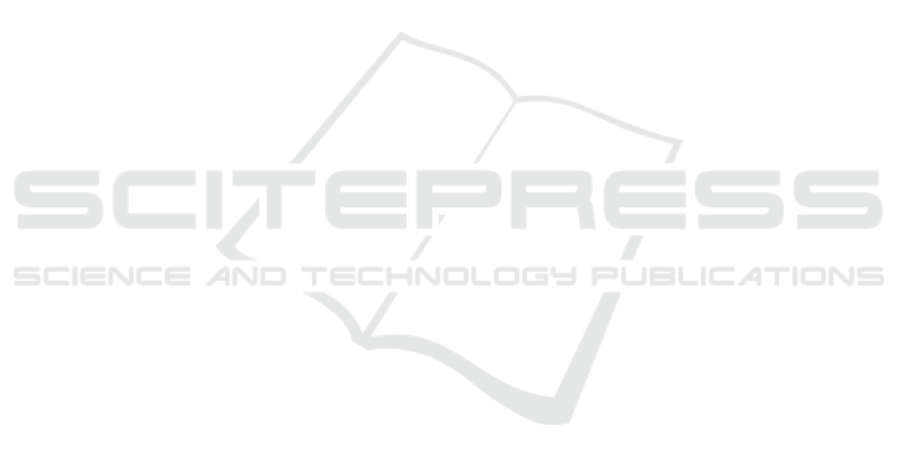
Jena supported in part by DFG grants INST 275/334-
1 FUGG and INST 275/363-1 FUGG.
REFERENCES
Amara, J., Bouaziz, B., Algergawy, A., et al. (2017). A deep
learning-based approach for banana leaf diseases clas-
sification. In BTW (workshops), volume 266, pages
79–88.
Ballester, P., Correa, U. B., Birck, M., and Araujo, R.
(2017). Assessing the performance of convolutional
neural networks on classifying disorders in apple tree
leaves. In Latin American Workshop on Computa-
tional Neuroscience, pages 31–38. Springer.
Brahimi, M., Arsenovic, M., Laraba, S., Sladojevic, S.,
Boukhalfa, K., and Moussaoui, A. (2018). Deep learn-
ing for plant diseases: detection and saliency map vi-
sualisation. In Human and machine learning, pages
93–117. Springer.
Brahimi, M., Mahmoudi, S., Boukhalfa, K., and Mous-
saoui, A. (2019). Deep interpretable architecture for
plant diseases classification. In 2019 Signal Process-
ing: Algorithms, Architectures, Arrangements, and
Applications (SPA), pages 111–116. IEEE.
Britannica, T. (2020). Editors of encyclopaedia. Argon.
Encyclopedia Britannica.
Chollet, F. (2021). Deep learning with Python. Simon and
Schuster.
Cimpoi, M., Maji, S., Kokkinos, I., Mohamed, S., and
Vedaldi, A. (2014). Describing textures in the wild.
In Proceedings of the IEEE conference on computer
vision and pattern recognition, pages 3606–3613.
Davis, J. and Goadrich, M. (2006). The relationship be-
tween precision-recall and roc curves. In Proceed-
ings of the 23rd international conference on Machine
learning, pages 233–240.
Deng, J., Dong, W., Socher, R., Li, L.-J., Li, K., and Fei-
Fei, L. (2009). Imagenet: A large-scale hierarchical
image database. In 2009 IEEE conference on com-
puter vision and pattern recognition, pages 248–255.
Ieee.
Doshi-Velez, F. and Kim, B. (2017). Towards a rigorous sci-
ence of interpretable machine learning. arXiv preprint
arXiv:1702.08608.
Ghorbani, A., Abid, A., and Zou, J. (2019). Interpretation
of neural networks is fragile. In Proceedings of the
AAAI conference on artificial intelligence, volume 33,
pages 3681–3688.
Ghosal, S., Blystone, D., Singh, A. K., Ganapathysubrama-
nian, B., Singh, A., and Sarkar, S. (2018). An explain-
able deep machine vision framework for plant stress
phenotyping. Proceedings of the National Academy
of Sciences, 115(18):4613–4618.
Hughes, D., Salath
´
e, M., et al. (2015). An open access
repository of images on plant health to enable the
development of mobile disease diagnostics. arXiv
preprint arXiv:1511.08060.
Isleib, J. (2012). Signs and symptoms of plant disease: Is
it fungal, viral or bacterial. Michigan State University
Extension.
Kim, B., Wattenberg, M., Gilmer, J., Cai, C., Wexler, J.,
Viegas, F., et al. (2018). Interpretability beyond fea-
ture attribution: Quantitative testing with concept ac-
tivation vectors (tcav). In International conference on
machine learning, pages 2668–2677. PMLR.
Kindermans, P.-J., Hooker, S., Adebayo, J., Alber, M.,
Sch
¨
utt, K. T., D
¨
ahne, S., Erhan, D., and Kim, B.
(2019). The (un) reliability of saliency methods. In
Explainable AI: Interpreting, Explaining and Visual-
izing Deep Learning, pages 267–280. Springer.
Kinger, S. and Kulkarni, V. (2021). Explainable ai for deep
learning based disease detection. In 2021 Thirteenth
International Conference on Contemporary Comput-
ing (IC3-2021), pages 209–216.
Lee, S. H., Go
¨
eau, H., Bonnet, P., and Joly, A. (2020). New
perspectives on plant disease characterization based
on deep learning. Computers and Electronics in Agri-
culture, 170:105220.
Lucieri, A., Bajwa, M. N., Braun, S. A., Malik, M. I., Den-
gel, A., and Ahmed, S. (2020). On interpretability of
deep learning based skin lesion classifiers using con-
cept activation vectors. In 2020 international joint
conference on neural networks (IJCNN), pages 1–10.
IEEE.
Molnar, C. (2020). Interpretable machine learning. Lulu.
com.
Sladojevic, S., Arsenovic, M., Anderla, A., Culibrk, D., and
Stefanovic, D. (2016). Deep neural networks based
recognition of plant diseases by leaf image classifi-
cation. Computational intelligence and neuroscience,
2016.
Szegedy, C., Liu, W., Jia, Y., Sermanet, P., Reed, S.,
Anguelov, D., Erhan, D., Vanhoucke, V., and Rabi-
novich, A. (2015). Going deeper with convolutions.
In Proceedings of the IEEE conference on computer
vision and pattern recognition, pages 1–9.
Szegedy, C., Vanhoucke, V., Ioffe, S., Shlens, J., and Wo-
jna, Z. (2016). Rethinking the inception architecture
for computer vision. In Proceedings of the IEEE con-
ference on computer vision and pattern recognition,
pages 2818–2826.
Toda, Y. and Okura, F. (2019). How convolutional neural
networks diagnose plant disease. Plant Phenomics,
2019.
Weiss, K., Khoshgoftaar, T. M., and Wang, D. (2016).
A survey of transfer learning. Journal of Big data,
3(1):1–40.
Zeiler, M. D. and Fergus, R. (2014). Visualizing and under-
standing convolutional networks. In European confer-
ence on computer vision, pages 818–833. Springer.
Zhou, B., Sun, Y., Bau, D., and Torralba, A. (2018). Inter-
pretable basis decomposition for visual explanation.
In Proceedings of the European Conference on Com-
puter Vision (ECCV), pages 119–134.
Concept Explainability for Plant Diseases Classification
253