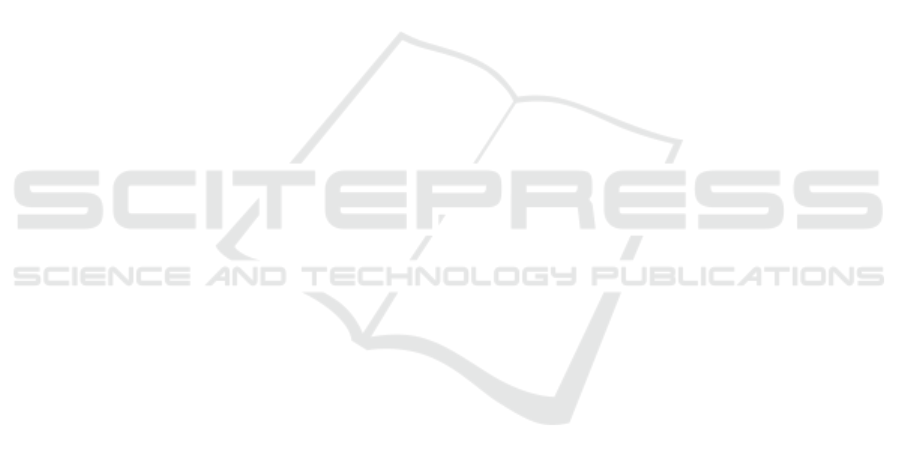
Jiang, H., Wang, J., Yuan, Z., Wu, Y., Zheng, N., and Li, S.
(2013). Salient object detection: A discriminative re-
gional feature integration approach. In Proceedings of
the IEEE conference on computer vision and pattern
recognition, pages 2083–2090.
Jiang, L., Xu, M., Liu, T., Qiao, M., and Wang, Z. (2018).
Deepvs: A deep learning based video saliency predic-
tion approach. In Proceedings of the european confer-
ence on computer vision (eccv), pages 602–617.
Jiang, L., Xu, M., and Wang, Z. (2017). Predicting video
saliency with object-to-motion cnn and two-layer con-
volutional lstm. arXiv preprint arXiv:1709.06316.
Jost, T., Ouerhani, N., Von Wartburg, R., M
¨
uri, R., and
H
¨
ugli, H. (2005). Assessing the contribution of color
in visual attention. Computer Vision and Image Un-
derstanding, 100(1-2):107–123.
Judd, T., Ehinger, K., Durand, F., and Torralba, A. (2009).
Learning to predict where humans look. In 2009
IEEE 12th international conference on computer vi-
sion, pages 2106–2113. IEEE.
Kruthiventi, S. S., Ayush, K., and Babu, R. V. (2017). Deep-
fix: A fully convolutional neural network for predict-
ing human eye fixations. IEEE Transactions on Image
Processing, 26(9):4446–4456.
Lateef, F., Kas, M., and Ruichek, Y. (2021). Saliency heat-
map as visual attention for autonomous driving using
generative adversarial network (gan). IEEE Transac-
tions on Intelligent Transportation Systems.
Le Meur, O., Le Callet, P., Barba, D., and Thoreau, D.
(2006). A coherent computational approach to model
bottom-up visual attention. IEEE transactions on pat-
tern analysis and machine intelligence, 28(5):802–
817.
Leboran, V., Garcia-Diaz, A., Fdez-Vidal, X. R., and Pardo,
X. M. (2016). Dynamic whitening saliency. IEEE
transactions on pattern analysis and machine intelli-
gence, 39(5):893–907.
Leifman, G., Rudoy, D., Swedish, T., Bayro-Corrochano,
E., and Raskar, R. (2017). Learning gaze transitions
from depth to improve video saliency estimation. In
Proceedings of the IEEE International Conference on
Computer Vision, pages 1698–1707.
Li, G., Xie, Y., Wei, T., Wang, K., and Lin, L. (2018).
Flow guided recurrent neural encoder for video salient
object detection. In Proceedings of the IEEE con-
ference on computer vision and pattern recognition,
pages 3243–3252.
