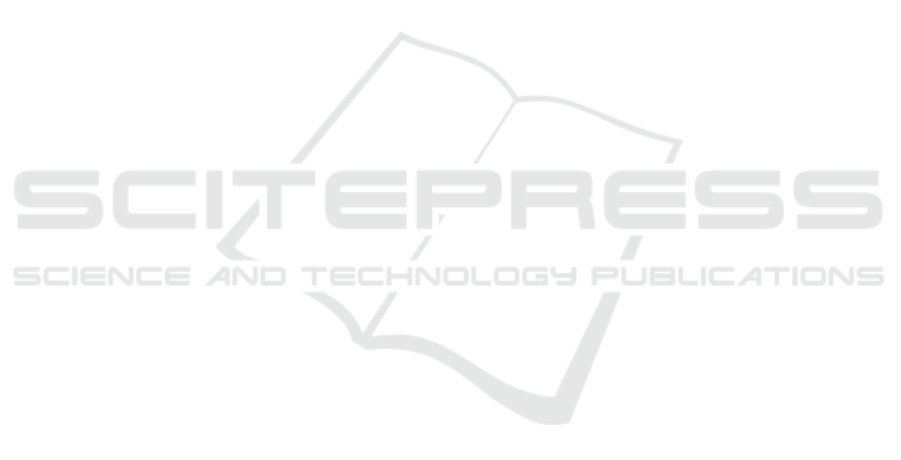
ACKNOWLEDGEMENTS
This research was supported by Health Labour Sci-
ences Research Grant 21AC1001.
REFERENCES
Aziz, M. M. A., Sadat, M. N., Alhadidi, D., Wang, S.,
Jiang, X., Brown, C. L., and Mohammed, N. (2019).
Privacy-preserving techniques of genomic data-a sur-
vey. Brief Bioinform., 20(3):887–895.
Cummings, R. and Desai, D. (2018). The role of differential
privacy in GDPR compliance.
Domingo-Ferrer, J. and Soria-Comas, J. (2015). From t-
closeness to differential privacy and vice versa in data
anonymization. Knowl.-Based Sys., 74:151–158.
Dwork, C. (2006). Differential privacy. In Bugliesi, M.,
Preneel, B., Sassone, V., and Wegener, I., editors, Au-
tomata, Languages and Programming, pages 1–12,
Berlin, Heidelberg. Springer Berlin Heidelberg.
Dwork, C., McSherry, F., Nissim, K., and Smith, A. (2006).
Calibrating noise to sensitivity in private data anal-
ysis. In Halevi, S. and Rabin, T., editors, Theory
of Cryptography, pages 265–284, Berlin, Heidelberg.
Springer Berlin Heidelberg.
Dwork, C. and Roth, A. (2014). The algorithmic foun-
dations of differential privacy. Found. Trends Theor.
Comput. Sci., 9(3–4):211–407.
Emam, K. E. and Dankar, F. K. (2008). Protecting pri-
vacy using k-anonymity. J. Am. Med. Inform. Assoc.,
15(5):627–637.
Erlingsson, U., Pihur, V., and Korolova, A. (2014). RAP-
POR: Randomized aggregatable privacy-preserving
ordinal response. In Proceedings of the 2014 ACM
SIGSAC Conference on Computer and Communica-
tions Security, CCS ’14, page 1054–1067, New York,
NY, USA. Association for Computing Machinery.
European Commission (2016). Regulation (EU) 2016/679
of the European Parliament and of the Council of 27
April 2016 on the protection of natural persons with
regard to the processing of personal data and on the
free movement of such data, and repealing Directive
95/46/EC (General Data Protection Regulation), OJ
2016 L 119/1.
Fanti, G., Pihur, V., and Erlingsson, U. (2016). Build-
ing a RAPPOR with the unknown: Privacy-
preserving learning of associations and data dictionar-
ies. Proceedings on Privacy Enhancing Technologies
(PoPETS), issue 3, 2016.
Ficek, J., Wang, W., Chen, H., Dagne, G., and Daley, E.
