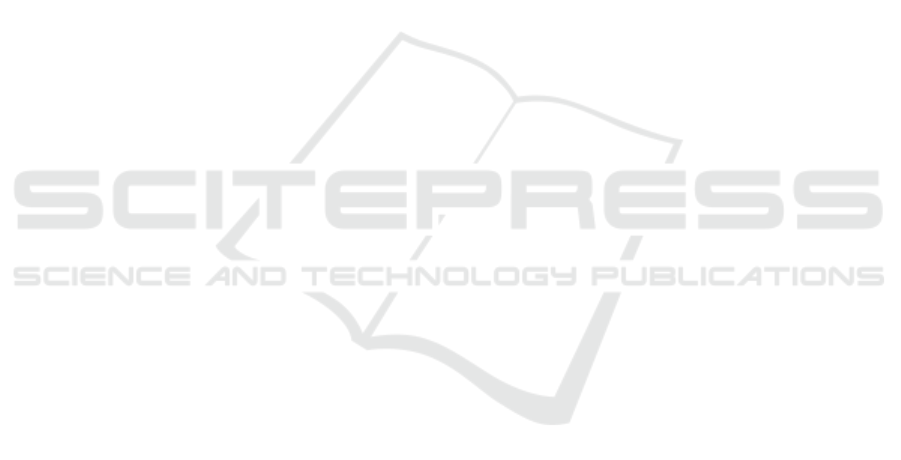
Chandra, R., Goyal, S., and Gupta, R. (2021). Evaluation of
deep learning models for multi-step ahead time series
prediction. IEEE Access, 9:83105–83123.
Cherif, A., Cardot, H., and Bon
´
e, R. (2011). Som time
series clustering and prediction with recurrent neural
networks. Neurocomputing, 74(11):1936–1944.
Franceschi, J.-Y., Dieuleveut, A., and Jaggi, M. (2020). Un-
supervised scalable representation learning for multi-
variate time series.
Grigsby, J., Wang, Z., and Qi, Y. (2021). Long-range
transformers for dynamic spatiotemporal forecasting.
arXiv preprint arXiv:2109.12218.
Hewamalage, H., Bergmeir, C., and Bandara, K. (2021).
Recurrent neural networks for time series forecast-
ing: Current status and future directions. International
Journal of Forecasting, 37(1):388–427.
Karunasinghe, D. S. and Liong, S.-Y. (2006). Chaotic time
series prediction with a global model: Artificial neural
network. Journal of Hydrology, 323(1-4):92–105.
Kohonen, T. (1982). Self-organized formation of topolog-
ically correct feature maps. Biological cybernetics,
43(1):59–69.
Lara-Ben
´
ıtez, P., Carranza-Garc
´
ıa, M., and Riquelme, J. C.
(2021). An experimental review on deep learning ar-
chitectures for time series forecasting. International
journal of neural systems, 31(03):2130001.
Liao, T. W. (2005). Clustering of time series data—a survey.
Pattern recognition, 38(11):1857–1874.
Liu, F. and Deng, Y. (2020). Determine the number of un-
known targets in open world based on elbow method.
IEEE Transactions on Fuzzy Systems, 29(5):986–995.
Mart
´
ınez-Rego, D., Fontenla-Romero, O., and Alonso-
Betanzos, A. (2011). Efficiency of local models en-
sembles for time series prediction. Expert Systems
with Applications, 38(6):6884–6894.
Marutho, D., Handaka, S. H., Wijaya, E., et al. (2018).
The determination of cluster number at k-mean us-
ing elbow method and purity evaluation on headline
news. In 2018 international seminar on applica-
tion for technology of information and communica-
tion, pages 533–538. IEEE.
Montero-Manso, P. and Hyndman, R. J. (2021). Principles
and algorithms for forecasting groups of time series:
Locality and globality. International Journal of Fore-
casting, 37(4):1632–1653.
