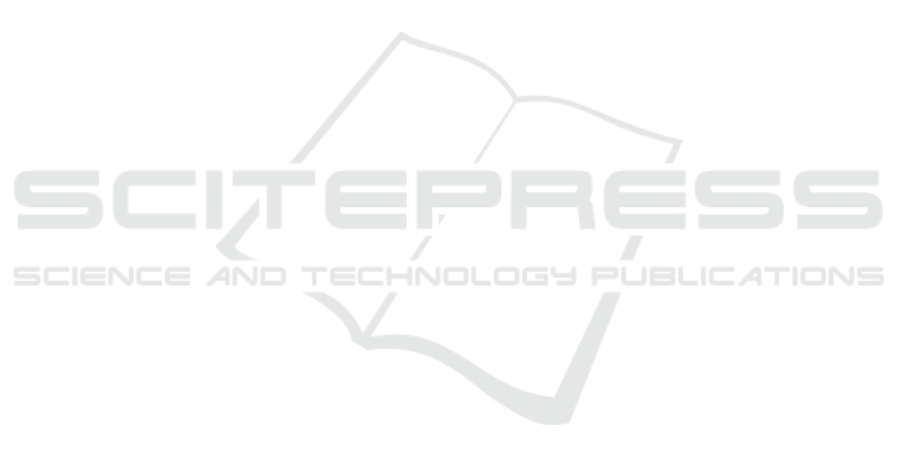
27th ACM SIGKDD Conference on Knowledge Dis-
covery & Data Mining, pages 4123–4124.
Fremont, V. H. J., Frick, J. E.,
˚
Age, L.-J., and Osarenkhoe,
A. (2018). Interaction through boundary objects: con-
troversy and friction within digitalization. Marketing
Intelligence & Planning.
Hardwicke, T. E., Mathur, M. B., MacDonald, K., Nilsonne,
G., Banks, G. C., Kidwell, M. C., Hofelich Mohr,
A., Clayton, E., Yoon, E. J., Henry Tessler, M., et al.
(2018). Data availability, reusability, and analytic re-
producibility: Evaluating the impact of a mandatory
open data policy at the journal cognition. Royal Soci-
ety open science, 5(8):180448.
Hey, A. J., Tansley, S., Tolle, K. M., et al. (2009). The
fourth paradigm: data-intensive scientific discovery,
volume 1. Microsoft research Redmond, WA.
Hossain, M. M., Roy, B., Roy, C. K., and Schneider, K. A.
(2020). Vizsciflow: A visually guided scripting frame-
work for supporting complex scientific data analysis.
Proceedings of the ACM on Human-Computer Inter-
action, 4(EICS):1–37.
Hunter-Zinck, H., de Siqueira, A. F., V
´
asquez, V. N.,
Barnes, R., and Martinez, C. C. (2021). Ten sim-
ple rules on writing clean and reliable open-source
scientific software. PLoS computational biology,
17(11):e1009481–e1009481.
Hwang, T. and Yu, H.-Y. (2011). Accommodating both
expert users and novice users in one interface by uti-
lizing multi-layer interface in complex function prod-
ucts. In International Conference on International-
ization, Design and Global Development, pages 159–
165. Springer.
Iqbal Chunpir, H., Rathmann, T., and Zaina, L. M. (2018).
An empirical evidence of barriers in a big data infras-
tructure. Interacting with Computers, 30(6):507–523.
Kalakoski, V., Henelius, A., Oikarinen, E., Ukkonen, A.,
and Puolam
¨
aki, K. (2019). Cognitive ergonomics for
data analysis. Experimental study of cognitive limita-
tions in a data-based judgement task. Behaviour &
Information Technology, 38(10):1038–1047.
Kim, C.-W., Yoon, H., Jin, D., and Park, S. O. (2016). Inte-
grated management system for a large computing re-
sources in a scientific data center. The Journal of Su-
percomputing, 72(9):3511–3521.
Kogan, M., Halfaker, A., Guha, S., Aragon, C., Muller, M.,
and Geiger, S. (2020). Mapping out human-centered
data science: Methods, approaches, and best prac-
tices. In Companion of the 2020 ACM International
Conference on Supporting Group Work, pages 151–
156.
