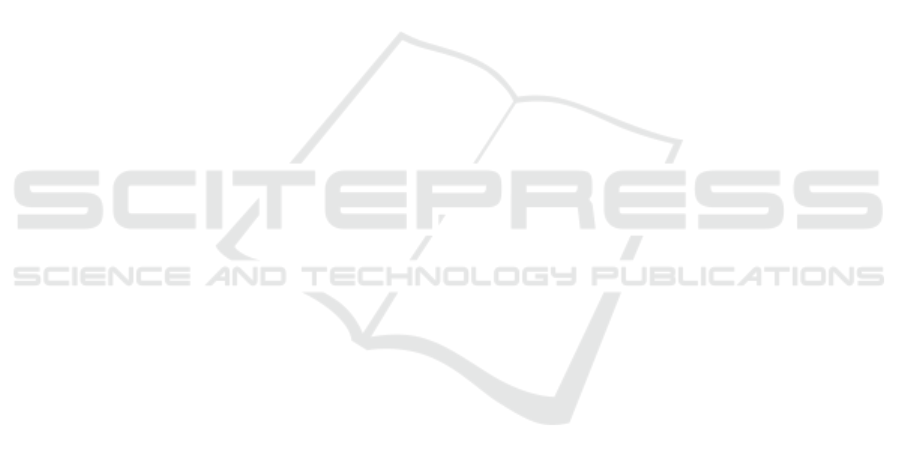
mance. For instance, designing robust mixture-based
probabilistic SVM kernels can help with this. Last but
not least, it is crucial to emphasize that the output of
various statistical MM models frequently depends on
the dataset’s sample size.
4 CONCLUSION
Mixture models (MM), an emerging statistical
method for modeling complex multimodal data,
is discussed in this paper. The current study presents
a recent brief review of the advances in MM mod-
els. Although there hasn’t been much research on
MM-based methods, and only a few publications have
time-varying indicators, we are optimistic that more
significant and insightful results will soon be made
available to the public.
REFERENCES
Akaike, H. (1974). A new look at the statistical model iden-
tification. IEEE Transactions on Automatic Control,
19(6):716–723.
Alharithi, F. S., Almulihi, A. H., Bourouis, S., Alroobaea,
R., and Bouguila, N. (2021). Discriminative learning
approach based on flexible mixture model for med-
ical data categorization and recognition. Sensors,
21(7):2450.
Allili, M. S., Bouguila, N., and Ziou, D. (2008). Finite
general gaussian mixture modeling and application to
image and video foreground segmentation. Journal of
Electronic Imaging, 17(1):013005–013005.
Alroobaea, R., Rubaiee, S., Bourouis, S., Bouguila, N.,
and Alsufyani, A. (2020). Bayesian inference frame-
work for bounded generalized gaussian-based mixture
model and its application to biomedical images clas-
sification. Int. J. Imaging Systems and Technology,
30(1):18–30.
Azam, M. and Bouguila, N. (2022). Multivariate bounded
support asymmetric generalized gaussian mixture
model with model selection using minimum mes-
sage length. Expert Systems with Applications,
204:117516.
Bouguila, N. (2011). Bayesian hybrid generative discrimi-
native learning based on finite liouville mixture mod-
els. Pattern Recognit., 44(6):1183–1200.
Bourouis, S., Alroobaea, R., Rubaiee, S., Andejany, M., Al-
mansour, F. M., and Bouguila, N. (2021a). Markov
chain monte carlo-based bayesian inference for learn-
ing finite and infinite inverted beta-liouville mixture
models. IEEE Access, 9:71170–71183.
Bourouis, S., Alroobaea, R., Rubaiee, S., Andejany, M., and
Bouguila, N. (2021b). Nonparametric bayesian learn-
ing of infinite multivariate generalized normal mixture
models and its applications. Applied Sciences, 11(13).
Bourouis, S. and Bouguila, N. (2021). Nonparametric learn-
ing approach based on infinite flexible mixture model
and its application to medical data analysis. Int. J.
Imaging Syst. Technol., 31(4):1989–2002.
Bourouis, S. and Bouguila, N. (2022). Unsupervised learn-
ing using expectation propagation inference of in-
verted beta-liouville mixture models for pattern recog-
nition applications. Cybernetics and Systems, pages
1–25.
Bourouis, S., Sallay, H., and Bouguila, N. (2021c). A com-
petitive generalized gamma mixture model for medi-
cal image diagnosis. IEEE Access, 9:13727–13736.
Bourouis, S., Zaguia, A., Bouguila, N., and Alroobaea, R.
(2019). Deriving probabilistic SVM kernels from flex-
ible statistical mixture models and its application to
retinal images classification. IEEE Access, 7:1107–
1117.
Bouveyron, C. and Girard, S. (2009). Robust super-
vised classification with mixture models: Learning
from data with uncertain labels. Pattern Recognition,
42(11):2649–2658.
Brooks, S. P. (2001). On bayesian analyses and finite mix-
tures for proportions. Stat. Comput., 11(2):179–190.
Channoufi, I., Bourouis, S., Bouguila, N., and Hamrouni,
K. (2018). Spatially constrained mixture model with
feature selection for image and video segmentation. In
Image and Signal Processing - 8th International Con-
ference, ICISP France, pages 36–44.
Cheng, N., Cao, C., Yang, J., Zhang, Z., and Chen, Y.
(2022). A spatially constrained skew student’s-t mix-
ture model for brain mr image segmentation and bias
field correction. Pattern Recognition, 128:108658.
Csurka, G., Dance, C., Fan, L., Willamowski, J., and Bray,
C. (2004). Visual categorization with bags of key-
points. In Workshop on statistical learning in com-
puter vision, ECCV, volume 1, pages 1–2. Prague.
Fan, W. and Bouguila, N. (2020). Modeling and clustering
positive vectors via nonparametric mixture models of
liouville distributions. IEEE Trans. Neural Networks
Learn. Syst., 31(9):3193–3203.
Fu, Y., Liu, X., Sarkar, S., and Wu, T. (2021). Gaus-
sian mixture model with feature selection: An embed-
ded approach. Computers and Industrial Engineering,
152:107000.
Fujimaki, R., Sogawa, Y., and Morinaga, S. (2011). On-
line heterogeneous mixture modeling with marginal
and copula selection. In International Conference
on Knowledge Discovery and Data Mining, SIGKDD,
USA, pages 645–653.
Hofmann, T. (2001). Unsupervised learning by probabilistic
latent semantic analysis. Mach. Learn., 42(1/2):177–
196.
Hu, C., Fan, W., Du, J., and Bouguila, N. (2019). A novel
statistical approach for clustering positive data based
on finite inverted beta-liouville mixture models. Neu-
rocomputing, 333:110–123.
Husmeier, D. (2000). The bayesian evidence scheme for
regularizing probability-density estimating neural net-
works. Neural Computation, 12(11):2685–2717.
ICPRAM 2023 - 12th International Conference on Pattern Recognition Applications and Methods
318