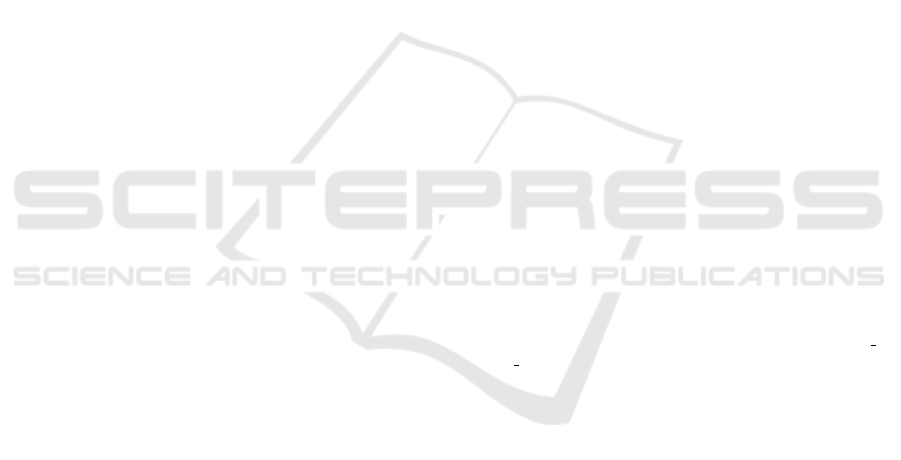
ACKNOWLEDGMENT
This paper was achieved in cooperation with HP
Inc. R&D Brazil, using incentives of the Brazilian
Informatics Law (n°. 8.2.48 of 1991). The authors
would like to thank Thomas da Silva Paula for being
the project conceiver and Eduardo Chagas for the
management support during this work.
REFERENCES
Antunes, R. S., Andr
´
e da Costa, C., K
¨
uderle, A., Yari,
I. A., and Eskofier, B. (2022). Federated learning
for healthcare: Systematic review and architecture
proposal. ACM Transactions on Intelligent Systems
and Technology (TIST).
Beutel, D. J., Topal, T., Mathur, A., Qiu, X., Parcollet, T.,
de Gusm
˜
ao, P. P., and Lane, N. D. (2020). Flower: A
friendly federated learning research framework. arXiv
preprint arXiv:2007.14390.
Bulatovich, B. K., Vladimirovna, E. E., Vyacheslavovich,
T. D., Sergeevna, S. N., Sergeevna, C. Y., Zuheng, M.,
Jean-Christophe, B., and Muzzamil, L. M. (2022a).
Midv-2020: A comprehensive benchmark dataset
for identity document analysis. Computer Optics,
46(2):252–270.
Bulatovich, B. K., Vladimirovna, E. E., Vyacheslavovich,
T. D., Sergeevna, S. N., Sergeevna, C. Y., Zuheng, M.,
Jean-Christophe, B., and Muzzamil, L. M. (2022b).
Midv-2020: A comprehensive benchmark dataset for
identity document analysis. 46(2):252–270.
Burie, J.-C., Chazalon, J., Coustaty, M., Eskenazi, S.,
Luqman, M. M., Mehri, M., Nayef, N., Ogier,
J.-M., Prum, S., and Rusi
˜
nol, M. (2015). Icdar2015
competition on smartphone document capture and ocr
(smartdoc). In 2015 13th International Conference on
Document Analysis and Recognition (ICDAR), pages
1161–1165. IEEE.
Caldas, S., Duddu, S. M. K., Wu, P., Li, T., Kone
ˇ
cn
`
y, J.,
McMahan, H. B., Smith, V., and Talwalkar, A. (2018).
Leaf: A benchmark for federated settings. arXiv
preprint arXiv:1812.01097.
Chernyshova, Y., Emelianova, E., Sheshkus, A., and
Arlazarov, V. V. (2021). Midv-lait: a challenging
dataset for recognition of ids with perso-arabic, thai,
and indian scripts. In International Conference on
Document Analysis and Recognition, pages 258–272.
