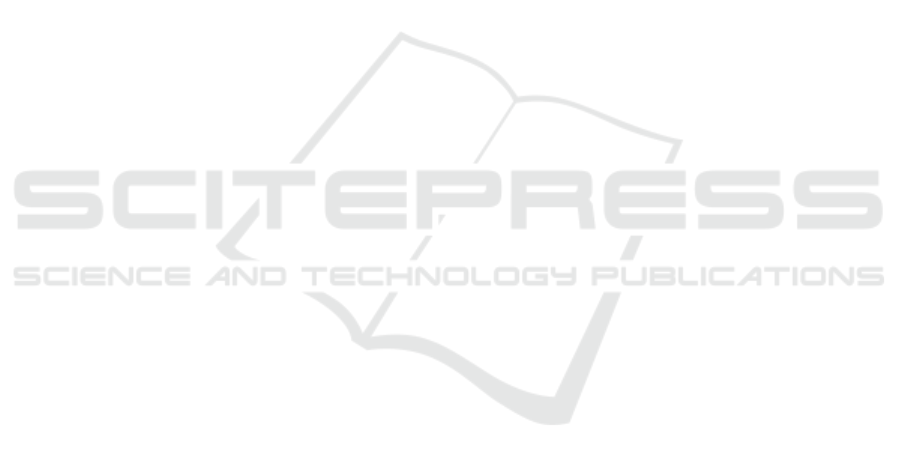
REFERENCES
Andrienko, N. and Andrienko, G. (2006). Exploratory anal-
ysis of spatial and temporal data: a systematic ap-
proach. Springer Science & Business Media.
Anscombe, F. J. (1973). Graphs in statistical analysis. The
American Statistician, 27(1):17–21.
Bartram, L., Correll, M., and Tory, M. (2022). Untidy
data: The unreasonable effectiveness of tables. IEEE
Transactions on Visualization & Computer Graphics,
28(01):686–696.
Dungey, S., Beloff, N., Puri, S., Boggon, R., Williams, T.,
and Tate, A. R. (2014). A pragmatic approach for
measuring data quality in primary care databases. In
IEEE-EMBS International Conference on Biomedical
and Health Informatics (BHI), pages 797–800. IEEE.
Fernstad, S. J. (2019). To identify what is not there: A
definition of missingness patterns and evaluation of
missing value visualization. Information Visualiza-
tion, 18(2):230–250.
Gratzl, S., Lex, A., Gehlenborg, N., Pfister, H., and Streit,
M. (2013). Lineup: Visual analysis of multi-attribute
rankings. IEEE Transactions on Visualization and
Computer Graphics, 19(12):2277–2286.
Gschwandtner, T., Aigner, W., Miksch, S., G
¨
artner, J.,
Kriglstein, S., Pohl, M., and Suchy, N. (2014). Time-
Cleanser: A visual analytics approach for data cleans-
ing of time-oriented data. In Proc. 14th Int. Conf.
Knowledge Technologies and Data-driven Business,
page 18. ACM.
Kandel, S., Parikh, R., Paepcke, A., Hellerstein, J. M., and
Heer, J. (2012). Profiler: Integrated statistical analy-
sis and visualization for data quality assessment. In
Proceedings of the working conference on Advanced
visual interfaces, pages 547–554. ACM.
Laranjeiro, N., Soydemir, S. N., and Bernardino, J. (2015).
A survey on data quality: classifying poor data. In
2015 IEEE 21st Pacific rim international symposium
on dependable computing (PRDC), pages 179–188.
IEEE.
Mackinlay, J. (1986). Automating the design of graphical
presentations of relational information. ACM Trans-
actions on Graphics (TOG), 5(2):110–141.
Maguire, E., Rocca-Serra, P., Sansone, S.-A., Davies, J.,
and Chen, M. (2012). Taxonomy-based glyph de-
sign—with a case study on visualizing workflows of
biological experiments. IEEE Transactions on Visual-
ization and Computer Graphics, 18(12):2603–2612.
Monroe, M., Lan, R., Lee, H., Plaisant, C., and Shneider-
man, B. (2013). Temporal event sequence simplifica-
tion. IEEE Transactions on Visualization and Com-
puter Graphics, 19(12):2227–2236.
Munzner, T. (2014). Visualization analysis and design.
CRC press.
Pham, V. and Dang, T. (2019). Outliagnostics: Visualizing
temporal discrepancy in outlying signatures of data
entries. In 2019 IEEE Visualization in Data Science
(VDS), pages 29–37. IEEE.
Ruddle, R. A., Fateen, W., Treanor, D., Sondergeld, P.,
and Ouirke, P. (2013). Leveraging wall-sized high-
resolution displays for comparative genomics analy-
ses of copy number variation. In 2013 IEEE Sympo-
sium on Biological Data Visualization (BioVis), pages
89–96. IEEE.
Ruddle, R. A. and Hall, M. (2019). Using miniature vi-
sualizations of descriptive statistics to investigate the
quality of electronic health records. In Proceedings of
the 12th International Joint Conference on Biomedi-
cal Engineering Systems and Technologies-Volume 5:
HEALTHINF, pages 230–238. SciTePress.
Shneiderman, B. (2003). The eyes have it: A task by data
type taxonomy for information visualizations. In The
craft of information visualization, pages 364–371. El-
sevier.
Spence, R. (2001). Information visualization, volume 1.
Springer.
Stolte, C., Tang, D., and Hanrahan, P. (2002). Polaris: A
system for query, analysis, and visualization of multi-
dimensional relational databases. IEEE Transactions
on Visualization and Computer Graphics, 8(1):52–65.
Tufte, E. R. (2006). Beautiful evidence. Graphics Press.
Tukey, J. W. (1977). Exploratory data analysis. Addison-
Wesley Series in Behavioral Science: Quantitative
Methods.
Wang, R. Y. and Strong, D. M. (1996). Beyond accuracy:
What data quality means to data consumers. Journal
of management information systems, 12(4):5–33.
Ward, M., Xie, Z., Yang, D., and Rundensteiner, E. (2011).
Quality-aware visual data analysis. Computational
Statistics, 26(4):567–584.
Weiskopf, N. G. and Weng, C. (2013). Methods and di-
mensions of electronic health record data quality as-
sessment: Enabling reuse for clinical research. Jour-
nal of the American Medical Informatics Association,
20(1):144–151.
Wirth, R. and Hipp, J. (2000). CRISP-DM: Towards a stan-
dard process model for data mining. In Proceedings of
the 4th International Conference on the Practical Ap-
plications of Knowledge Discovery and Data mining,
volume 1, pages 29–40. Manchester.
APPENDIX
The table below lists the 79 data quality issues and
number of participants who identified each issue.
The last page combines the data quality taxonomies
from seven previous works: a (ISO/IEC 25012:2008),
b (Dungey et al., 2014), c (Gschwandtner et al., 2014),
d (Kandel et al., 2012), e (Laranjeiro et al., 2015),
f (Wang and Strong, 1996), g (Weiskopf and Weng,
2013). An “*” indicates characteristics that were
identified in the present research but do not appear
in any of those taxonomies.
IVAPP 2023 - 14th International Conference on Information Visualization Theory and Applications
98