Liu, N., Han, J., Liu, T., and Li, X. (2016). Learning to
predict eye fixations via multiresolution convolutional
neural networks. IEEE transactions on neural net-
works and learning systems, 29(2):392–404.
Liu, T., Yuan, Z., Sun, J., Wang, J., Zheng, N., Tang, X.,
and Shum, H.-Y. (2010). Learning to detect a salient
object. IEEE Transactions on Pattern analysis and
machine intelligence, 33(2):353–367.
Mahadevan, V. and Vasconcelos, N. (2009). Spatiotempo-
ral saliency in dynamic scenes. IEEE transactions on
pattern analysis and machine intelligence, 32(1):171–
177.
Marat, S., Ho Phuoc, T., Granjon, L., Guyader, N., Pel-
lerin, D., and Gu
´
erin-Dugu
´
e, A. (2009). Modelling
spatio-temporal saliency to predict gaze direction for
short videos. International journal of computer vision,
82(3):231–243.
Mathe, S. and Sminchisescu, C. (2014). Actions in the eye:
Dynamic gaze datasets and learnt saliency models for
visual recognition. IEEE transactions on pattern anal-
ysis and machine intelligence, 37(7):1408–1424.
Mech, R. and Wollborn, M. (1997). A noise robust
method for segmentation of moving objects in video
sequences. In 1997 IEEE International conference on
acoustics, speech, and signal processing, volume 4,
pages 2657–2660. IEEE.
Mital, P. K., Smith, T. J., Hill, R. L., and Henderson, J. M.
(2011). Clustering of gaze during dynamic scene
viewing is predicted by motion. Cognitive computa-
tion, 3(1):5–24.
Mnih, V., Heess, N., Graves, A., et al. (2014). Recurrent
models of visual attention. Advances in neural infor-
mation processing systems, 27.
Pal, A., Mondal, S., and Christensen, H. I. (2020). ” look-
ing at the right stuff”-guided semantic-gaze for au-
tonomous driving. In Proceedings of the IEEE/CVF
Conference on Computer Vision and Pattern Recogni-
tion, pages 11883–11892.
Pan, J., Sayrol, E., Giro-i Nieto, X., McGuinness, K., and
O’Connor, N. E. (2016). Shallow and deep convolu-
tional networks for saliency prediction. In Proceed-
ings of the IEEE conference on computer vision and
pattern recognition, pages 598–606.
Peters, R. J., Iyer, A., Itti, L., and Koch, C. (2005). Compo-
nents of bottom-up gaze allocation in natural images.
Vision research, 45(18):2397–2416.
Rice, L., Wong, E., and Kolter, Z. (2020). Overfitting in
adversarially robust deep learning. In International
Conference on Machine Learning, pages 8093–8104.
PMLR.
Roberts, R., Ta, D.-N., Straub, J., Ok, K., and Dellaert, F.
(2012). Saliency detection and model-based tracking:
a two part vision system for small robot navigation
in forested environment. In Unmanned Systems Tech-
nology XIV, volume 8387, page 83870S. International
Society for Optics and Photonics.
Rodriguez, M. D., Ahmed, J., and Shah, M. (2008). Action
mach a spatio-temporal maximum average correlation
height filter for action recognition. In 2008 IEEE con-
ference on computer vision and pattern recognition,
pages 1–8. IEEE.
Rudoy, D., Goldman, D. B., Shechtman, E., and Zelnik-
Manor, L. (2013). Learning video saliency from hu-
man gaze using candidate selection. In Proceedings of
the IEEE Conference on Computer Vision and Pattern
Recognition, pages 1147–1154.
Schauerte, B. and Stiefelhagen, R. (2014). “look at this!”
learning to guide visual saliency in human-robot inter-
action. In 2014 IEEE/RSJ International Conference
on Intelligent Robots and Systems, pages 995–1002.
IEEE.
Interactive Video Saliency Prediction: The Stacked-convLSTM Approach
167