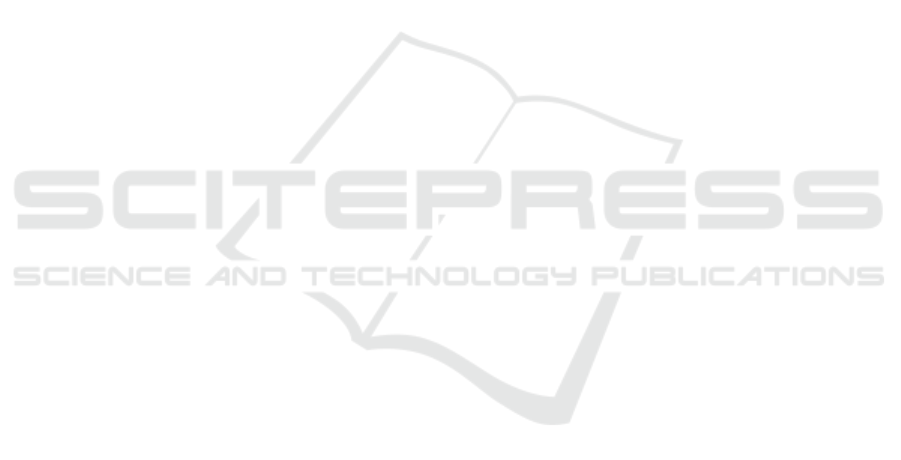
ACKNOWLEDGEMENTS
This work was supported in part by the JSPS Grant-
in-Aid for Scientific Research (17H00745).
REFERENCES
Carreira, J. and Zisserman, A. (2017). Quo Vadis, action
recognition? a new model and the kinetics dataset. In
Proceedings of the 2017 IEEE Conference on Com-
puter Vision and Pattern Recognition, pages 4724–
4733, Honolulu, HI. IEEE.
Gunnar, B. (1982). Psychophysical bases of perceived ex-
ertion. Medicine & Science in Sports & Exercise,
14(5):377–381.
Hyung, E.-J., Lee, H.-O., and Kwon, Y.-J. (2016). Influ-
ence of load and carrying method on gait, specifically
pelvic movement. Journal of Physical Therapy Sci-
ence, 28(7):2059–2062.
Kato, H., Hirayama, T., Kawanishi, Y., Doman, K., Ide, I.,
Deguchi, D., and Murase, H. (2017). Toward describ-
ing human gaits by onomatopoeias. In Proceedings of
the 16th IEEE International Conference on Computer
Vision Workshops, pages 1573–1580. IEEE.
Liu, J., Shahroudy, A., Xu, D., and Wang, G. (2016).
Spatio-temporal LSTM with trust gates for 3D human
action recognition. In Leibe, B., Matas, J., Sebe, N.,
and Welling, M., editors, Computer Vision – ECCV
2016, volume 9907, pages 816–833. Springer Interna-
tional Publishing.
Liu, J., Wang, G., Hu, P., Duan, L.-Y., and Kot, A. C.
(2017). Global context-aware attention LSTM net-
works for 3D action recognition. In Proceedings of
the 2017 IEEE Conference on Computer Vision and
Pattern Recognition, pages 3671–3680, Honolulu, HI.
IEEE.
Liu, Z., Zhang, H., Chen, Z., Wang, Z., and Ouyang, W.
(2020). Disentangling and unifying graph convolu-
tions for skeleton-based action recognition. In Pro-
ceedings of the 2020 IEEE/CVF Conference on Com-
puter Vision and Pattern Recognition, pages 140–149,
Seattle, WA, USA. IEEE.
Maas, A. L., Hannun, A. Y., and Ng, A. Y. (2013). Rectifier
nonlinearities improve neural network acoustic mod-
els. In Proceedings of the 30th International Confer-
ence on Machine Learning Workshop, pages 1–6.
Majd, M. and Safabakhsh, R. (2020). Correlational convo-
lutional LSTM for human action recognition. Neuro-
computing, 396:224–229.
Nishida, N., Kawanishi, Y., Deguchi, D., Ide, I., Murase,
H., and Piao, J. (2020). SOANets: Encoder-Decoder
based skeleton orientation alignment network for
white cane user recognition from 2D human skeleton
sequence. In Proceedings of the 15th International
Joint Conference on Computer Vision, Imaging and
Computer Graphics Theory and Applications, pages
435–443, Valletta, Malta. SCITEPRESS - Science and
Technology Publications.
Oji, T., Makino, Y., and Shinoda, H. (2018). Weight esti-
mation of lifted object from body motions using neu-
ral network. In Prattichizzo, D., Shinoda, H., Tan,
H. Z., Ruffaldi, E., and Frisoli, A., editors, Proceed-
ings of the Haptics: Science, Technology, and Appli-
cations, volume 10894, pages 3–13. Springer Interna-
tional Publishing, Cham.
Teepe, T., Khan, A., Gilg, J., Herzog, F., H
¨
ormann, S., and
Rigoll, G. (2021). GaitGraph: Graph convolutional
network for skeleton-based gait recognition. In Pro-
ceedings of the 2021 IEEE International Conference
on Image Processing, pages 2314–2318.
Temuroglu, O., Kawanishi, Y., Deguchi, D., Hirayama, T.,
Ide, I., Murase, H., Iwasaki, M., and Tsukada, A.
(2020). Occlusion-aware skeleton trajectory represen-
tation for abnormal behavior detection. In Ohyama,
W. and Jung, S. K., editors, Proceedings of the
2020 International Workshop on Frontiers of Com-
puter Vision, volume 1212, pages 108–121, Singa-
pore. Springer Singapore.
Ullah, A., Ahmad, J., Muhammad, K., Sajjad, M., and Baik,
S. W. (2018). Action recognition in video sequences
using deep bi-directional LSTM with CNN features.
IEEE Access, 6:1155–1166.
Yamaguchi, Y., Kamitani, T., Nishiyama, M., Iwai, Y., and
Kushida, D. (2020). Extracting features of body sway
for baggage weight classification. In Proceedings of
the 9th IEEE Global Conference on Consumer Elec-
tronics, pages 345–348. IEEE.
Yamamoto, T., Terada, K., Ochiai, A., Saito, F., Asahara,
Y., and Murase, K. (2019). Development of human
support robot as the research platform of a domestic
mobile manipulator. ROBOMECH Journal, 6(1):1–
15.
Yan, S., Xiong, Y., and Lin, D. (2018). Spatial temporal
graph convolutional networks for skeleton-based ac-
tion recognition. In Proceedings of the 32nd AAAI
Conference on Artificial Intelligence, pages 7444–
7452.
Yuguchi, A., Kawano, S., Yoshino, K., Ishi, C. T., Kawan-
ishi, Y., Nakamura, Y., Minato, T., Saito, Y., and Mi-
noh, M. (2022). Butsukusa: A conversational mo-
bile robot describing its own observations and internal
states. In Proceedings of the 2022 17th ACM/IEEE In-
ternational Conference on Human-Robot Interaction,
pages 1114–1118, Sapporo, Japan. IEEE.
VISAPP 2023 - 18th International Conference on Computer Vision Theory and Applications
574