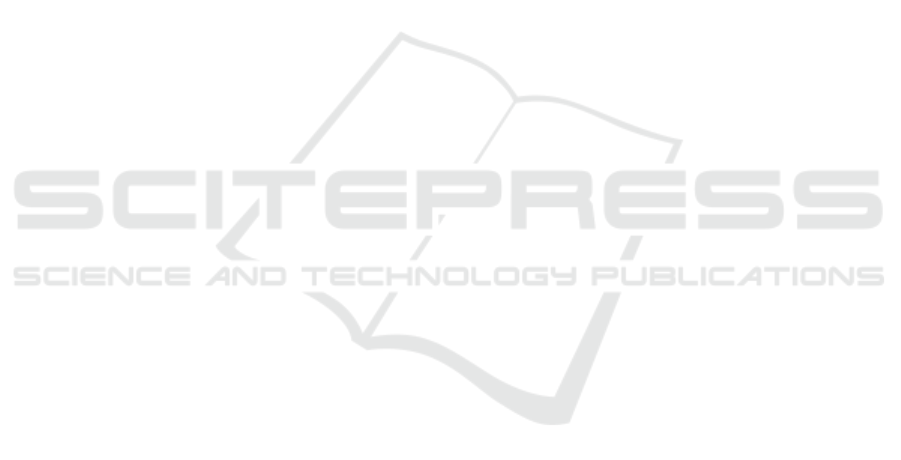
This line of research presents numerous intrigu-
ing opportunities in healthcare scenarios. This pro-
posal presents an opportunity to optimize pathways
of diagnosis and prognosis and develop personalized
treatment strategies by creating and utilizing larger
datasets. Furthermore, analyzing pictures of earlobes
for non-invasive DELC detection is among the most
important applications. Besides, monitoring ears dur-
ing aging is possible and may provide patient-specific
insight into current health and alert medical staff to
risk situations.
ACKNOWLEDGEMENTS
We want to acknowledge Dr. Cecilia Meiler-
Rodr
´
ıguez for her creative suggestions and inspiring
ideas. This work is partially funded by the ULPGC
under project ULPGC2018-08, the Spanish Ministry
of Economy and Competitiveness (MINECO) under
project RTI2018-093337-B-I00, the Spanish Ministry
of Science and Innovation under projects PID2019-
107228RB-I00 and PID2021-122402OB-C22, and
by the ACIISI-Gobierno de Canarias and European
FEDER funds under projects ProID2020010024,
ProID2021010012 and ULPGC Facilities Net and
Grant EIS 2021 04.
REFERENCES
Alshazly, H., Linse, C., Barth, E., and Martinetz, T. (2019).
Handcrafted versus cnn features for ear recognition.
Symmetry, 11(12).
Ardila, D., Kiraly, A., Bharadwaj, S., Choi, B., and Shetty,
S. (2019). End-to-end lung cancer screening with
three-dimensional deep learning on low-dose chest
computed tomography. Nat Med, 25:954–961.
Boudoulas, K., Triposkiadis, F., Geleris, P., and Boudoulas,
H. (2016). Coronary Atherosclerosis: Pathophysio-
logic Basis for Diagnosis and Management. Prog.
Cardiovasc. Dis, 58:676–692.
Buslaev, A., Iglovikov, V. I., Khvedchenya, E., Parinov, A.,
Druzhinin, M., and Kalinin, A. A. (2020). Albumen-
tations: Fast and flexible image augmentations. Infor-
mation, 11(2).
Castrill
´
on-Santana, M., Lorenzo-Navarro, J., Travieso-
Gonz
´
alez, C. M., Freire-Obreg
´
on, D., and Alonso-
Hern
´
andez, J. B. (2018). Evaluation of local descrip-
tors and cnns for non-adult detection in visual content.
Pattern Recognition Letters, 113:10–18.
CDC (2022). About Multiple Cause of Death, 1999–2020.
Cha, D., Pae, C., Seong, S.-B., Choi, J. Y., and Park, H.-J.
(2019). Automated diagnosis of ear disease using en-
semble deep learning with a big otoendoscopy image
database. EBioMedicine, 45:606–614.
Chollet, F. (2016). Xception: Deep learning with depthwise
separable convolutions. CoRR, abs/1610.02357.
Deng, J., Dong, W., Socher, R., Li, L.-J., Li, K., and Fei-
Fei, L. (2009). Imagenet: A large-scale hierarchical
image database. In 2009 IEEE Conference on Com-
puter Vision and Pattern Recognition, pages 248–255.
Emer
ˇ
si
ˇ
c,
ˇ
Z.,
ˇ
Struc, V., and Peer, P. (2017). Ear recogni-
tion: More than a survey. Neurocomputing, 255:26–
39. Bioinspired Intelligence for machine learning.
Frank, S. (1973). Aural sign of coronary-artery disease.
IEEE Transactions on Medical Imaging, 289(6):327–
328.
Freire-Obreg
´
on, D., Barra, P., Castrill
´
on-Santana, M., and
de Marsico, M. (2022). Inflated 3D ConvNet context
analysis for violence detection. Machine Vision and
Applications, 33(15).
Freire-Obreg
´
on, D., Rosales-Santana, K., Mar
´
ın-Reyes,
P. A., Penate-Sanchez, A., Lorenzo-Navarro, J., and
Castrill
´
on-Santana, M. (2021). Improving user veri-
fication in human-robot interaction from audio or im-
age inputs through sample quality assessment. Pattern
Recognition Letters, 149:179–184.
Gald
´
amez, P. L., Raveane, W., and Gonz
´
alez Arrieta, A.
(2017). A brief review of the ear recognition process
using deep neural networks. Journal of Applied Logic,
24:62–70. SI:SOCO14.
He, K., Zhang, X., Ren, S., and Sun, J. (2016). Deep resid-
ual learning for image recognition. In 2016 IEEE
Conf. on Computer Vision and Pattern Recognition,
pages 770–778.
Hirano, H., Katsumata, R., Futagawa, M., and Higashi, Y.
(2016). Towards view-invariant expression analysis
using analytic shape manifolds. In 2016 IEEE Engi-
neering in Medicine and Biology Society, pages 2374–
2377.
Howard, A. G., Zhu, M., Chen, B., Kalenichenko, D.,
Wang, W., Weyand, T., Andreetto, M., and Adam,
H. (2017). Mobilenets: Efficient convolutional neu-
ral networks for mobile vision applications. CoRR,
abs/1704.04861.
Huang, G., Liu, Z., van der Maaten, L., and Weinberger,
K. Q. (2016). Densely connected convolutional net-
works. CoRR, abs/1608.06993.
Isgum, I., Prokop, M., Niemeijer, M., Viergever, M. A.,
and van Ginneken, B. (2012). Automatic coro-
nary calcium scoring in low-dose chest computed to-
mography. IEEE Transactions on Medical Imaging,
31(12):2322–2334.
Kingma, D. P. and Ba, J. (2015). Adam: A method for
stochastic optimization. In 2015 Int. Conf. on Learn-
ing Representations.
Lee, M. C. H., Petersen, K., Pawlowski, N., Glocker, B.,
and Schaap, M. (2019). TeTrIS: Template Trans-
former Networks for Image Segmentation With Shape
Priors. IEEE Transactions on Medical Imaging,
38(11):2596–2606.
Mallinson, T. and Brooke, D. (2017). Limited diagnostic
potential of diagonal earlobe crease. Annals of emer-
gency medicine, 70(4):602–603.
ICPRAM 2023 - 12th International Conference on Pattern Recognition Applications and Methods
80