Springer.
de S
´
a Soares, A., das Neves Junior, R. B., and Bezerra,
B. L. D. (2020). Bid dataset: a challenge dataset for
document processing tasks. In Anais Estendidos do
XXXIII Conference on Graphics, Patterns and Images,
pages 143–146. SBC.
Goecks, V. G., Waytowich, N., Watkins, D., and Prakash, B.
(2021). Combining learning from human feedback and
knowledge engineering to solve hierarchical tasks in
minecraft. arXiv preprint arXiv:2112.03482.
He, C., Li, S., So, J., Zeng, X., Zhang, M., Wang, H., Wang,
X., Vepakomma, P., Singh, A., Qiu, H., et al. (2020).
Fedml: A research library and benchmark for federated
machine learning. arXiv preprint arXiv:2007.13518.
Huang, Y., Ma, X., Fan, X., Liu, J., and Gong, W. (2017).
When deep learning meets edge computing. In
2017 IEEE 25th international conference on network
protocols (ICNP), pages 1–2. IEEE.
Kairouz, P., McMahan, H. B., Avent, B., Bellet, A.,
Bennis, M., Bhagoji, A. N., Bonawitz, K., Charles, Z.,
Cormode, G., Cummings, R., et al. (2021). Advances
and open problems in federated learning. Foundations
and Trends® in Machine Learning, 14(1–2):1–210.
Kang, L., Kumar, J., Ye, P., Li, Y., and Doermann, D. (2014).
Convolutional neural networks for document image
classification. In 2014 22nd International Conference
on Pattern Recognition, pages 3168–3172. IEEE.
Kingma, D. P. and Ba, J. (2014). Adam: A
method for stochastic optimization. arXiv preprint
arXiv:1412.6980.
Kirkpatrick, J., Pascanu, R., Rabinowitz, N., Veness, J.,
Desjardins, G., Rusu, A. A., Milan, K., Quan, J.,
Ramalho, T., Grabska-Barwinska, A., et al. (2017).
Overcoming catastrophic forgetting in neural networks.
Proceedings of the national academy of sciences,
114(13):3521–3526.
Koh, P. W., Sagawa, S., Marklund, H., Xie, S. M., Zhang,
M., Balsubramani, A., Hu, W., Yasunaga, M., Phillips,
R. L., Gao, I., et al. (2021). Wilds: A benchmark
of in-the-wild distribution shifts. In International
Conference on Machine Learning.
Kontar, R., Shi, N., Yue, X., Chung, S., Byon, E.,
Chowdhury, M., Jin, J., Kontar, W., Masoud, N.,
Nouiehed, M., et al. (2021). The internet of federated
things (ioft). IEEE Access.
LGPD (2018). General law for personal data protection
(lgpd). http://www.planalto.gov.br/ccivil 03/
ato2015-2018/2018/lei/L13709.htm. Accessed:
2022-09-19.
Li, Q., Wen, Z., Wu, Z., Hu, S., Wang, N., Li, Y., Liu, X.,
and He, B. (2021). A survey on federated learning
systems: vision, hype and reality for data privacy and
protection. IEEE Transactions on Knowledge and Data
Engineering.
Li, T., Sahu, A. K., Talwalkar, A., and Smith, V. (2020).
Federated learning: Challenges, methods, and future
directions. IEEE Signal Processing Magazine.
Li, W., Milletar
`
ı, F., Xu, D., Rieke, N., Hancox, J., Zhu, W.,
Baust, M., Cheng, Y., Ourselin, S., Cardoso, M. J., et al.
(2019). Privacy-preserving federated brain tumour
segmentation. In International workshop on machine
learning in medical imaging.
Liu, L., Wang, Z., Qiu, T., Chen, Q., Lu, Y., and Suen, C. Y.
(2021). Document image classification: Progress over
two decades. Neurocomputing, 453:223–240.
Liu, Y., Huang, A., Luo, Y., Huang, H., Liu, Y., Chen,
Y., Feng, L., Chen, T., Yu, H., and Yang, Q. (2020).
Fedvision: An online visual object detection platform
powered by federated learning. In Proceedings of the
AAAI Conference on Artificial Intelligence.
FedBID and FedDocs: A Dataset and System for Federated Document Analysis
557