(2021). Differential privacy in health research: A
scoping review. Journal of the American Medical In-
formatics Association, 28(10):2269–2276.
Gaboardi, M. and Rogers, R. (2018). Local private hy-
pothesis testing: Chi-square tests. In Dy, J. and
Krause, A., editors, Proceedings of the 35th Interna-
tional Conference on Machine Learning, volume 80
of Proceedings of Machine Learning Research, pages
1626–1635. PMLR.
Hl
´
avka, J. P. (2020). Chapter 10 - security, privacy, and
information-sharing aspects of healthcare artificial in-
telligence. In Bohr, A. and Memarzadeh, K., editors,
Artificial Intelligence in Healthcare, pages 235–270.
Academic Press.
Holohan, N., Antonatos, S., Braghin, S., and Aonghusa,
P. M. (2017). (k,ε)-anonymity: k-anonymity with ε-
differential privacy. arXiv: Cryptography and Secu-
rity.
Kullback, S. and Leibler, R. A. (1951). On information and
sufficiency. Ann. Math. Statist., 22(1):79–86.
Lee, H., Kim, S., Kim, J. W., and Chung, Y. D. (2017).
Utility-preserving anonymization for health data pub-
lishing. BMC Med. Inform. Decis. Mak., 17:104.
Li, N., Qardaji, W., and Su, D. (2012). On sam-
pling, anonymization, and differential privacy or, k-
anonymization meets differential privacy. In Proceed-
ings of the 7th ACM Symposium on Information, Com-
puter and Communications Security, ASIACCS ’12,
page 32–33, New York, NY, USA. Association for
Computing Machinery.
Meng, X., Li, H., and Cui, J. (2017). Different strategies for
differentially private histogram publication. J. Com-
mun. Inf. Netw., 2:68–77.
Rakesh Kumar, S., Gayathri, N., Muthuramalingam, S.,
Balamurugan, B., Ramesh, C., and Nallakaruppan, M.
(2019). Chapter 13 - medical big data mining and pro-
cessing in e-healthcare. In Balas, V. E., Son, L. H.,
Jha, S., Khari, M., and Kumar, R., editors, Internet
of Things in Biomedical Engineering, pages 323–339.
Academic Press.
Su, J., Cao, Y., Chen, Y., Liu, Y., and Song, J. (2021).
Privacy protection of medical data in social network.
BMC Med. Inform. Decis. Mak., 21:286.
Sweeney, L. (2002). K-anonymity: A model for protect-
ing privacy. Int. J. Uncertain. Fuzziness Knowl.-Based
Syst., 10(5):557–570.
Tsou, Y.-T., Alraja, M. N., Chen, L.-S., Chang, Y.-H., Hu,
Y.-L., Huang, Y., Yu, C.-M., and Tsai, P.-Y. (2021).
(k, ε, δ)-anonymization: Privacy-preserving data re-
lease based on k-anonymity and differential privacy.
Serv. Oriented Comput. Appl., 15(3):175–185.
Wang, Y., Wu, X., and Hu, D. (2016). Using random-
ized response for differential privacy preserving data
collection. In Palpanas, T. and Stefanidis, K., edi-
tors, Proceedings of the Workshops of the EDBT/ICDT
2016 Joint Conference, EDBT/ICDT Workshops 2016,
Bordeaux, France, March 15, 2016, volume 1558 of
CEUR Workshop Proceedings.
Warner, S. L. (1965). Randomized response: A survey tech-
nique for eliminating evasive answer bias. Journal of
the American Statistical Association, 60(309):63–69.
Wu, W.-T., Li, Y.-J., Feng, A.-Z., Li, L., Huang, T., Xu,
A.-D., and Lyu, J. (2021). Data mining in clinical
big data: the frequently used databases, steps, and
methodological models. Military Med. Res., 8:44.
Ye, Y., Zhang, M., Feng, D., Li, H., and Chi, J. (2019). Mul-
tiple privacy regimes mechanism for local differential
HEALTHINF 2023 - 16th International Conference on Health Informatics
296