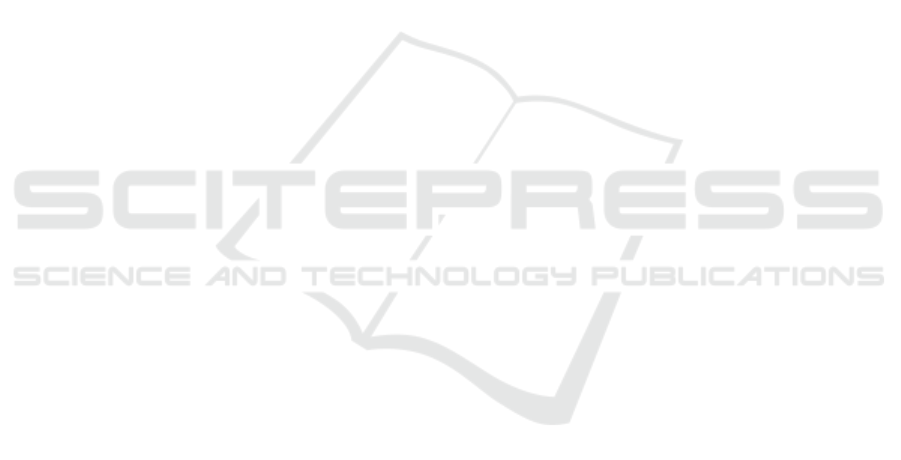
International Conference on Consumer Electronics
(ICCE), pages 145–146. IEEE.
Liang, R., Yan, L., Gao, P., Qian, X., Zhang, Z., and Sun,
H. (2010). Aviation video moving-target detection
with inter-frame difference. In 2010 3rd International
Congress on Image and Signal Processing, volume 3,
pages 1494–1497.
Mahapatra, D., Winkler, S., and Yen, S.-C. (2008). Mo-
tion saliency outweighs other low-level features while
watching videos. In Human Vision and Electronic
Imaging XIII, volume 6806, pages 246–255. SPIE.
Mohtavipour, S. M., Saeidi, M., and Arabsorkhi, A. (2022).
A multi-stream cnn for deep violence detection in
video sequences using handcrafted features. The Vi-
sual Computer, 38(6):2057–2072.
Motlagh, O., Nakhaeinia, D., Tang, S. H., Karasfi, B., and
Khaksar, W. (2014). Automatic navigation of mobile
robots in unknown environments. Neural Computing
and Applications, 24(7):1569–1581.
Nakashima, T. and Yabuta, Y. (2018). Object detection
by using interframe difference algorithm. In 2018
12th France-Japan and 10th Europe-Asia Congress
on Mechatronics, pages 98–102. IEEE.
Narayana, M., Hanson, A., and Learned-Miller, E. (2013).
Coherent motion segmentation in moving camera
videos using optical flow orientations. In Proceed-
ings of the IEEE International Conference on Com-
puter Vision, pages 1577–1584.
Ochs, P., Malik, J., and Brox, T. (2013). Segmentation of
moving objects by long term video analysis. IEEE
transactions on pattern analysis and machine intelli-
gence, 36(6):1187–1200.
Rozantsev, A., Lepetit, V., and Fua, P. (2016). Detecting
flying objects using a single moving camera. IEEE
transactions on pattern analysis and machine intelli-
gence, 39(5):879–892.
Sehairi, K., Chouireb, F., and Meunier, J. (2017). Com-
parative study of motion detection methods for video
surveillance systems. Journal of Electronic Imaging,
26(2):023025.
Sengar, S. S. and Mukhopadhyay, S. (2016). A novel
method for moving object detection based on block
based frame differencing. In 2016 3rd International
Conference on Recent Advances in Information Tech-
nology (RAIT), pages 467–472. IEEE.
Sengar, S. S. and Mukhopadhyay, S. (2017). Foreground
detection via background subtraction and improved
three-frame differencing. Arabian Journal for Science
and Engineering, 42(8):3621–3633.
