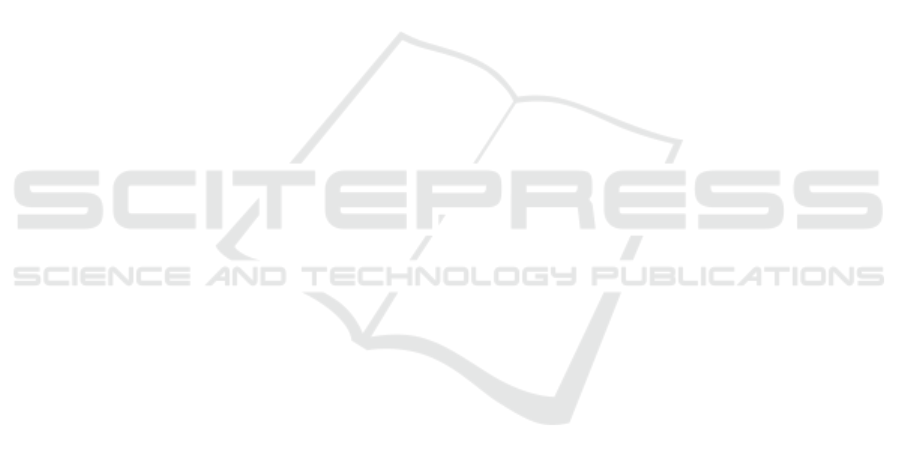
automatically de-identified corpus of french ehrs? In
Proceedings of the Sixth International Workshop on
Health Text Mining and Information Analysis, pages
31–39.
Hanslo, R. (2021). Deep learning transformer archi-
tecture for named entity recognition on low re-
sourced languages: State of the art results. CoRR,
abs/2111.00830.
Holohan, N., Leith, D. J., and Mason, O. (2017). Optimal
differentially private mechanisms for randomised re-
sponse. IEEE Transactions on Information Forensics
and Security, 12(11):2726–2735.
Huang, K., Altosaar, J., and Ranganath, R. (2019). Clinical-
bert: Modeling clinical notes and predicting hospital
readmission. arXiv preprint arXiv:1904.05342.
Johnson, A. E., Pollard, T. J., Shen, L., Lehman, L.-w. H.,
Feng, M., Ghassemi, M., Moody, B., Szolovits, P.,
Anthony Celi, L., and Mark, R. G. (2016). Mimic-
iii, a freely accessible critical care database. Scientific
data, 3(1):1–9.
Kersloot, M. G., van Putten, F. J., Abu-Hanna, A., Cornet,
R., and Arts, D. L. (2020). Natural language pro-
cessing algorithms for mapping clinical text fragments
onto ontology concepts: a systematic review and rec-
ommendations for future studies. Journal of biomedi-
cal semantics, 11(1):1–21.
Kumar, V., Stubbs, A., Shaw, S., and Uzuner,
¨
O. (2015).
Creation of a new longitudinal corpus of clinical nar-
ratives. Journal of biomedical informatics, 58:S6–
S10.
Lafferty, J. D., McCallum, A., and Pereira, F. C. N. (2001).
Conditional random fields: Probabilistic models for
segmenting and labeling sequence data. In Proceed-
ings of the Eighteenth International Conference on
Machine Learning, ICML ’01, pages 282–289, San
Francisco, CA, USA. Morgan Kaufmann Publishers
Inc.
Le, H., Vial, L., Frej, J., Segonne, V., Coavoux, M., Lecou-
teux, B., Allauzen, A., Crabb
´
e, B., Besacier, L., and
Schwab, D. (2019). Flaubert: Unsupervised lan-
guage model pre-training for french. arXiv preprint
arXiv:1912.05372.
Lee, J., Yoon, W., Kim, S., Kim, D., Kim, S., So, C. H.,
and Kang, J. (2019). Biobert: a pre-trained biomedi-
cal language representation model for biomedical text
mining. Bioinformatics.
