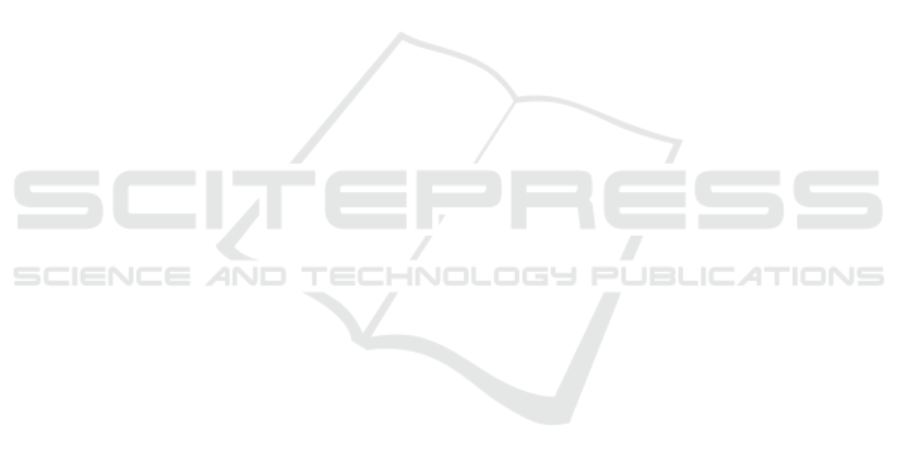
mechanism to select good “sets” of sentences, rather
than just individually relevant sentences. Further-
more, the semantic information gap, arising from the
challenge of incorporating it through non-dense fea-
tures, could be a means of obtaining even more pow-
erful models in the future.
5 CONCLUSIONS
In this work, we present the application of EBM and
GAMI-Net to interpretable extractive summarization,
as a simple but attractive alternative to traditional
classification algorithms. Our results show that, de-
spite more restrictive than full-complexity models in
terms of formulation, GAMs with interactions were
able to achieve similar results to former black-box
models.
Although the need for feature engineering can be
seen as a disadvantage when comparing traditional
approaches to neural models, with a concise set of
features, both EBM and GAMI-Net models showed
promising results for extractive summarization in tex-
tual datasets. The combination of intelligible features
and the transparency of GAMs with interactions can
be a tool to enlighten the view of the extractive sum-
marization decisive process.
We present this paper as a preliminary effort con-
cerning the topic of learning-based interpretable ex-
tractive summarization and believe that the percep-
tions presented into this work could help future re-
search exploring the topic of intelligibility for ATS
systems.
ACKNOWLEDGEMENTS
The authors are grateful to FAPESP grants
#2013/07375-0, #2014/12236-1, #2019/07665-4,
#2019/18287-0, and #2021/05516-1, and CNPq grant
308529/2021-9.
REFERENCES
Afsharizadeh, M., Ebrahimpour-Komleh, H., and Bagheri,
A. (2018). Query-oriented text summarization us-
ing sentence extraction technique. In 2018 4th inter-
national conference on web research (ICWR), pages
128–132. IEEE.
Arrieta, A. B., D
´
ıaz-Rodr
´
ıguez, N., Del Ser, J., Bennetot,
A., Tabik, S., Barbado, A., Garc
´
ıa, S., Gil-L
´
opez, S.,
Molina, D., Benjamins, R., et al. (2020). Explainable
artificial intelligence (xai): Concepts, taxonomies, op-
portunities and challenges toward responsible ai. In-
formation Fusion, 58:82–115.
Bird, S., Klein, E., and Loper, E. (2009). Natural language
processing with Python: analyzing text with the natu-
ral language toolkit. ” O’Reilly Media, Inc.”.
Cohan, A., Dernoncourt, F., Kim, D. S., Bui, T., Kim, S.,
Chang, W., and Goharian, N. (2018). A discourse-
aware attention model for abstractive summarization
of long documents. In Proceedings of the 2018 Con-
ference of the North American Chapter of the Asso-
ciation for Computational Linguistics: Human Lan-
guage Technologies, Volume 2 (Short Papers), pages
615–621.
Danilevsky, M., Qian, K., Aharonov, R., Katsis, Y., Kawas,
B., and Sen, P. (2020). A survey of the state of explain-
able ai for natural language processing. In Proceed-
ings of the 1st Conference of the Asia-Pacific Chap-
ter of the Association for Computational Linguistics
and the 10th International Joint Conference on Natu-
ral Language Processing, pages 447–459.
Do
ˇ
silovi
´
c, F. K., Br
ˇ
ci
´
c, M., and Hlupi
´
c, N. (2018). Ex-
plainable artificial intelligence: A survey. In 2018 41st
International convention on information and commu-
nication technology, electronics and microelectronics
(MIPRO), pages 0210–0215. IEEE.
El-Kassas, W. S., Salama, C. R., Rafea, A. A., and Mo-
hamed, H. K. (2020). Automatic text summarization:
A comprehensive survey. Expert Systems with Appli-
cations, 165:113679.
Ferreira, R., de Souza Cabral, L., Lins, R. D., e Silva, G. P.,
Freitas, F., Cavalcanti, G. D., Lima, R., Simske, S. J.,
and Favaro, L. (2013). Assessing sentence scoring
techniques for extractive text summarization. Expert
systems with applications, 40(14):5755–5764.
Ghodratnama, S., Beheshti, A., Zakershahrak, M., and Sob-
hanmanesh, F. (2020). Extractive document sum-
marization based on dynamic feature space mapping.
IEEE Access, 8:139084–139095.
Hastie, T. and Tibshirani, R. (1987). Generalized additive
models: some applications. Journal of the American
Statistical Association, 82(398):371–386.
Hermann, K. M., Kocisky, T., Grefenstette, E., Espeholt,
L., Kay, W., Suleyman, M., and Blunsom, P. (2015).
Teaching machines to read and comprehend. Ad-
vances in neural information processing systems, 28.
Kedzie, C., Mckeown, K., and Daum
´
e III, H. (2018). Con-
tent selection in deep learning models of summa-
rization. In Proceedings of the 2018 Conference on
Empirical Methods in Natural Language Processing,
pages 1818–1828.
Lin, C.-Y. (2004). Rouge: A package for automatic evalu-
ation of summaries. In Text summarization branches
out, pages 74–81.
Liu, Y. (2019). Fine-tune bert for extractive summarization.
arXiv preprint arXiv:1903.10318.
Lou, Y., Caruana, R., and Gehrke, J. (2012). Intelligible
models for classification and regression. In Proceed-
ings of the 18th ACM SIGKDD international confer-
ence on Knowledge discovery and data mining, pages
150–158.
VISAPP 2023 - 18th International Conference on Computer Vision Theory and Applications
744