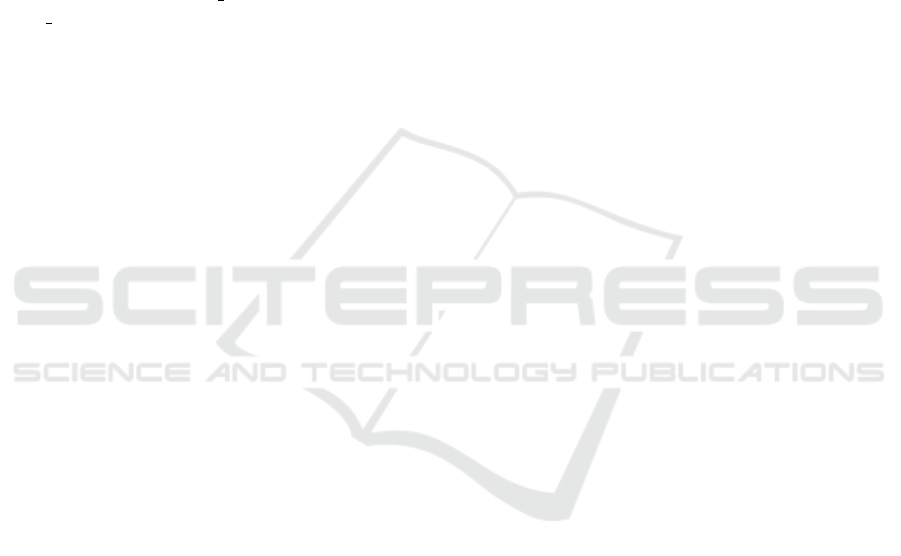
ACKNOWLEDGEMENTS
This work was supported by JSPS KAKENHI
Grant Numbers JP8K18517, JP22H00661, and JST
SPRING Grant Number JPMJSP2112.
AVAILABILITY OF DATA
The datasets generated and/or analyzed dur-
ing the current study are available under
the license in the NIT-UVEC-OMNI repos-
itory https://drive.google.com/drive/folders/
1SbdaCIDhijvYdaFDdRiL NTlRwk9xc00?usp=
share link.
REFERENCES
Loper, M., Mahmood, N., Romero, J., Pons-Moll, G., and
Black, M. J. (2015). SMPL: a skinned multi-person
linear model. ACM Transactions on Graphics, 34(6).
Martinez, J., Hossain, R., Romero, J., and Little, J. J.
(2017). A Simple Yet Effective Baseline for 3d Hu-
man Pose Estimation. In IEEE International Confer-
ence on Computer Vision, pages 2659–2668.
Mehta, D., Sridhar, S., Sotnychenko, O., Rhodin, H.,
Shafiei, M., Seidel, H.-P., Xu, W., Casas, D., and
Theobalt, C. (2017). VNect: Real-time 3D Human
Pose Estimation with a Single RGB Camera. ACM
Transactions on Graphics, 36:1–14.
Miura, T. and Sako, S. (2022). Simple yet effective 3D
ego-pose lift-up based on vector and distance for a
mounted omnidirectional camera. Applied Intelli-
gence.
Newell, A., Yang, K., and Deng, J. (2016). Stacked Hour-
glass Networks for Human Pose Estimation. In Euro-
pean Conference on Computer Vision, pages 483–499.
Pavlakos, G., Zhou, X., Derpanis, K. G., and Daniilidis,
K. (2017). Coarse-to-Fine Volumetric Prediction for
Single-Image 3D Human Pose. In IEEE Conference
on Computer Vision and Pattern Recognition, pages
1263–1272.
Sandler, M., Howard, A., Zhu, M., Zhmoginov, A., and
Chen, L.-C. (2018). MobileNetV2: Inverted Resid-
uals and Linear Bottlenecks. In IEEE Conference
on Computer Vision and Pattern Recognition, pages
4510–4520, New York, NY, USA. IEEE.
Scaramuzza, D., Martinelli, A., and Siegwart, R. (2006). A
Toolbox for Easily Calibrating Omnidirectional Cam-
eras. In IEEE/RSJ International Conference on Intel-
ligent Robots and Systems, pages 5695–5701.
Sun, K., Xiao, B., Liu, D., and Wang, J. (2019). Deep High-
Resolution Representation Learning for Human Pose
Estimation. In IEEE Conference on Computer Vision
and Pattern Recognition, pages 5686–5696.
Tekin, B., Katircioglu, I., Salzmann, M., Lepetit, V., and
Fua, P. (2016). Structured Prediction of 3D Human
Pose with Deep Neural Networks. In British Machine
Vision Conference, pages 130.1–130.11.
Tome, D., Alldieck, T., Peluse, P., Pons-Moll, G., Agapito,
L., Badino, H., and la Torre, F. D. (2020). Self-
Pose: 3D Egocentric Pose Estimation from a Head-
set Mounted Camera. IEEE Transactions on Pattern
Analysis and Machine Intelligence, pages 1–1.
Tome, D., Peluse, P., Agapito, L., and Badino, H. (2019).
xR-EgoPose: Egocentric 3D Human Pose From an
HMD Camera. In The IEEE International Conference
on Computer Vision, pages 7727–7737.
Varol, G., Romero, J., Martin, X., Mahmood, N., Black,
M. J., Laptev, I., and Schmid, C. (2017). Learning
from Synthetic Humans. In IEEE Conference on Com-
puter Vision and Pattern Recognition, pages 4627–
4635.
Wang, J., Liu, L., Xu, W., Sarkar, K., Luvizon, D., and
Theobalt, C. (2022). Estimating Egocentric 3D Hu-
man Pose in the Wild With External Weak Supervi-
sion. In IEEE Conference on Computer Vision and
Pattern Recognition, pages 13157–13166.
Wei, S.-E., Ramakrishna, V., Kanade, T., and Sheikh, Y.
(2016). Convolutional Pose Machines. In IEEE Con-
ference on Computer Vision and Pattern Recognition,
pages 4724–4732.
Xiao, B., Wu, H., and Wei, Y. (2018). Simple Baselines for
Human Pose Estimation and Tracking. In European
Conference on Computer Vision, pages 472–487.
Xiaowei, Z., Menglong, Z., Spyridon, L., and Kostas, D.
(2017). Sparse Representation for 3D Shape Estima-
tion: A Convex Relaxation Approach. IEEE Trans-
actions on Pattern Analysis and Machine Intelligence,
39(8):1648–1661.
Xu, W., Chatterjee, A., Zollh
¨
ofer, M., Rhodin, H., Fua, P.,
Seidel, H.-P., and Theobalt, C. (2019). Mo2Cap2:
Real-time Mobile 3D Motion Capture with a Cap-
mounted Fisheye Camera. IEEE Transactions on
Visualization and Computer Graphics, 25(5):2093–
2101.
Zhou, X., Sun, X., Zhang, W., Liang, S., and Wei, Y. (2016).
Deep Kinematic Pose Regression. In European Con-
ference on Computer Vision, pages 186–201.
VISAPP 2023 - 18th International Conference on Computer Vision Theory and Applications
606