Levine, J. M. (2003). De-identification of ICU patient
records. PhD thesis, Massachusetts Institute of Tech-
nology.
Liu, Z., Tang, B., Wang, X., and Chen, Q. (2017). De-
identification of clinical notes via recurrent neural
network and conditional random field. Journal of
Biomedical Informatics, 75.
Loshchilov, I. and Hutter, F. (2017). Decoupled weight de-
cay regularization. arXiv preprint arXiv:1711.05101.
Nothman, J., Ringland, N., Radford, W., Murphy, T.,
and Curran, J. R. (2013). Learning multilingual
named entity recognition from wikipedia. Artificial
Intelligence, 194:151–175. Artificial Intelligence,
Wikipedia and Semi-Structured Resources.
Polignano, M., de Gemmis, M., and Semeraro, G. (2021).
Comparing transformer-based NER approaches for
analysing textual medical diagnoses. In Faggioli,
G., Ferro, N., Joly, A., Maistro, M., and Piroi, F.,
editors, Proceedings of the Working Notes of CLEF
2021 - Conference and Labs of the Evaluation Forum,
Bucharest, Romania, September 21st - to - 24th, 2021,
volume 2936 of CEUR Workshop Proceedings, pages
818–833. CEUR-WS.org.
Sch
¨
afer, H., Idrissi-Yaghir, A., Horn, P., and Friedrich, C.
(2022). Cross-language transfer of high-quality anno-
tations: Combining neural machine translation with
cross-linguistic span alignment to apply NER to clini-
cal texts in a low-resource language. In Proceedings of
the 4th Clinical Natural Language Processing Work-
shop, pages 53–62, Seattle, WA. Association for Com-
putational Linguistics.
Stubbs, A., Kotfila, C., and Uzuner,
¨
O. (2015). Auto-
mated systems for the de-identification of longitudinal
clinical narratives: Overview of 2014 i2b2/uthealth
shared task track 1. Journal of biomedical informatics,
58:S11–S19.
Sun, W., Rumshisky, A., and Uzuner, O. (2013). Evaluat-
ing temporal relations in clinical text: 2012 i2b2 chal-
lenge. Journal of the American Medical Informatics
Association, 20(5):806–813.
Sweeney, L. (1996). Replacing personally-identifying in-
formation in medical records, the scrub system. In
Proceedings of the AMIA annual fall symposium, page
333. American Medical Informatics Association.
Tchouka, Y., Couchot, J., Coulmeau, M., Laiymani, D.,
Selles, P., Rahmani, A., and Guyeux, C. (2022). De-
identification of french unstructured clinical notes for
machine learning tasks. CoRR, abs/2209.09631.
Uzuner,
¨
O., Luo, Y., and Szolovits, P. (2007). Evaluating the
state-of-the-art in automatic de-identification. Jour-
nal of the American Medical Informatics Association,
14(5):550–563.
Vaswani, A., Shazeer, N., Parmar, N., Uszkoreit, J., Jones,
L., Gomez, A. N., Kaiser, Ł., and Polosukhin, I.
(2017). Attention is all you need. Advances in neural
information processing systems, 30.
Velupillai, S., Suominen, H., Liakata, M., Roberts, A.,
Shah, A. D., Morley, K., Osborn, D., Hayes, J., Stew-
art, R., Downs, J., et al. (2018). Using clinical natu-
ral language processing for health outcomes research:
overview and actionable suggestions for future ad-
vances. Journal of biomedical informatics, 88:11–19.
Xiao, Y. and Xiong, L. (2015). Protecting locations with
differential privacy under temporal correlations. In
Proceedings of the 22nd ACM SIGSAC Conference
on Computer and Communications Security, pages
1298–1309.
Zhao, Y. and Chen, J. (2022). A survey on differential pri-
vacy for unstructured data content. ACM Computing
Surveys (CSUR).
HEALTHINF 2023 - 16th International Conference on Health Informatics
104