Lacroix, Z. and Critchlow, T. (2003). Compared evaluation
of scientific data management systems. In Bioinfor-
matics, pages 371–391. Elsevier.
Levin, N. and Leonelli, S. (2017). How does one “open”
science? questions of value in biological research.
Science, Technology, & Human Values, 42(2):280–
305.
Lin, Y.-W., Bates, J., and Goodale, P. (2016). Co-observing
the weather, co-predicting the climate: Human factors
in building infrastructures for crowdsourced data. Sci-
ence and Technology Studies, 29(3):10–27.
Liu, J., Pacitti, E., Valduriez, P., and Mattoso, M. (2015). A
survey of data-intensive scientific workflow manage-
ment. Journal of Grid Computing, 13(4):457–493.
Macaulay, C., Sloan, D., Jiang, X., Forbes, P., Loynton,
S., Swedlow, J. R., and Gregor, P. (2009). Usability
and user-centered design in scientific software devel-
opment. IEEE software, 26(1):96.
Machado Paix
˜
ao-Cortes, V. S., da Silva Tanus, M. d. S.,
Paix
˜
ao-Cortes, W. R., de Souza, O. N., de Borba Cam-
pos, M., and Silveira, M. S. (2018). Usability as the
key factor to the design of a web server for the cref
protein structure predictor: The wcref. Information,
9(1):20.
Michener, W. K., Allard, S., Budden, A., Cook, R. B.,
Douglass, K., Frame, M., Kelling, S., Koskela, R.,
Tenopir, C., and Vieglais, D. A. (2012). Participa-
tory design of dataone—enabling cyberinfrastructure
for the biological and environmental sciences. Eco-
logical Informatics, 11:5–15.
Mortier, R., Haddadi, H., Henderson, T., McAuley, D.,
and Crowcroft, J. (2013). Challenges & opportunities
in human-data interaction. University of Cambridge,
Computer Laboratory.
N
´
eron, B., M
´
enager, H., Maufrais, C., Joly, N., Maupetit,
J., Letort, S., Carrere, S., Tuffery, P., and Letondal,
C. (2009). Mobyle: a new full web bioinformatics
framework. Bioinformatics, 25(22):3005–3011.
Newman, G. J. (2010). Designing and evaluating participa-
tory cyber-infrastructure systems for multi-scale citi-
zen science. PhD thesis, Colorado State University.
Oliver, H., Diallo, G., De Quincey, E., Alexopoulou, D.,
Habermann, B., Kostkova, P., Schroeder, M., Jupp,
S., Khelif, K., Stevens, R., et al. (2009). A user-
centred evaluation framework for the sealife semantic
web browsers. BMC bioinformatics, 10(10):1–15.
Overmyer, T. (2019). UX methods in the data lab: arguing
for validity. In Proceedings of the 37th ACM Inter-
national Conference on the design of communication,
SIGDOC ’19, pages 1–6. ACM.
Parsons, M. and Duerr, R. (2005). Designating user com-
munities for scientific data: challenges and solutions.
Data Science Journal, 4:31–38.
Patterson, R. E., Blaha, L. M., Grinstein, G. G., Liggett,
K. K., Kaveney, D. E., Sheldon, K. C., Havig, P. R.,
and Moore, J. A. (2014). A human cognition frame-
work for information visualization. Computers &
Graphics, 42:42–58.
P
´
erez, F. and Granger, B. E. (2007). Ipython: a system for
interactive scientific computing. Computing in science
& engineering, 9(3):21–29.
Perez, F. and Granger, B. E. (2015). Project jupyter: Com-
putational narratives as the engine of collaborative
data science. Retrieved September, 11(207):108.
Perry, M. (2003). Distributed cognition. HCI models, theo-
ries, and frameworks: Toward a multidisciplinary sci-
ence, pages 193–223.
Poole, A. H. (2015). How has your science data grown?
Usability Assessment in Scientific Data Analysis: A Literature Review
71