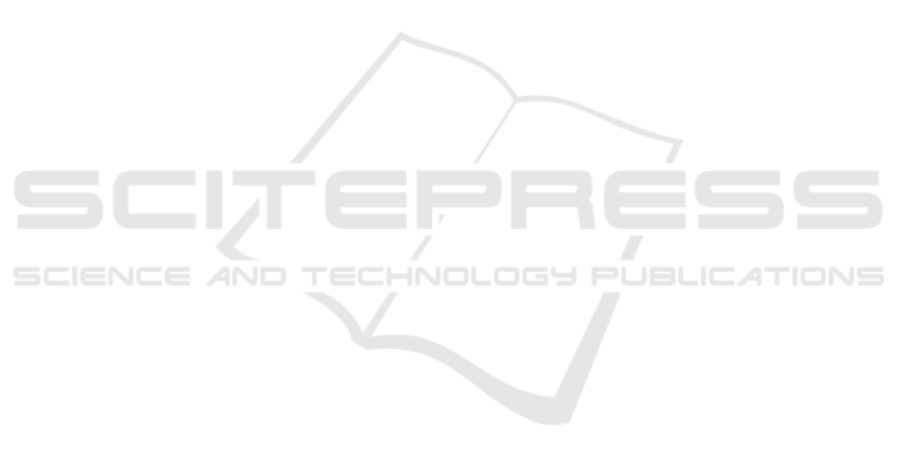
method of atrial fibrillation classes with high order
statistics and classification using machine learning. In
BIOSIGNALS.
de Albuquerque, V. H. C., Nunes, T. M., Pereira, D. R.,
da S. Luz, E. J., Menotti, D., Papa, J. P., and Tavares,
J. M. R. S. (2018). Robust automated cardiac arrhyth-
mia detection in ecg beat signals. Neural Comput &
Applic, 29:679–693.
Fuadah, Y. N. and Lim, K. M. (2022). Optimal classification
of atrial fibrillation and congestive heart failure using
machine learning. Frontiers in Physiology, 12.
Ganapathy, N., Baumg
¨
artel, D., and Deserno, T. M. (2021).
Automatic detection of atrial fibrillation in ecg us-
ing co-occurrence patterns of dynamic symbol assign-
ment and machine learning. Sensors, 21(10).
Goldberger, A., Amaral, L., Glass, L., JM, H., Ivanov,
P., Mark, R., Mietus, J., Moody, G., Peng, C.,
and Stanley, H. (2000). Physiobank, physiotoolkit,
and physionet: components of a new research re-
source for complex physiologic signals. Circulation.,
101(23):E215–20.
Hassan, S. U., Zahid, M. S. M., Abdullah, T. A., and
Husain, K. (2022). Classification of cardiac ar-
rhythmia using a convolutional neural network and
bi-directional long short-term memory. DIGITAL
HEALTH, 8:20552076221102766.
Horoba, K., Czabanski, R., Wrobel, J., Matonia, A., Mar-
tinek, R., Kupka, T., Kahankova, R., Leski, J. M., and
Graczyk, S. (2019). Recognition of atrial fibrilation
episodes in heart rate variability signals using a ma-
chine learning approach. In 2019 MIXDES - 26th In-
ternational Conference ”Mixed Design of Integrated
Circuits and Systems”, pages 419–424.
Humphreys, M., Warlow, C., and McGowan, J. (2011). Ar-
rhythmias and their Management, chapter 10, pages
132–155. John Wiley & Sons, Ltd.
Irfan, S., Anjum, N., Althobaiti, T., Alotaibi, A. A., Sid-
diqui, A. B., and Ramzan, N. (2022). Heartbeat classi-
fication and arrhythmia detection using a multi-model
deep-learning technique. Sensors, 22(15).
Kuila, S., Dhanda, N., and Joardar, S. (2020). Fea-
ture extraction of electrocardiogram signal using ma-
chine learning classification. International Journal
of Electrical and Computer Engineering (IJECE),
10(6):6598–6605.
Mkoko, P., Bahiru, E., Ajijola, O. A., Bonny, A., and
Chin, A. (2020). Cardiac arrhythmias in low- and
middle-income countries. Cardiovasc Diagn Ther.,
10(2):350–360.
Mohebbanaaz, Kumari, L. V. R., and Sai, Y. P. (2022). Clas-
sification of ecg beats using optimized decision tree
and adaptive boosted optimized decision tree. Signal,
Image and Video Processing, 16:695–703.
Mohebbanaaz, Sai, Y. P., and kumari, L. R. (2020). A
review on arrhythmia classification using ecg sig-
nals. In 2020 IEEE International Students’ Confer-
ence on Electrical,Electronics and Computer Science
(SCEECS), pages 1–6.
Moody, G. B. and Mark, R. G. (2001). The impact of the
mit-bih arrhythmia database. IEEE Engineering in
Medicine and Biology Magazine., 20(3):45–50.
Mustaqeem, A., Anwar, S. M., and Majid, M. (2018). Mul-
ticlass classification of cardiac arrhythmia using im-
proved feature selection and svm invariants. Com-
putational and Mathematical Methods in Medicine,
2018:7310496.
Ramesh, J., Solatidehkordi, Z., Aburukba, R., and Sagahy-
roon, A. (2021). Atrial fibrillation classification with
smart wearables using short-term heart rate variabil-
ity and deep convolutional neural networks. Sensors,
21(21).
Sager, S., Bernhardt, F., Kehrle, F., Merkert, M., Potschka,
A., Meder, B., Katus, H., and Scholz, E. (2021).
Expert-enhanced machine learning for cardiac ar-
rhythmia classification. PLoS One, 16(12):e0261571.
Shalini, B., Nandini, V., Sandhya, M., and R, B. (2019).
Prediction and classification of cardiac arrhythmia.
International Research Journal of Engineering and
Technology (IRJET), 6(6):572–576.
Singh, V., Tewary, S., Sardana, V., and Sardana, H. K.
(2019). Arrhythmia detection - a machine learning
based comparative analysis with mit-bih ecg data. In
2019 IEEE 5th International Conference for Conver-
gence in Technology (I2CT), pages 1–5.
WHO (2021). Cardiovascular diseases (cvds). fact sheets.
Available online. https://www.who.int/en/news-
room/fact-sheets/detail/cardiovascular-diseases-
(cvds). Accessed Oct/2022.
Zhang, J., Liu, A., Gao, M., Chen, X., Zhang, X., and
Chen, X. (2020). Ecg-based multi-class arrhythmia
detection using spatio-temporal attention-based con-
volutional recurrent neural network. Artificial Intelli-
gence in Medicine, 106:101856.
Arrythmia Classification Using MATLAB
R
Classification Learner App
225