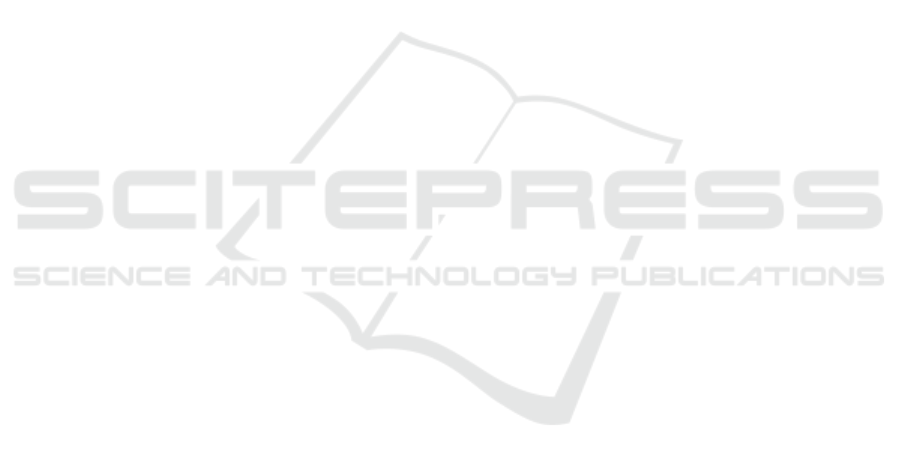
datasets would be preferable, however the availability
of such datasets that contain labeled CT scans of full
thoracic field of view is limited.
ACKNOWLEDGEMENTS
The authors acknowledge the National Cancer Insti-
tute and the Foundation for the National Institutes of
Health, and their critical role in the creation of the free
publicly available (Clark et al., 2013b) LIDC/IDRI
Database (Armato III, Samuel G. et al., 2015) used
in this study; and also the Multi-national NIH Con-
sortium for CT AI in COVID-19. This research was
funded by the National Research, Development, and
Innovation Fund of Hungary under Grant TKP2021-
EGA-02.
REFERENCES
Altini, N., De Giosa, G., Fragasso, N., Coscia, C., Sibi-
lano, E., Prencipe, B., Hussain, S. M., Brunetti, A.,
Buongiorno, D., Guerriero, A., et al. (2021). Segmen-
tation and identification of vertebrae in ct scans using
cnn, k-means clustering and k-nn. In Informatics, vol-
ume 8, page 40. Multidisciplinary Digital Publishing
Institute.
An, P., Xu, S., Harmon, S. A., Turkbey, E. B., Sanford,
T. H., Amalou, A., Kassin, M., Varble, N., Blain,
M., Anderson, V., Patella, F., Carrafiello, G., Turkbey,
B. T., and Wood, B. J. (2020). Ct images in covid-19.
Armato, S., MacMahon, H., Engelmann, R., Roberts, R.,
Starkey, A., Caligiuri, P., McLennan, G., Bidaut,
L., Qing, D., McNitt-Gray, M., et al. (2011). The
lung image database consortium (lidc) and image
database resource initiative (idri): A completed ref-
erence database of lung nodules on ct scans. Medical
Physics, 38(2):915–931.
Armato III, Samuel G., McLennan, G., Bidaut, L., et al.
(2015). Data from lidc-idri.
Athertya, J. S. and Kumar, G. S. (2016). Automatic seg-
mentation of vertebral contours from ct images using
fuzzy corners. Computers in biology and medicine,
72:75–89.
Castro-Mateos, I., Pozo, J. M., Perea
˜
nez, M., Lekadir, K.,
Lazary, A., and Frangi, A. F. (2015). Statistical in-
terspace models (sims): application to robust 3d spine
segmentation. IEEE transactions on medical imaging,
34(8):1663–1675.
Clark, K., Vendt, B., Smith, K., Freymann, J., Kirby, J.,
Koppel, P., Moore, S., Phillips, S., Maffitt, D., Pringle,
M., et al. (2013a). The cancer imaging archive (tcia):
maintaining and operating a public information repos-
itory. Journal of digital imaging, 26(6):1045–1057.
Clark, K., Vendt, B., Smith, K., Freymann, J., Kirby, J.,
Koppel, P., Moore, S., Phillips, S., Maffitt, D., Pringle,
M., Tarbox, L., and Prior, F. (2013b). The cancer
imaging archive (TCIA): Maintaining and operating
a public information repository. Journal of Digital
Imaging, 26(6):1045–1057.
Deng, Y., Wang, C., Hui, Y., Li, Q., Li, J., Luo, S., Sun, M.,
Quan, Q., Yang, S., Hao, Y., et al. (2021). Ctspine1k:
A large-scale dataset for spinal vertebrae segmenta-
tion in computed tomography. arXiv e-prints, pages
arXiv–2105.
Fischler, M. A. and Bolles, R. C. (1981). Random sample
consensus: a paradigm for model fitting with appli-
cations to image analysis and automated cartography.
Communications of the ACM, 24(6):381–395.
Gonzalez, R. (2009). Digital Image Processing. Pearson
Education.
Harmon, S. A., Sanford, T. H., Xu, S., Turkbey, E. B., Roth,
H., Xu, Z., Yang, D., Myronenko, A., Anderson, V.,
Amalou, A., et al. (2020). Artificial intelligence for
the detection of covid-19 pneumonia on chest ct us-
ing multinational datasets. Nature communications,
11(1):1–7.
Ibragimov, B., Korez, R., Likar, B., Pernu
ˇ
s, F., Xing, L.,
and Vrtovec, T. (2017). Segmentation of pathological
structures by landmark-assisted deformable models.
IEEE transactions on medical imaging, 36(7):1457–
1469.
Lessmann, N., Van Ginneken, B., De Jong, P. A., and
I
ˇ
sgum, I. (2019). Iterative fully convolutional neu-
ral networks for automatic vertebra segmentation and
identification. Medical image analysis, 53:142–155.
Ronneberger, O., Fischer, P., and Brox, T. (2015). U-net:
Convolutional networks for biomedical image seg-
mentation. In International Conference on Medical
image computing and computer-assisted intervention,
pages 234–241. Springer.
Sekuboyina, A., Bayat, A., Husseini, M. E., L
¨
offler, M.,
Rempfler, M., Kuka
ˇ
cka, J., Tetteh, G., Valentinitsch,
A., Payer, C., Urschler, M., et al. (2020). Verse: a ver-
tebrae labelling and segmentation benchmark. arXiv.
org e-Print archive.
Sekuboyina, A., Kuka
ˇ
cka, J., Kirschke, J. S., Menze,
B. H., and Valentinitsch, A. (2017). Attention-driven
deep learning for pathological spine segmentation. In
International Workshop on Computational Methods
and Clinical Applications in Musculoskeletal Imag-
ing, pages 108–119. Springer.
Automatic Spine Segmentation in CT Scans
93