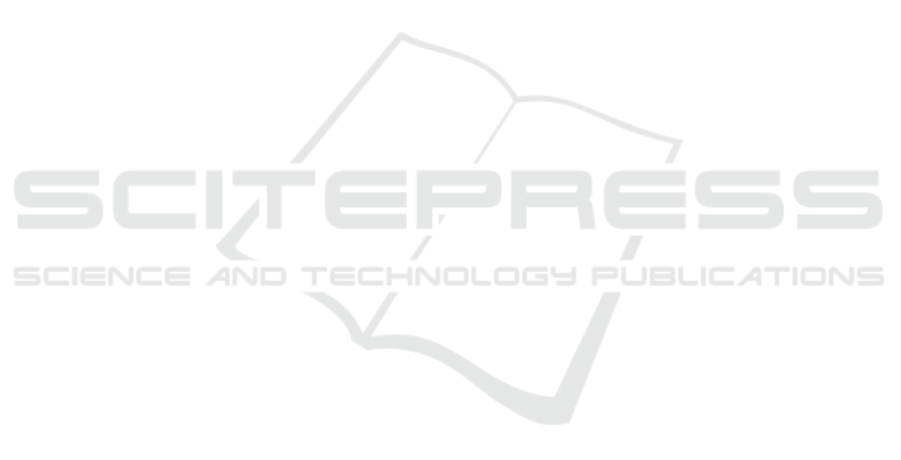
trol an EWH in order to maximize comfort and to
minimize energy cost. Results showed that the for-
mulation of DHW production as a multi-objective
sequential decision problem allows to have multiple
policies that can suit each user in terms of prefer-
ences. The proposed approach can save energy cost
up to 10.24 % in a cautious control case without any
real impact on comfort. It turns out that a trained
agent with the most conservative policy for comfort
can have better results in terms of comfort and cost re-
duction than decreasing the rule-based control by 10
◦
C compared to the baseline. In future work, these re-
sults can be compared to a multi-objective optimiza-
tion with known DHW consumption needs. Thus, the
Pareto front can be estimated and this will allow to
check the optimality of the obtained policies.
The presented method can also be used to find
trade-offs between energy consumption reduction and
comfort for multiple applications. This can be useful
during the current energy crisis in Europe and allows
energy consumption to be reduced without impacting
comfort and habits of users.
Some limitations of the proposed method are
known. The method requires a prior knowledge of
preferences over different objectives and the expres-
sion of preferences can be limited to linear scalariza-
tion. In addition, the architecture of the NN can be
improved to solve problems with more objectives.
ACKNOWLEDGEMENTS
The authors would thank the partners of the COREN-
STOCK Industrial Research Chair, as a national ANR
project for providing the context of this work.
REFERENCES
Amasyali, K., Munk, J., Kurte, K., Kuruganti, T., and
Zandi, H. (2021). Deep reinforcement learning for au-
tonomous water heater control. Buildings, 11(11):548.
Booysen, M., Engelbrecht, J., Ritchie, M., Apperley, M.,
and Cloete, A. (2019). How much energy can optimal
control of domestic water heating save? Energy for
Sustainable Development, 51:73–85.
Heidari, A., Mar
´
echal, F., and Khovalyg, D. (2022).
An occupant-centric control framework for balancing
comfort, energy use and hygiene in hot water systems:
A model-free reinforcement learning approach. Ap-
plied Energy, 312:118833.
Heidari, A., Olsen, N., Mermod, P., Alahi, A., and Khova-
lyg, D. (2021). Adaptive hot water production based
on supervised learning. Sustainable Cities and Soci-
ety, 66:102625.
Hendron, B., Burch, J., and Barker, G. (2010). Tool for
generating realistic residential hot water event sched-
ules. Technical report, National Renewable Energy
Lab.(NREL), Golden, CO (United States).
Hwang, C.-L. and Masud, A. S. M. (2012). Multiple ob-
jective decision making—methods and applications: a
state-of-the-art survey, volume 164. Springer Science
& Business Media.
Issabekov, R. and Vamplew, P. (2012). An empirical com-
parison of two common multiobjective reinforcement
learning algorithms. In Australasian Joint Conference
on Artificial Intelligence, pages 626–636. Springer.
Kapsalis, V., Safouri, G., and Hadellis, L. (2018).
Cost/comfort-oriented optimization algorithm for op-
eration scheduling of electric water heaters under
dynamic pricing. Journal of cleaner production,
198:1053–1065.
Kazmi, H., Mehmood, F., Lodeweyckx, S., and Driesen,
J. (2018). Gigawatt-hour scale savings on a budget
of zero: Deep reinforcement learning based optimal
control of hot water systems. Energy, 144:159–168.
Liu, C., Xu, X., and Hu, D. (2014). Multiobjective rein-
forcement learning: A comprehensive overview. IEEE
Transactions on Systems, Man, and Cybernetics: Sys-
tems, 45(3):385–398.
Mnih, V., Kavukcuoglu, K., Silver, D., Rusu, A. A., Ve-
ness, J., Bellemare, M. G., Graves, A., Riedmiller, M.,
Fidjeland, A. K., Ostrovski, G., et al. (2015). Human-
level control through deep reinforcement learning. na-
ture, 518(7540):529–533.
Patyn, C., Peirelinck, T., Deconinck, G., and Nowe, A.
(2018). Intelligent electric water heater control with
varying state information. In 2018 IEEE Interna-
tional Conference on Communications, Control, and
Computing Technologies for Smart Grids (SmartGrid-
Comm), pages 1–6. IEEE.
Roijers, D. M., Vamplew, P., Whiteson, S., and Dazeley,
R. (2013). A survey of multi-objective sequential
decision-making. Journal of Artificial Intelligence Re-
search, 48:67–113.
Ruelens, F., Claessens, B. J., Quaiyum, S., De Schutter, B.,
Babu
ˇ
ska, R., and Belmans, R. (2016). Reinforcement
learning applied to an electric water heater: From the-
ory to practice. IEEE Transactions on Smart Grid,
9(4):3792–3800.
Shen, G., Lee, Z. E., Amadeh, A., and Zhang, K. M. (2021).
A data-driven electric water heater scheduling and
control system. Energy and Buildings, 242:110924.
Tesauro, G., Das, R., Chan, H., Kephart, J., Levine, D.,
Rawson, F., and Lefurgy, C. (2007). Managing power
consumption and performance of computing systems
using reinforcement learning. Advances in neural in-
formation processing systems, 20.
Vamplew, P., Dazeley, R., Berry, A., Issabekov, R., and
Dekker, E. (2011). Empirical evaluation methods
for multiobjective reinforcement learning algorithms.
Machine learning, 84(1):51–80.
Van Moffaert, K. (2016). Multi-criteria reinforcement
learning for sequential decision making problems.
PhD thesis, Ph. D. thesis, Vrije Universiteit Brussel.
Multi-Objective Deep Q-Networks for Domestic Hot Water Systems Control
241