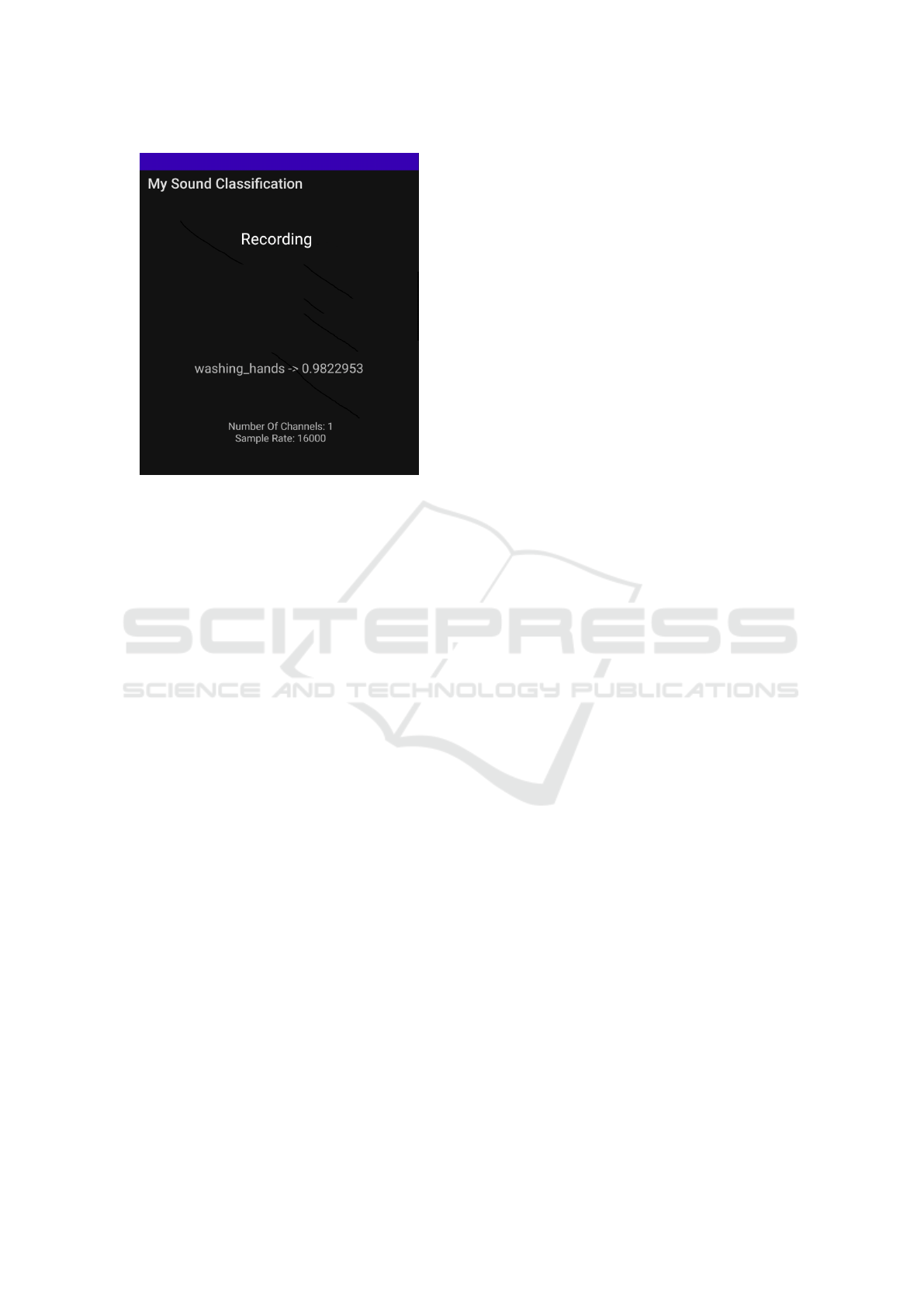
Figure 7: Screenshot of the developed prototype application
running on Android mobile operating system. The winning
class along and the associated probability is displayed to the
user.
to classify human activities using a reasonably-sized
dataset. The obtained results demonstrate the supe-
riority of the proposed system over the state-of-art
based on supervised feature learning. Weaknesses of
the model could emerge in case of scaling the num-
ber of classes with proportional number of instances:
indeed, the model would need more data to learn a
more complex problem for which the current neural
architecture may not be enough accurate.
