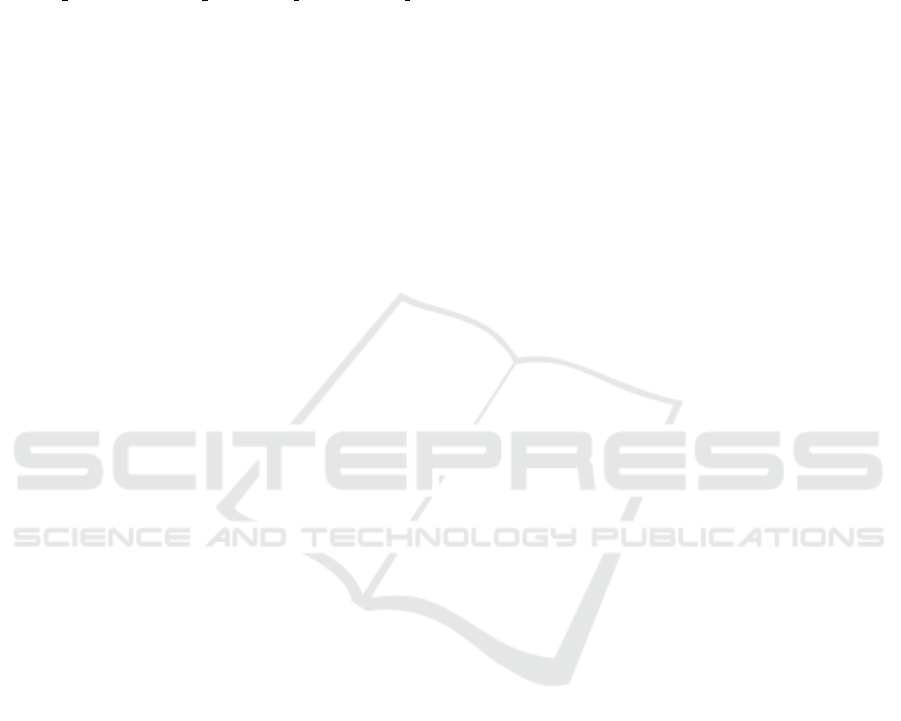
Jord
˜
ao, A. and Pedrini, H. (2021). On the effect of prun-
ing on adversarial robustness. In Proceedings of the
IEEE/CVF International Conference on Computer Vi-
sion (ICCV) Workshops, pages 1–11.
KIA Project Booklet (2022). KI Absicherung
Overview and Poster Abstracts. https:
//www.ki-absicherung-projekt.de/fileadmin/
KI Absicherung/Final Event/KI-A poster-booklet
Onlineversion.pdf.
Kwon, S. J., Lee, D., Kim, B., Kapoor, P., Park, B., and
Wei, G.-Y. (2020). Structured compression by weight
encryption for unstructured pruning and quantization.
In Proceedings of the IEEE/CVF Conference on Com-
puter Vision and Pattern Recognition, pages 1909–
1918.
LeCun, Y., Denker, J., and Solla, S. (1989). Optimal brain
damage. Advances in neural information processing
systems, 2.
Lee, N., Ajanthan, T., and Torr, P. H. (2018). Snip: Single-
shot network pruning based on connection sensitivity.
arXiv preprint arXiv:1810.02340.
Li, H., Kadav, A., Durdanovic, I., Samet, H., and Graf, H. P.
(2016). Pruning filters for efficient convnets. arXiv
preprint arXiv:1608.08710.
Li, Z., Chen, T., Li, L., Li, B., and Wang, Z. (2022). Can
pruning improve certified robustness of neural net-
works? arXiv preprint arXiv:2206.07311.
Lin, M., Ji, R., Wang, Y., Zhang, Y., Zhang, B., Tian, Y., and
Shao, L. (2020). Hrank: Filter pruning using high-
rank feature map. In Proceedings of the IEEE/CVF
conference on computer vision and pattern recogni-
tion, pages 1529–1538.
Liu, W., Anguelov, D., Erhan, D., Szegedy, C., Reed, S.,
Fu, C.-Y., and Berg, A. C. (2016). Ssd: Single shot
multibox detector. In European conference on com-
puter vision, pages 21–37. Springer.
Montavon, G., Lapuschkin, S., Binder, A., Samek, W., and
M
¨
uller, K.-R. (2017a). Explaining nonlinear classifi-
cation decisions with deep taylor decomposition. Pat-
tern Recognition, 65:211 – 222.
Montavon, G., Lapuschkin, S., Binder, A., Samek, W., and
M
¨
uller, K.-R. (2017b). Explaining nonlinear classifi-
cation decisions with deep taylor decomposition. Pat-
tern recognition, 65:211–222.
Nadizar, G., Medvet, E., Pellegrino, F. A., Zullich, M.,
and Nichele, S. (2021). On the effects of pruning
on evolved neural controllers for soft robots. In Pro-
ceedings of the Genetic and Evolutionary Computa-
tion Conference Companion, pages 1744–1752.
Otter, D. W., Medina, J. R., and Kalita, J. K. (2020). A
survey of the usages of deep learning for natural lan-
guage processing. IEEE transactions on neural net-
works and learning systems, 32(2):604–624.
Samek, W., Montavon, G., Lapuschkin, S., Anders, C. J.,
and M
¨
uller, K.-R. (2021). Explaining deep neural net-
works and beyond: A review of methods and applica-
tions. Proceedings of the IEEE, 109(3):247–278.
Sehwag, V., Wang, S., Mittal, P., and Jana, S. (2020). Hy-
dra: Pruning adversarially robust neural networks. In
Larochelle, H., Ranzato, M., Hadsell, R., Balcan, M.,
and Lin, H., editors, Advances in Neural Information
Processing Systems, volume 33, pages 19655–19666.
Curran Associates, Inc.
Simonyan, K. and Zisserman, A. (2014). Very deep con-
volutional networks for large-scale image recognition.
arXiv preprint arXiv:1409.1556.
Wang, X., Yu, F., Dou, Z.-Y., and Gonzalez, J. E. (2017).
Skipnet: Learning dynamic routing in convolutional
networks. CoRR, abs/1711.09485.
Weichert, D., Link, P., Stoll, A., R
¨
uping, S., Ihlenfeldt, S.,
and Wrobel, S. (2019). A review of machine learning
for the optimization of production processes. The In-
ternational Journal of Advanced Manufacturing Tech-
nology, 104(5):1889–1902.
Ye, S., Xu, K., Liu, S., Cheng, H., Lambrechts, J., Zhang,
H., Zhou, A., Ma, K., Wang, Y., and Lin, X. (2019).
Adversarial robustness vs. model compression, or
both? In 2019 IEEE/CVF International Confer-
ence on Computer Vision (ICCV), pages 111–120, Los
Alamitos, CA, USA. IEEE Computer Society.
Zeng, W. and Urtasun, R. (2019). Mlprune: Multi-layer
pruning for automated neural network compression.
ICPRAM 2023 - 12th International Conference on Pattern Recognition Applications and Methods
88