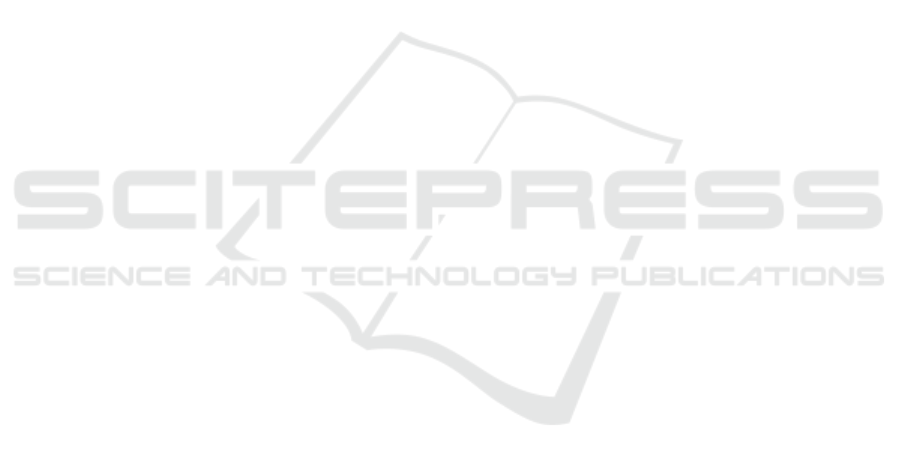
Gay, A.-S., Humbert, S., Taleb, A.-L., Lefebvre, V.,
Dalverny, C., Desjouis, G., and Bizien, T. (2016).
Combined characterization of cobalt aggregates by
haadf-stem electron tomography and anomalous x-
ray scattering. European Microscopy Congress 2016:
Proceedings, pages 39–40.
Genc, A., Kovarik, L., and Fraser, H. L. (2022). A deep
learning approach for semantic segmentation of un-
balanced data in electron tomography of catalytic ma-
terials. arXiv preprint arXiv:2201.07342.
Grill, J.-B., Strub, F., Altch
´
e, F., Tallec, C., Richemond,
P. H., Buchatskaya, E., Doersch, C., Pires, B. A., Guo,
Z. D., Azar, M. G., Piot, B., Kavukcuoglu, K., Munos,
R., and Valko, M. (2020). Bootstrap Your Own Latent:
A new approach to self-supervised learning. Neural
Information Processing Systems.
Hadsell, R., Chopra, S., and LeCun, Y. (2006). Dimen-
sionality reduction by learning an invariant mapping.
2006 IEEE Conference on Computer Vision and Pat-
tern Recognition (CVPR’06), 2:1735–1742.
He, W., Ladinsky, M. S., Huey-Tubman, K. E., Jensen,
G. J., McIntosh, J. R., and Bj
¨
orkman, P. J. (2008).
Fcrn-mediated antibody transport across epithelial
cells revealed by electron tomography. nature,
455(7212):542–546.
Kern, D., Klauck, U., Ropinski, T., and Mastmeyer, A.
(2021). 2D vs. 3D U-Net abdominal organ segmenta-
tion in CT data using organ bounds. Medical Imaging
2021: Imaging Informatics for Healthcare, Research,
and Applications, 11601:192 – 200.
Khadangi, A., Boudier, T., and Rajagopal, V. (2021). Em-
net: Deep learning for electron microscopy image seg-
mentation. 2020 25th International Conference on
Pattern Recognition (ICPR), pages 31–38.
Khosla, P., Teterwak, P., Wang, C., Sarna, A., Tian, Y.,
Isola, P., Maschinot, A., Liu, C., and Krishnan, D.
(2020). Supervised contrastive learning. Advances
in Neural Information Processing Systems, 33:18661–
18673.
Koch, G., Zemel, R., and Salakhutdinov, R. (2015).
Siamese neural networks for one-shot image recogni-
tion. ICML deep learning workshop, 2.
Li, X., Yu, L., Chen, H., Fu, C., and Heng, P. (2018). Semi-
supervised skin lesion segmentation via transforma-
tion consistent self-ensembling model. British Ma-
chine Vision Conference 2018, BMVC 2018, Newcas-
tle, UK, September 3-6, 2018, page 63.
Medeiros-Costa, I. C., Laroche, C., P
´
erez-Pellitero, J., and
Coasne, B. (2019). Characterization of hierarchi-
cal zeolites: Combining adsorption/intrusion, electron
microscopy, diffraction and spectroscopic techniques.
Microporous and Mesoporous Materials, 287:167–
176.
Milletari, F., Navab, N., and Ahmadi, S.-A. (2016). V-
net: Fully convolutional neural networks for volumet-
ric medical image segmentation. 2016 fourth interna-
tional conference on 3D vision (3DV), pages 565–571.
Ouali, Y., Hudelot, C., and Tami, M. (2020). Semi-
supervised semantic segmentation with cross-
consistency training. Proceedings of the IEEE/CVF
Conference on Computer Vision and Pattern Recogni-
tion, pages 12674–12684.
Pan, S. J. and Yang, Q. (2009). A survey on transfer learn-
ing. IEEE Transactions on knowledge and data engi-
neering, 22(10):1345–1359.
Peng, J., Estrada, G., Pedersoli, M., and Desrosiers, C.
(2020). Deep co-training for semi-supervised image
segmentation. Pattern Recognition, 107:107269.
Ronneberger, O., Fischer, P., and Brox, T. (2015). U-net:
Convolutional networks for biomedical image seg-
mentation. Medical Image Computing and Computer-
Assisted Intervention – MICCAI 2015, pages 234–
241.
Sun, K., Xiao, B., Liu, D., and Wang, J. (2019). Deep high-
resolution representation learning for human pose es-
timation. Proceedings of the IEEE/CVF Conference
on Computer Vision and Pattern Recognition, pages
5693–5703.
Volkmann, N. (2010). Methods for segmentation and in-
terpretation of electron tomographic reconstructions.
Methods in enzymology, 483:31–46.
Wurm, M., Stark, T., Zhu, X. X., Weigand, M., and
Taubenb
¨
ock, H. (2019). Semantic segmentation of
slums in satellite images using transfer learning on
fully convolutional neural networks. ISPRS Journal
of Photogrammetry and Remote Sensing, 150:59–69.
Zhao, X., Vemulapalli, R., Mansfield, P. A., Gong, B.,
Green, B., Shapira, L., and Wu, Y. (2021). Con-
trastive learning for label efficient semantic segmen-
tation. Proceedings of the IEEE/CVF International
Conference on Computer Vision, pages 10623–10633.
Zhou, Z., Rahman Siddiquee, M. M., Tajbakhsh, N., and
Liang, J. (2018). Unet++: A nested u-net architecture
for medical image segmentation. In Deep learning in
medical image analysis and multimodal learning for
clinical decision support, pages 3–11. Springer.
Toward Few Pixel Annotations for 3D Segmentation of Material from Electron Tomography
131