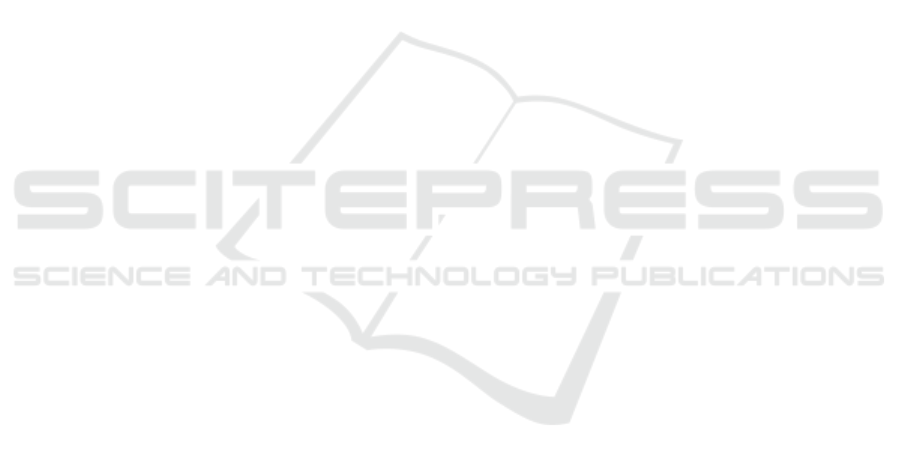
6 CONCLUSIONS
There exist potential avenues for future work. Quantita-
tively analyzing natural language is faster than manual
annotation and less subject to researcher bias. The
description of insight differences provided by this
study can give researchers a general understanding
of how different visualizations enable insight genera-
tion. When used in combination with insight complex-
ity metrics, the results provide a more holistic view
of participant insights. The insight analysis method
currently only analyzes insights on a per-word basis.
Extending the method to look at phrases, rather than
individual words, may yield interesting results. In this
case, a phrase-based approach may better capture the
idea of groups of points such as “aquatic animals” or
“physical traits”. Within education research, the insight
analysis may also be helpful to develop an automatic
grading scheme of natural language insights.
The presented case study analysis identifies differ-
ences in insight complexity and vocabulary within
Andromeda
. Across all interaction types available
within
Andromeda
, the dimensionality of insights in-
creases with its usage. While the insights see con-
sistent complexity changes, their vocabulary differs
based on the interaction types available. When compar-
ing insights generated with parametric interaction and
observation-level interaction, it is clear that insights
generated with parametric interaction are associated
with WMDS-related terminology, while insights gener-
ated with observation-level interaction tend to describe
WMDS interpretations of relationships in the data. The
analysis method presented in this work can be applied
and improved to further visualization research that
seeks to understand through automated processes.
REFERENCES
Amar, R. A., Eagan, J. R., and Stasko, J. T. (2005). Low-
level components of analytic activity in information
visualization. In INFOVIS.
Andromeda Website. Andromeda: Semantic interaction
for dimension reduction. https://infovis.cs.vt.edu/
andromeda.
Benjamini, Y. and Hochberg, Y. (1995). Controlling the
false discovery rate: A practical and powerful approach
to multiple testing. Journal of the Royal Statistical
Society Series B (Methodological), 57(1):289–300.
Card, S. K., Mackinlay, J. D., and Shneiderman, B., editors
(1999). Readings in Information Visualization: Using
Vision to Think. Morgan Kaufmann Publishers Inc.,
San Francisco, CA, USA.
Endert, A., Han, C., Maiti, D., House, L., Leman, S., and
North, C. (2011). Observation-level interaction with
statistical models for visual analytics. In Visual An-
alytics Science and Technology (VAST) 2011 IEEE
Conference, pages 121–130.
Gomez, S. R., Guo, H., Ziemkiewicz, C., and Laidlaw, D. H.
(2014). An insight- and task-based methodology for
evaluating spatiotemporal visual analytics. In 2014
IEEE Conference on Visual Analytics Science and
Technology (VAST), pages 63–72.
He, C., Micallef, L., He, L., Peddinti, G., Aittokallio, T., and
Jacucci, G. (2021). Characterizing the quality of in-
sight by interactions: A case study. IEEE Transactions
on Visualization & Computer Graphics, 27(08):3410–
3424.
House, L., Leman, S., and Han, C. (2015). Bayesian Visual
Analytics (BaVA). Journal of Statistical Analysis and
Data Mining, 8(2):1–13.
Kruskal, J. and Wish, M. (1978). Multidimensional Scaling.
SAGE Publications, Inc.
North, C. (2006). Toward measuring visualization insight.
IEEE Computer Graphics and Applications, 26(3):6–9.
O’Brien, T., Ritz, A., Raphael, B., and Laidlaw, D. (2011).
Gremlin: An interactive visualization model for ana-
lyzing genomic rearrangements. IEEE transactions on
visualization and computer graphics, 16:918–26.
Saraiya, P., North, C., and Duca, K. (2005). An insight-
based methodology for evaluating bioinformatics vi-
sualizations. IEEE Transactions on Visualization and
Computer Graphics, 11(4):443–456.
Self, J. Z., Dowling, M., Wenskovitch, J., Crandell, I.,
Wang, M., House, L., Leman, S., and North, C. (2018).
Observation-level and parametric interaction for high-
dimensional data analysis. ACM Transactions on Inter-
active Intelligent Systems, 8(2).
Self, J. Z., House, L., Leman, S., and North, C. (2015). An-
dromeda: Observation-level and parametric interaction
for exploratory data analysis. Blacksburg.
Self, J. Z., Hu, X., House, L., Leman, S., and North, C.
(2016). Designing usable interactive visual analytics
tools for dimension reduction. In CHI 2016 Work-
shop on Human-Centered Machine Learning (HCML),
volume May, page 7.
Self, J. Z., Self, N., House, L., Evia, J. R., Leman, S.,
and North, C. (2017). Bringing interactive visual an-
alytics to the classroom for developing eda skills. In
Proceedings of the 33rd Annual Consortium of Com-
puting Sciences in Colleges (CCSC) Eastern Regional
Conference, page 10.
Self, J. Z., Self, N., House, L., Leman, S., and North, C.
(2014). Improving students’ cognitive dimensionality
through education with object-level interaction.
Xian, Y., Lampert, C. H., Schiele, B., and Akata, Z. (2019).
Zero-shot learning—a comprehensive evaluation of
the good, the bad and the ugly. IEEE Transactions on
Pattern Analysis and Machine Intelligence, 41(9):2251–
2265.
Zeitz, J., Self, N., House, L., Evia, J. R., Leman, S., and
North, C. (2018). Bringing interactive visual analytics
to the classroom for developing eda skills. Journal of
Computing Sciences in Colleges, 33(3):115–125.
Evaluating Differences in Insights from Interactive Dimensionality Reduction Visualizations Through Complexity and Vocabulary
165