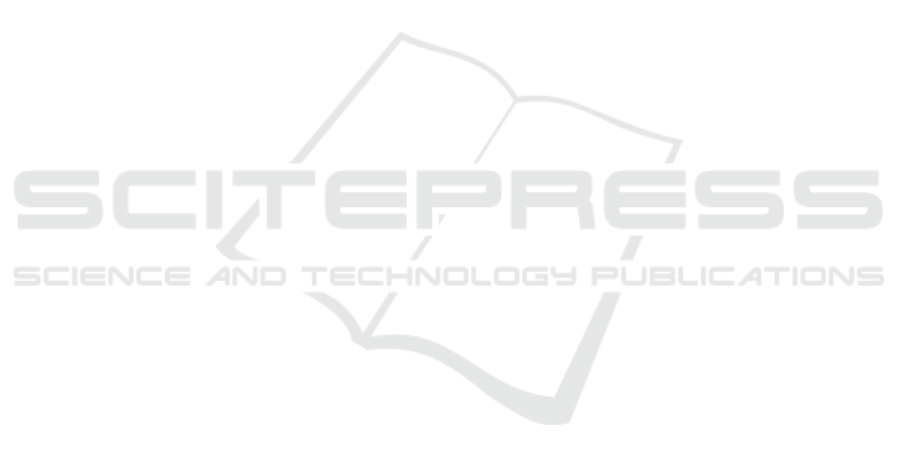
Regarding future work, we intend to test the per-
formance of the fine-tuning in the bias of the invisible
layer b or even fine-tune the parameters simultane-
ously, e.g. a and b.
ACKNOWLEDGEMENTS
The authors are grateful to FAPESP grants
#2013/07375-0, #2014/12236-1, #2019/07665-4,
#2019/18287-0, #2019/02205-5 and, #2021/05516-1,
and CNPq grants 308529/2021-9 and 427968/2018-6.
REFERENCES
Ackley, D., Hinton, G., and Sejnowski, T. J. (1988). A
learning algorithm for boltzmann machines. In Waltz,
D. and Feldman, J., editors, Connectionist Models and
Their Implications: Readings from Cognitive Science,
pages 285–307. Ablex Publishing Corp., Norwood,
NJ, USA.
Chou, J.-S. and Truong, D.-N. (2021). A novel metaheuris-
tic optimizer inspired by behavior of jellyfish in ocean.
Applied Mathematics and Computation, 389:125535.
Hatamlou, A. (2018). Solving travelling salesman problem
using black hole algorithm. Soft Computing, 22:8167–
8175.
Hembecker, F., Lopes, H. S., and Godoy, W. (2007). Par-
ticle swarm optimization for the multidimensional
knapsack problem. In Beliczynski, B., Dzielinski,
A., Iwanowski, M., and Ribeiro, B., editors, Adaptive
and Natural Computing Algorithms, pages 358–365,
Berlin, Heidelberg. Springer Berlin Heidelberg.
Hinton, G. E. (2002). Training products of experts by min-
imizing contrastive divergence. Neural Computation,
14(8):1771–1800.
Hinton, G. E. (2012). A practical guide to training restricted
Boltzmann machines. In Montavon, G., Orr, G., and
Muller, K.-R., editors, Neural Networks: Tricks of the
Trade, volume 7700 of Lecture Notes in Computer
Science, pages 599–619. Springer Berlin Heidelberg.
Kennedy, J. and Eberhart, R. C. (2001). Swarm Intelli-
gence. Morgan Kaufmann Publishers Inc., San Fran-
cisco, USA.
Kuremoto, T., Kimura, S., Kobayashi, K., and Obayashi,
M. (2012). Time series forecasting using restricted
boltzmann machine. In International Conference on
Intelligent Computing, pages 17–22. Springer.
Papa, J. P., Rosa, G. H., Costa, K. A. P., Marana, A. N.,
Scheirer, W., and Cox, D. D. (2015). On the model
selection of bernoulli restricted boltzmann machines
through harmony search. In Proceedings of the
Genetic and Evolutionary Computation Conference,
pages 1449–1450, New York, NY, USA. ACM.
Papa, J. P., Scheirer, W., and Cox, D. D. (2016). Fine-tuning
deep belief networks using harmony search. Applied
Soft Computing, 46:875–885.
Passos, L. A., de Souza Jr, L. A., Mendel, R., Ebigbo, A.,
Probst, A., Messmann, H., Palm, C., and Papa, J. P.
(2019). Barrett’s esophagus analysis using infinity re-
stricted Boltzmann machines. Journal of Visual Com-
munication and Image Representation.
Wang, K.-P., Huang, L., Zhou, C.-G., and Pang, W. (2003).
Particle swarm optimization for traveling salesman
problem. In Proceedings of the 2003 International
Conference on Machine Learning and Cybernetics
(IEEE Cat. No.03EX693), volume 3, pages 1583–
1585.
Wilcoxon, F. (1945). Individual comparisons by ranking
methods. Biometrics Bulletin, 1(6):80–83.
Fine-Tuning Restricted Boltzmann Machines Using No-Boundary Jellyfish
73