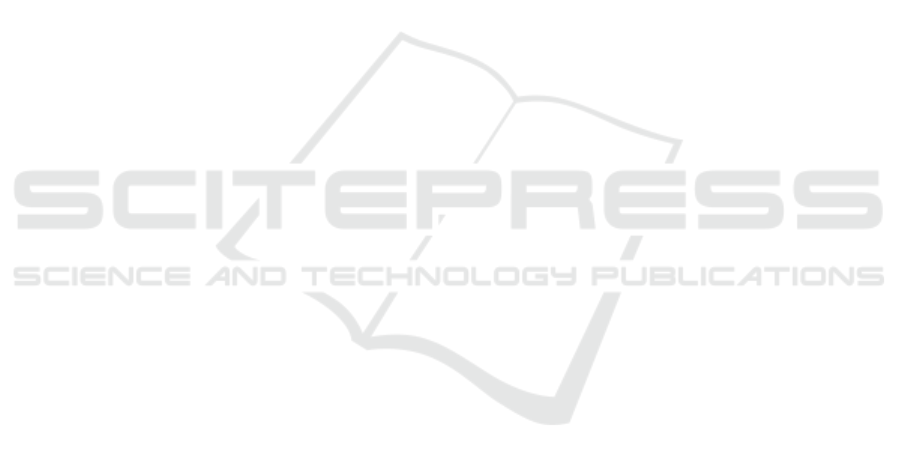
Hasan, M., Choi, J., Neumann, J., Roy-Chowdhury, A. K.,
and Davis, L. S. (2016). Learning temporal regularity
in video sequences. In CVPR, pages 733–742.
Ionescu, R. T., Khan, F. S., Georgescu, M.-I., and Shao,
L. (2019). Object-centric auto-encoders and dummy
anomalies for abnormal event detection in video. In
CVPR, pages 7842–7851.
Kingma, D. P. and Ba, J. (2015). Adam: A method for
stochastic optimization. In ICLR.
Kiran, B. R., Thomas, D. M., and Parakkal, R. (2018).
An overview of deep learning based methods for un-
supervised and semi-supervised anomaly detection in
videos. J Imaging, 4:36.
Li, W., Mahadevan, V., and Vasconcelos, N. (2013).
Anomaly detection and localization in crowded
scenes. IEEE Trans Pattern Anal Mach Intell, 36:18–
32.
Liu, W., Luo, W., Lian, D., and Gao, S. (2018). Future
frame prediction for anomaly detection–a new base-
line. In CVPR, pages 6536–6545.
Liu, X., Zhang, F., Hou, Z., Mian, L., Wang, Z., Zhang, J.,
and Tang, J. (2021a). Self-supervised learning: Gen-
erative or contrastive. IEEE Trans Knowl Data Eng.
Liu, Z., Nie, Y., Long, C., Zhang, Q., and Li, G.
(2021b). A hybrid video anomaly detection frame-
work via memory-augmented flow reconstruction and
flow-guided frame prediction. In ICCV, pages 13588–
13597.
Lohani, D., Crispim-Junior, C., Barth
´
elemy, Q., Bertrand,
S., Robinault, L., and Tougne, L. (2021). Spatio-
temporal convolutional autoencoders for perimeter in-
trusion detection. In RRPR, pages 47–65.
Lu, C., Shi, J., and Jia, J. (2013). Abnormal event detection
at 150 fps in Matlab. In ICCV, pages 2720–2727.
Luo, W., Liu, W., and Gao, S. (2017). A revisit of
sparse coding based anomaly detection in stacked
RNN framework. In ICCV, pages 341–349.
Lv, H., Chen, C., Cui, Z., Xu, C., Li, Y., and Yang, J.
(2021a). Learning normal dynamics in videos with
meta prototype network. In CVPR, pages 15425–
15434.
Lv, H., Zhou, C., Cui, Z., Xu, C., Li, Y., and Yang, J.
(2021b). Localizing anomalies from weakly-labeled
videos. IEEE Trans Image Process, 30:4505–4515.
Menon, V. and Stephen, K. (2021). Re learning memory
guided normality for anomaly detection. In ML Re-
producibility Challenge 2020.
Park, C., Cho, M., Lee, M., and Lee, S. (2022). Fas-
tAno: Fast anomaly detection via spatio-temporal
patch transformation. In WACV, pages 2249–2259.
Park, H., Noh, J., and Ham, B. (2020). Learning memory-
guided normality for anomaly detection. In CVPR,
pages 14372–14381.
Ramachandra, B., Jones, M. J., and Vatsavai, R. R. (2022).
A survey of single-scene video anomaly detection.
IEEE Trans Pattern Anal Mach Intell, 44:2293–2312.
Ristea, N.-C., Madan, N., Ionescu, R. T., Nasrollahi,
K., Khan, F. S., Moeslund, T. B., and Shah, M.
(2022). Self-supervised predictive convolutional at-
tentive block for anomaly detection. In CVPR, pages
13576–13586.
Sinha, P. and Russell, R. (2011). A perceptually based
comparison of image similarity metrics. Perception,
40:1269–1281.
Szymanowicz, S., Charles, J., and Cipolla, R. (2022). Dis-
crete neural representations for explainable anomaly
detection. In WACV, pages 148–156.
Tang, Y., Zhao, L., Zhang, S., Gong, C., Li, G., and Yang, J.
(2020). Integrating prediction and reconstruction for
anomaly detection. Pattern Recognit Lett, 129:123–
130.
Tian, Y., Pang, G., Chen, Y., Singh, R., Verjans, J. W.,
and Carneiro, G. (2021). Weakly-supervised video
anomaly detection with robust temporal feature mag-
nitude learning. In ICCV, pages 4975–4986.
Wang, J., Jiao, J., and Liu, Y.-H. (2020a). Self-supervised
video representation learning by pace prediction. In
ECCV, pages 504–521.
Wang, Z., Zou, Y., and Zhang, Z. (2020b). Cluster attention
contrast for video anomaly detection. In ACM Int Conf
Multimed, pages 2463–2471.
Yao, Y., Liu, C., Luo, D., Zhou, Y., and Ye, Q. (2020). Video
playback rate perception for self-supervised spatio-
temporal representation learning. In CVPR, pages
6548–6557.
Ye, M., Peng, X., Gan, W., Wu, W., and Qiao, Y. (2019).
AnoPCN: Video anomaly detection via deep predic-
tive coding network. In ACM Int Conf Multimed,
pages 1805–1813.
Yu, G., Wang, S., Cai, Z., Zhu, E., Xu, C., Yin, J., and Kloft,
M. (2020). Cloze test helps: Effective video anomaly
detection via learning to complete video events. In
ACM Int Conf Multimed, pages 583–591.
Zhang, R. (2019). Making convolutional networks shift-
invariant again. In ICML, pages 7324–7334.
Zhao, Y., Deng, B., Shen, C., Liu, Y., Lu, H., and Hua,
X.-S. (2017). Spatio-temporal autoencoder for video
anomaly detection. In ACM Int Conf Multimed, pages
1933–1941.
Leveraging Unsupervised and Self-Supervised Learning for Video Anomaly Detection
143