Siam, M., Mahgoub, H., Zahran, M., Yogamani, S., Jager-
sand, M., and El-Sallab, A. (2018). Modnet: Motion
and appearance based moving object detection net-
work for autonomous driving. In 2018 21st Interna-
tional Conference on Intelligent Transportation Sys-
tems (ITSC), pages 2859–2864.
Simonyan, K. and Zisserman, A. (2014). Very deep con-
volutional networks for large-scale image recognition.
arXiv preprint arXiv:1409.1556.
Stauffer, C. and Grimson, W. E. L. (1999). Adaptive back-
ground mixture models for real-time tracking. In
Proceedings. 1999 IEEE computer society conference
on computer vision and pattern recognition (Cat. No
PR00149), volume 2, pages 246–252. IEEE.
Tezcan, M. O., Ishwar, P., and Konrad, J. (2021). Bsuv-net
2.0: Spatio-temporal data augmentations for video-
agnostic supervised background subtraction. IEEE
Access, 9:53849–53860.
Tsai, C.-M. and Yeh, Z.-M. (2013). Intelligent mov-
ing objects detection via adaptive frame differencing
method. In Asian Conference on Intelligent Informa-
tion and Database Systems, pages 1–11. Springer.
Wang, H., Wang, P., and Qian, X. (2018). Mpnet: An
end-to-end deep neural network for object detection
in surveillance video. IEEE Access, 6:30296–30308.
Wang, Y., Jodoin, P.-M., Porikli, F., Konrad, J., Benezeth,
Y., and Ishwar, P. (2014). Cdnet 2014: An expanded
change detection benchmark dataset. In Proceedings
of the IEEE conference on computer vision and pat-
tern recognition workshops, pages 387–394.
Weng, M., Huang, G., and Da, X. (2010). A new interframe
difference algorithm for moving target detection. In
2010 3rd international congress on image and signal
processing, volume 1, pages 285–289. IEEE.
Williams, E. H., Cristino, F., and Cross, E. S. (2019). Hu-
man body motion captures visual attention and elicits
pupillary dilation. Cognition, 193:104029.
Xiao, J., Cheng, H., Sawhney, H., and Han, F. (2010). Vehi-
cle detection and tracking in wide field-of-view aerial
video. In 2010 IEEE Computer Society Conference
on Computer Vision and Pattern Recognition, pages
679–684. IEEE.
Xu, Z., Zhang, D., and Du, L. (2017). Moving object de-
tection based on improved three frame difference and
background subtraction. In 2017 International Con-
ference on Industrial Informatics-Computing Technol-
ogy, Intelligent Technology, Industrial Information In-
tegration (ICIICII), pages 79–82. IEEE.
Yang, Y., Gong, H., Wang, X., and Sun, P. (2017). Aerial
target tracking algorithm based on faster r-cnn com-
bined with frame differencing. Aerospace, 4(2):32.
Yin, J., Liu, L., Li, H., and Liu, Q. (2016). The infrared
moving object detection and security detection related
algorithms based on w4 and frame difference. Infrared
Physics & Technology, 77:302–315.
Zhang, Y., Kiselewich, S. J., Bauson, W. A., and Ham-
moud, R. (2006). Robust moving object detection at
distance in the visible spectrum and beyond using a
moving camera. In 2006 Conference on Computer Vi-
sion and Pattern Recognition Workshop (CVPRW’06),
pages 131–131. IEEE.
Zhang, Y., Liu, X., Chang, M.-C., Ge, W., and Chen, T.
(2012). Spatio-temporal phrases for activity recogni-
tion. In European Conference on Computer Vision,
pages 707–721. Springer.
Zhao, N., Xia, Y., Xu, C., Shi, X., and Liu, Y. (2016).
Appos: An adaptive partial occlusion segmentation
method for multiple vehicles tracking. Journal of
Visual Communication and Image Representation,
37:25–31.
ICAART 2023 - 15th International Conference on Agents and Artificial Intelligence
318