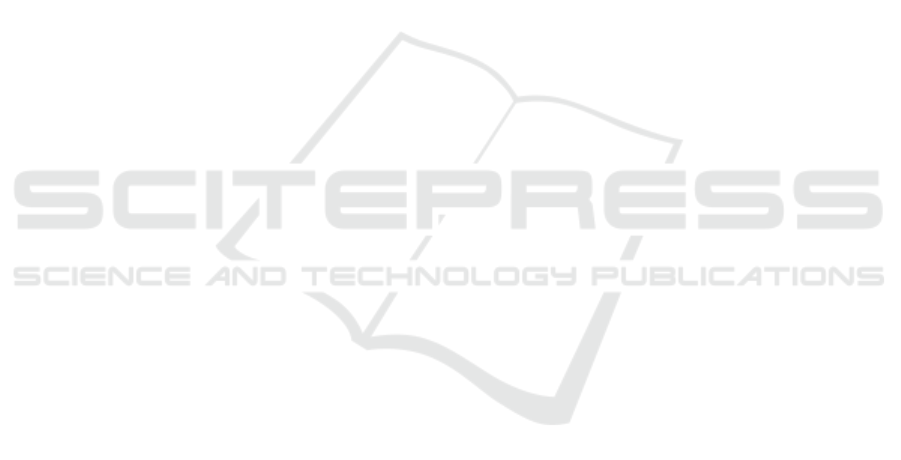
for the assessment. A guideline for the appropri-
ateness can be Bloom’s taxonomy.
With this work, we want to encourage researchers
and developers working in the field of AI-supported
assessment to give more consideration to the per-
spective of students. The development and imple-
mentation of tools that affect students and their as-
sessment should always be critically accompanied by
them. The findings presented in this paper can be a
first guideline on how to design systems in a student-
friendly way.
REFERENCES
Baker, T., Smith, L., and Anissa, N. (2019). Educ-ai-tion re-
booted? exploring the future of artificial intelligence
in schools and colleges. Technical report, nesta foun-
dation.
Choi, J. H., Hickman, K. E., Monahan, A., and Schwarcz,
D. (2023). Chatgpt goes to law school. SSRN.
Davis, F. D. (1989). Perceived usefulness, perceived ease of
use, and user acceptance of information technology.
MIS Quarterly, 13(3):319–340.
Forehand, M. (2010). Bloom’s taxonomy. Emerging
perspectives on learning, teaching, and technology,
41(4):47–56.
Funk, S. C. and Dickson, K. L. (2011). Multiple-choice and
short-answer exam performance in a college class-
room. Teaching of Psychology, 38(4):273–277.
Galassi, A. and Vittorini, P. (2021). Automated feedback
to students in data science assignments: Improved im-
plementation and results. In CHItaly 2021: 14th Bian-
nual Conference of the Italian SIGCHI Chapter, CHI-
taly ’21, New York, NY, USA. Association for Com-
puting Machinery.
Gibbs, G. (2006). Why assessment is changing. In Inno-
vative assessment in higher education, pages 31–42.
Routledge.
Gilson, A., Safranek, C. W., Huang, T., Socrates, V., Chi,
L., Taylor, R. A., and Chartash, D. (2023). How does
chatgpt perform on the united states medical licensing
examination? the implications of large language mod-
els for medical education and knowledge assessment.
JMIR Med Educ, 9:e45312.
Medland, E. (2016). Assessment in higher education:
drivers, barriers and directions for change in the
uk. Assessment & Evaluation in Higher Education,
41(1):81–96.
Mehrabi, N., Morstatter, F., Saxena, N., Lerman, K., and
Galstyan, A. (2021). A survey on bias and fairness in
machine learning. ACM Computing Surveys (CSUR),
54(6):1–35.
Mirmotahari, O., Berg, Y., Gjessing, S., Fremstad, E., and
Damsa, C. (2019). A case-study of automated feed-
back assessment. In 2019 IEEE Global Engineer-
ing Education Conference (EDUCON), pages 1190–
1197.
Paxton, M. (2000). A linguistic perspective on multi-
ple choice questioning. Assessment & Evaluation in
Higher Education, 25(2):109–119.
Roberts, T. S. (2006). The use of multiple choice tests
for formative and summative assessment. In Proceed-
ings of the 8th Australasian Conference on Computing
Education-Volume 52, pages 175–180.
S
´
anchez-Prieto, J. C., Cruz-Benito, J., Ther
´
on S
´
anchez, R.,
Garc
´
ıa Pe
˜
nalvo, F. J., et al. (2020). Assessed by ma-
chines: development of a tam-based tool to measure
ai-based assessment acceptance among students. In-
ternational Journal of Interactive Multimedia and Ar-
tificial Intelligence, 6(4):80.
Scharber, C., Dexter, S., and Riedel, E. (2008). Students’
experiences with an automated essay scorer. Journal
of Technology, Learning, and Assessment, 7(1).
Tan, S. H. S., Thibault, G., Chew, A. C. Y., and Rajalingam,
P. (2022). Enabling open-ended questions in team-
based learning using automated marking: Impact on
student achievement, learning and engagement. Jour-
nal of Computer Assisted Learning, page 1–13.
United Nations (2016). Transforming our world: The 2030
agenda for sustainable development.
Zawacki-Richter, O., Mar
´
ın, V. I., Bond, M., and Gou-
verneur, F. (2019). Systematic review of re-
search on artificial intelligence applications in higher
education–where are the educators? International
Journal of Educational Technology in Higher Educa-
tion, 16(1):1–27.
APPENDIX
This appendix contains the questions that were used
for the survey of students with teaching experience
and as a guideline for the semi-structured interviews
(Appendix 6.1), as well as the questions of the second
survey among the general student population (Ap-
pendix 6.2).
Survey I
• What is your study?
• How important do you think human input is for
grading open-ended questions?
– Why do you think that is important?
– Can you name a few benefits of having a
teacher/student grading an open-ended ques-
tion instead of a machine/tool?
– Are there any issues that can arise when assess-
ing open-ended questions?
• Do you believe that automation can help with the
assessment of open-ended questions? (Why/Why
not?)
CSEDU 2023 - 15th International Conference on Computer Supported Education
78