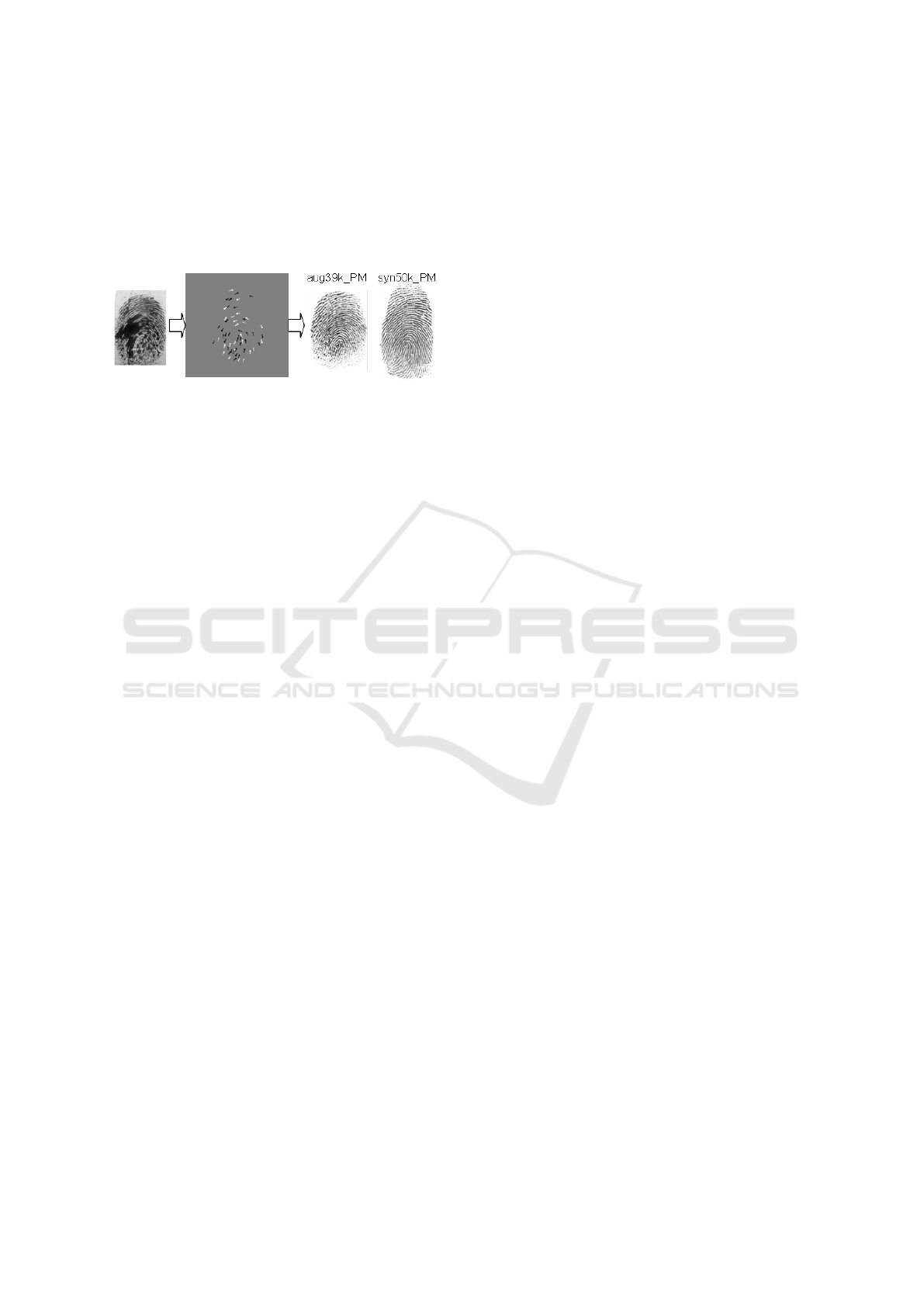
with both models aug39k and syn50k. The 15 epoch
snapshots are utilized. Examples with 30 and 55
epoch snapshots can be found on our website. The
images show that our models perform a style transfer
i.e. the appearance of resulting fingerprints is similar
to those captured with a CrossMatch sensor.
Figure 7: Reconstruction example of a URU fingerprint.
Although the ridge patterns in reconstructed sam-
ples are not exactly the same as in target fingerprints,
the minutiae co-allocation is reproduced accurately
enough to enable matching with the source of minu-
tiae. Hence, we state that pix2pix in conjunction with
PM or DL encoding is a valid approach for fingerprint
reconstruction from minutiae. We have also shown
that the pix2pix architecture is scalable to larger im-
ages and training with 512x512 pixel images can be
done within a reasonable time frame.
