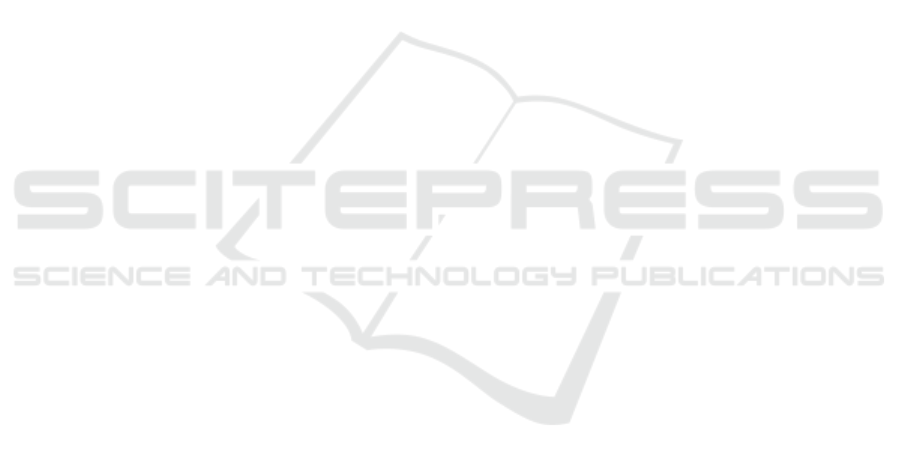
REFERENCES
Antol, S., Agrawal, A., Lu, J., Mitchell, M., Batra, D.,
Zitnick, C. L., and Parikh, D. (2015). Vqa: Visual
question answering. In Proceedings of the IEEE inter-
national conference on computer vision, pages 2425–
2433.
Banerjee, S. and Lavie, A. (2005). Meteor: An automatic
metric for mt evaluation with improved correlation
with human judgments. In Proceedings of the acl
workshop on intrinsic and extrinsic evaluation mea-
sures for machine translation and/or summarization,
pages 65–72.
Cho, K., Van Merri
¨
enboer, B., Gulcehre, C., Bahdanau, D.,
Bougares, F., Schwenk, H., and Bengio, Y. (2014).
Learning phrase representations using rnn encoder-
decoder for statistical machine translation. arXiv
preprint arXiv:1406.1078.
Dosovitskiy, A., Beyer, L., Kolesnikov, A., Weissenborn,
D., Zhai, X., Unterthiner, T., Dehghani, M., Minderer,
M., Heigold, G., Gelly, S., et al. (2020). An image is
worth 16x16 words: Transformers for image recogni-
tion at scale. arXiv preprint arXiv:2010.11929.
He, K., Zhang, X., Ren, S., and Sun, J. (2016a). Deep resid-
ual learning for image recognition. In Proceedings of
the IEEE conference on computer vision and pattern
recognition, pages 770–778.
He, K., Zhang, X., Ren, S., and Sun, J. (2016b). Deep resid-
ual learning for image recognition. In Proceedings of
the IEEE conference on computer vision and pattern
recognition, pages 770–778.
Hochreiter, S. and Schmidhuber, J. (1997). Long short-term
memory. Neural computation, 9(8):1735–1780.
Kafle, K. and Kanan, C. (2016). Answer-type prediction for
visual question answering. In Proceedings of the IEEE
conference on computer vision and pattern recogni-
tion, pages 4976–4984.
Kim, J.-H., Lee, S.-W., Kwak, D., Heo, M.-O., Kim, J., Ha,
J.-W., and Zhang, B.-T. (2016). Multimodal residual
learning for visual qa. Advances in neural information
processing systems, 29.
Kiros, R., Zhu, Y., Salakhutdinov, R. R., Zemel, R., Ur-
tasun, R., Torralba, A., and Fidler, S. (2015). Skip-
thought vectors. Advances in neural information pro-
cessing systems, 28.
Krishna, R., Zhu, Y., Groth, O., Johnson, J., Hata,
K., Kravitz, J., Chen, S., Kalantidis, Y., Li, L.-
J., Shamma, D. A., et al. (2017). Visual genome:
Connecting language and vision using crowdsourced
dense image annotations. International journal of
computer vision, 123(1):32–73.
Lin, C.-Y. (2004). Rouge: A package for automatic evalu-
ation of summaries. In Text summarization branches
out, pages 74–81.
Lin, T.-Y., Maire, M., Belongie, S., Hays, J., Perona, P.,
Ramanan, D., Doll
´
ar, P., and Zitnick, C. L. (2014).
Microsoft coco: Common objects in context. In Euro-
pean conference on computer vision, pages 740–755.
Springer.
Lu, J., Yang, J., Batra, D., and Parikh, D. (2016). Hierar-
chical question-image co-attention for visual question
answering. Advances in neural information process-
ing systems, 29.
Malinowski, M. and Fritz, M. (2014). A multi-world ap-
proach to question answering about real-world scenes
based on uncertain input. Advances in neural infor-
mation processing systems, 27.
Malinowski, M., Rohrbach, M., and Fritz, M. (2015). Ask
your neurons: A neural-based approach to answering
questions about images. In Proceedings of the IEEE
international conference on computer vision, pages 1–
9.
Papineni, K., Roukos, S., Ward, T., and Zhu, W.-J. (2002).
Bleu: a method for automatic evaluation of machine
translation. In Proceedings of the 40th annual meet-
ing of the Association for Computational Linguistics,
pages 311–318.
Saito, K., Shin, A., Ushiku, Y., and Harada, T. (2017). Du-
alnet: Domain-invariant network for visual question
answering. In 2017 IEEE International Conference on
Multimedia and Expo (ICME), pages 829–834. IEEE.
Simonyan, K. and Zisserman, A. (2014). Very deep con-
volutional networks for large-scale image recognition.
arXiv preprint arXiv:1409.1556.
Thomee, B., Shamma, D. A., Friedland, G., Elizalde, B.,
Ni, K., Poland, D., Borth, D., and Li, L.-J. (2016).
Yfcc100m: The new data in multimedia research.
Communications of the ACM, 59(2):64–73.
Wu, Z. (1994). Palmer, m.: Verbs semantics and lexical
selection. In Proceedings of the 32nd Annual Meet-
ing of Association for Computational Linguistics, Las
Cruces, New Mexico.
Yang, Z., He, X., Gao, J., Deng, L., and Smola, A. (2016).
Stacked attention networks for image question an-
swering. In Proceedings of the IEEE conference on
computer vision and pattern recognition, pages 21–
29.
Zhou, B., Tian, Y., Sukhbaatar, S., Szlam, A., and Fergus,
R. (2015). Simple baseline for visual question answer-
ing. arXiv preprint arXiv:1512.02167.
ICPRAM 2023 - 12th International Conference on Pattern Recognition Applications and Methods
288