Oussous, A., Benjelloun, F.-Z., Lahcen, A. A., and Belfkih,
S. (2018). Big data technologies: A survey. Journal
of King Saud University-Computer and Information
Sciences, 30(4):431–448.
Pan, M., Zhou, H., Cao, J., Liu, Y., Hao, J., Li, S., and Chen,
C.-H. (2020). Water level prediction model based on
gru and cnn. Ieee Access, 8:60090–60100.
Papacharalampous, G., Tyralis, H., and Koutsoyiannis, D.
(2018). Univariate time series forecasting of tempera-
ture and precipitation with a focus on machine learn-
ing algorithms: A multiple-case study from greece.
Water resources management, 32(15):5207–5239.
Pavlidis, N. G., Plagianakos, V. P., Tasoulis, D. K., and Vra-
hatis, M. N. (2006). Financial forecasting through
unsupervised clustering and neural networks. Oper-
ational Research, 6(2):103–127.
Rangapuram, S. S., Seeger, M. W., Gasthaus, J., Stella, L.,
Wang, Y., and Januschowski, T. (2018). Deep state
space models for time series forecasting. Advances in
neural information processing systems, 31.
Rani, S. and Sikka, G. (2012). Recent techniques of cluster-
ing of time series data: a survey. International Journal
of Computer Applications, 52(15).
Sajjad, M., Khan, Z. A., Ullah, A., Hussain, T., Ullah, W.,
Lee, M. Y., and Baik, S. W. (2020). A novel cnn-gru-
based hybrid approach for short-term residential load
forecasting. Ieee Access, 8:143759–143768.
Sen, R., Yu, H.-F., and Dhillon, I. S. (2019). Think glob-
ally, act locally: A deep neural network approach to
high-dimensional time series forecasting. Advances
in neural information processing systems, 32.
Sfetsos, A. and Siriopoulos, C. (2004). Time series fore-
casting with a hybrid clustering scheme and pattern
recognition. IEEE Transactions on Systems, Man, and
Cybernetics-Part A: Systems and Humans, 34(3):399–
405.
Stoean, R., Stoean, C., Becerra-Garc
´
ıa, R., Garc
´
ıa-
Berm
´
udez, R., Atencia, M., Garc
´
ıa-Lagos, F.,
Vel
´
azquez-P
´
erez, L., and Joya, G. (2020). A hy-
brid unsupervised—deep learning tandem for elec-
trooculography time series analysis. Plos one,
15(7):e0236401.
Syakur, M., Khotimah, B., Rochman, E., and Satoto, B. D.
(2018). Integration k-means clustering method and
elbow method for identification of the best customer
profile cluster. In IOP conference series: materials
science and engineering, volume 336, page 012017.
IOP Publishing.
Tadayon, M. and Iwashita, Y. (2020). A clustering approach
to time series forecasting using neural networks: A
comparative study on distance-based vs. feature-based
clustering methods. arXiv preprint arXiv:2001.09547.
Wan, R., Mei, S., Wang, J., Liu, M., and Yang, F. (2019).
Multivariate temporal convolutional network: A deep
neural networks approach for multivariate time series
forecasting. Electronics, 8(8):876.
Wang, S. and Jiang, J. (2015). Learning natural language
inference with lstm. arXiv preprint arXiv:1512.08849.
Yatish, H. and Swamy, S. (2020). Recent trends in
time series forecasting–a survey. International Re-
search Journal of Engineering and Technology (IR-
JET), 7(04):5623–5628.
Yu, J., Zhang, X., Xu, L., Dong, J., and Zhangzhong, L.
(2021). A hybrid cnn-gru model for predicting soil
moisture in maize root zone. Agricultural Water Man-
agement, 245:106649.
Zhang, Y., Luo, L., Yang, J., Liu, D., Kong, R., and Feng, Y.
(2019). A hybrid arima-svr approach for forecasting
emergency patient flow. Journal of Ambient Intelli-
gence and Humanized Computing, 10(8):3315–3323.
ICAART 2023 - 15th International Conference on Agents and Artificial Intelligence
148