine (BIBM), pages 1325–1330, Los
Alamitos, CA, USA. IEEE Computer Society.
Johnson, A. E., Pollard, T. J., Shen, L., Lehman, L.-w. H.,
Feng, M., Ghassemi, M., Moody, B., Szolovits, P.,
Anthony Celi, L., and Mark, R. G. (2016). Mimic-
iii, a freely accessible critical care database. Scientific
data, 3(1):1–9.
Kim, Y. (2014). Convolutional neural networks for sentence
classification. arXiv preprint arXiv:1408.5882.
Koci
´
an, M., N
´
aplava, J.,
ˇ
Stancl, D., and Kadlec, V. (2022).
Siamese bert-based model for web search relevance
ranking evaluated on a new czech dataset. Proceed-
ings of the AAAI Conference on Artificial Intelligence,
36(11):12369–12377.
Lenc, L. and Kr
´
al, P. (2016). Deep neural networks for
czech multi-label document classification. In Interna-
tional Conference on Intelligent Text Processing and
Computational Linguistics, pages 460–471. Springer.
McAuley, J. and Leskovec, J. (2013). Hidden factors and
hidden topics: understanding rating dimensions with
review text. In Proceedings of the 7th ACM conference
on Recommender systems, pages 165–172.
ICAART 2023 - 15th International Conference on Agents and Artificial Intelligence
232