6 CONCLUSION
Reconstruction of realistic fingerprints from minu-
tiae is an important step towards controlled genera-
tion of high-quality datasets of synthetic fingerprints.
Since, the minutiae co-allocation defines the finger-
print’s identity, reconstruction from pseudo-random
minutiae maps ensures anonymity and diversity of re-
sulting patterns and enables synthesis of mated fin-
gerprints. This paper introduces and compares four
pix2pix models trained with fingerprint images of
512x512 pixels at fingerprint-native resolution from
real and synthetic datasets with two types of minu-
tiae encoding. Our experiments show that a pix2pix
network is a valid solution to the reconstruction prob-
lem with a scalable architecture enabling training
with 512x512 pixel images, that reconstructed ridge
patterns appear realistic, that pointing minutiae en-
coding is superior to directed line encoding, that an
augmented dataset of 39k real fingerprints used for
training is superior to a dataset of 50k synthetic fin-
gerprints, but if pointing minutiae encoding is ap-
plied, the difference in reconstruction performances
between real and synthetic training data is lower than
1.7%. Future work will be devoted to compilation of
a large-scale synthetic fingerprint dataset appropriate
for evaluation of fingerprint matching algorithms.
ACKNOWLEDGEMENTS
This research has been funded in part by the Deutsche
Forschungsgemeinschaft (DFG) through the research
project GENSYNTH under the number 421860227.
REFERENCES
Ansari, A. H. (2011). Generation and storage of large syn-
thetic fingerprint database. M.E. Thesis, Indian Insti-
tute of Science Bangalore.
Bahmani, K., Plesh, R., Johnson, P., Schuckers, S., and
Swyka, T. (2021). High fidelity fingerprint generation:
Quality, uniqueness, and privacy. In Proc. ICIP’21,
pages 3018–3022.
Bouzaglo, R. and Keller, Y. (2022). Synthesis and recon-
struction of fingerprints using generative adversarial
networks. CoRR, abs/2201.06164.
Cao, K. and Jain, A. K. (2015). Learning fingerprint re-
construction: From minutiae to image. IEEE TIFS,
10:104–117.
Cappelli, R. (2009). SFinGe. In Li, S. Z. and Jain, A., ed-
itors, Encyclopedia of Biometrics, pages 1169–1176.
Springer US, Boston, MA.
Cappelli, R., Maio, D., Lumini, A., and Maltoni, D. (2007).
Fingerprint image reconstruction from standard tem-
plates. IEEE PAMI, 29:1489–1503.
Feng, J. and Jain, A. (2009). FM model based fingerprint re-
construction from minutiae template. In Proc. ICB’09,
pages 544–553.
Isola, P., Zhu, J.-Y., Zhou, T., and Efros, A. A. (2017).
Image-to-image translation with conditional adversar-
ial networks. In Proc. CVPR’17, pages 5967–5976.
Karras, T., Aittala, M., Hellsten, J., Laine, S., Lehtinen, J.,
and Aila, T. (2020). Training generative adversarial
networks with limited data. CoRR, abs/2006.06676.
Karras, T., Laine, S., and Aila, T. (2018). A style-based
generator architecture for generative adversarial net-
works. CoRR, abs/1812.04948.
Karras, T., Laine, S., Aittala, M., Hellsten, J., Lehtinen, J.,
and Aila, T. (2019). Analyzing and improving the im-
age quality of StyleGAN. CoRR, abs/1912.04958.
Kim, H., Cui, X., Kim, M.-G., and Nguyen, T.-H.-B.
(2019). Reconstruction of fingerprints from minu-
tiae using conditional adversarial networks. In Proc.
IWDW’18, pages 353–362.
Li, S. and Kot, A. C. (2012). An improved scheme for
full fingerprint reconstruction. IEEE TIFS, 7(6):1906–
1912.
Makrushin, A., Kauba, C., Kirchgasser, S., Seidlitz, S.,
Kraetzer, C., Uhl, A., and Dittmann, J. (2021). Gen-
eral requirements on synthetic fingerprint images for
biometric authentication and forensic investigations.
In Proc. IH&MMSec’21, page 93–104.
Makrushin, A., Mannam, V. S., B.N, M. R., and Dittmann,
J. (2022). Data-driven reconstruction of fingerprints
from minutiae maps. In Proc. MMSP’22.
VISAPP 2023 - 18th International Conference on Computer Vision Theory and Applications
236