Future works include the use of artificial data aug-
mentation to enlarge the dataset. Possibly YAMNet
hyper-parameters could be fine-tuned if the dataset
is sufficiently large. Moreover, the effectiveness of
the smartphone application should be assessed thor-
oughly in terms of complexity along with the required
resources. Finally, the developed application could be
employed to enhance the capabilities of a wide range
of systems including smart-home assistants, such as
Amazon Alexa, Google Home, etc.
REFERENCES
Beddiar, D. R., Nini, B., Sabokrou, M., and Hadid,
A. (2020). Vision-based human activity recogni-
tion: A survey. Multimedia Tools and Applications,
79(41):30509–30555.
Bevilacqua, A., MacDonald, K., Rangarej, A., Widjaya, V.,
Caulfield, B., and Kechadi, T. (2018). Human activ-
ity recognition with convolutional neural networks. In
Joint European Conference on Machine Learning and
Knowledge Discovery in Databases, pages 541–552.
Springer.
Chahuara, P., Fleury, A., Portet, F., and Vacher, M. (2016).
On-line human activity recognition from audio and
home automation sensors: Comparison of sequential
and non-sequential models in realistic smart homes 1.
Journal of Ambient Intelligence and Smart Environ-
ments, 8(4):399–422.
Chen, K., Zhang, D., Yao, L., Guo, B., Yu, Z., and Liu, Y.
(2021). Deep learning for sensor-based human activ-
ity recognition: Overview, challenges, and opportuni-
ties. ACM Computing Surveys, 54(4):77:1–77:40.
Garc
´
ıa-Hern
´
andez, A., Galv
´
an-Tejada, C., Galv
´
an-Tejada,
J., Celaya-Padilla, J., Gamboa-Rosales, H., Velasco-
Elizondo, P., and C
´
ardenas-Vargas, R. (2017). A sim-
ilarity analysis of audio signal to develop a human ac-
tivity recognition using similarity networks. Sensors,
17(11):2688.
Giannakopoulos, T. and Pikrakis, A. (2014). Introduction
to Audio Analysis: A MATLAB Approach. Academic
Press, Inc., USA, 1st edition.
Hota, S. and Pathak, S. (2018). KNN classifier based ap-
proach for multi-class sentiment analysis of twitter
data. International Journal of Engineering and Tech-
nology, 7(3):1372.
Howard, A. G., Zhu, M., Chen, B., Kalenichenko, D.,
Wang, W., Weyand, T., Andreetto, M., and Adam,
H. (2017). Mobilenets: Efficient convolutional neu-
ral networks for mobile vision applications. CoRR,
abs/1704.04861.
Kahl, S., Wilhelm-Stein, T., Hussein, H., Klinck, H., Kow-
erko, D., Ritter, M., and Eibl, M. (2017). Large-scale
bird sound classification using convolutional neural
networks. In CLEF (working notes), volume 1866.
Kingma, D. P. and Ba, J. (2014). Adam: A method for
stochastic optimization.
Lau, J., Zimmerman, B., and Schaub, F. (2018). Alexa, are
you listening? Proceedings of the ACM on Human-
Computer Interaction, 2(CSCW):1–31.
Liang, D., Song, W., and Thomaz, E. (2020). Characteriz-
ing the effect of audio degradation on privacy percep-
tion and inference performance in audio-based human
activity recognition. In 22nd International Conference
on Human-Computer Interaction with Mobile Devices
and Services, MobileHCI ’20, pages 1–10, New York,
NY, USA. Association for Computing Machinery.
Ntalampiras, S. and Potamitis, I. (2018). Transfer learning
for improved audio-based human activity recognition.
Biosensors, 8(3):60.
Ntalampiras, S., Potamitis, I., and Fakotakis, N. (2012).
Acoustic detection of human activities in natural en-
vironments. AES: Journal of the Audio Engineering
Society, 60(9):686–695.
Ntalampiras, S. and Roveri, M. (2016). An incremental
learning mechanism for human activity recognition.
In 2016 IEEE Symposium Series on Computational
Intelligence (SSCI), pages 1–6.
Pan, S. J. and Yang, Q. (2010). A survey on transfer learn-
ing. IEEE Transactions on Knowledge and Data En-
gineering, 22(10):1345–1359.
ICPRAM 2023 - 12th International Conference on Pattern Recognition Applications and Methods
788