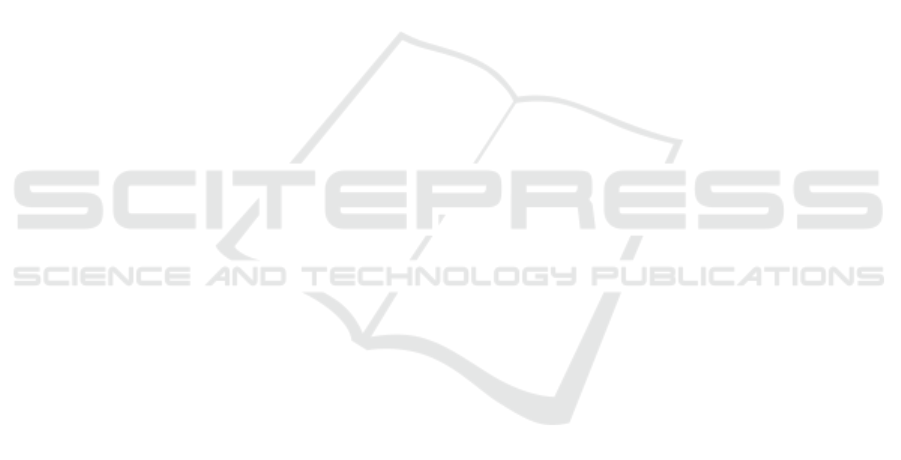
on simulation and synthesis in medical imaging, pages
31–41. Springer.
Hiasa, Y., Otake, Y., Takao, M., Matsuoka, T., Takashima,
K., Carass, A., Prince, J. L., Sugano, N., and Sato,
Y. (2018b). Cross-modality image synthesis from un-
paired data using cyclegan. In Gooya, A., Goksel,
O., Oguz, I., and Burgos, N., editors, Simulation and
Synthesis in Medical Imaging, pages 31–41, Cham.
Springer International Publishing.
Isola, P., Zhu, J.-Y., Zhou, T., and Efros, A. A. (2017).
Image-to-image translation with conditional adversar-
ial networks. In Computer Vision and Pattern Recog-
nition (CVPR), 2017 IEEE Conference on.
Jiang, J., Hu, Y.-C., Tyagi, N., Zhang, P., Rimner, A.,
Mageras, G. S., Deasy, J. O., and Veeraraghavan, H.
(2018). Tumor-aware, adversarial domain adaptation
from ct to mri for lung cancer segmentation. In In-
ternational conference on medical image computing
and computer-assisted intervention, pages 777–785.
Springer.
Jin, C.-B., Kim, H., Liu, M., Jung, W., Joo, S., Park, E.,
Ahn, Y. S., Han, I. H., Lee, J. I., and Cui, X. (2019).
Deep ct to mr synthesis using paired and unpaired
data. Sensors, 19(10):2361.
Karras, T., Laine, S., and Aila, T. (2018). A style-based
generator architecture for generative adversarial net-
works. CoRR, abs/1812.04948.
Kavur, A. E., Selver, M. A., Dicle, O., Barıs¸, M., and Gezer,
N. S. (2019). CHAOS - Combined (CT-MR) Healthy
Abdominal Organ Segmentation Challenge Data.
Kida, S., Kaji, S., Nawa, K., Imae, T., Nakamoto, T., Ozaki,
S., Ohta, T., Nozawa, Y., and Nakagawa, K. (2020).
Visual enhancement of cone-beam ct by use of cycle-
gan. Medical physics, 47(3):998–1010.
Kingma, D. P. and Ba, J. (2015). Adam: A method for
stochastic optimization. In Bengio, Y. and LeCun,
Y., editors, 3rd International Conference on Learn-
ing Representations, ICLR 2015, San Diego, CA, USA,
May 7-9, 2015, Conference Track Proceedings.
Kuckertz, S., Papenberg, N., Honegger, J., Morgas, T.,
Haas, B., and Heldmann, S. (2020). Learning de-
formable image registration with structure guidance
constraints for adaptive radiotherapy. In
ˇ
Spiclin,
ˇ
Z.,
McClelland, J., Kybic, J., and Goksel, O., editors,
Biomedical Image Registration, pages 44–53, Cham.
Springer International Publishing.
Kurz, C., Maspero, M., Savenije, M. H., Landry, G., Kamp,
F., Pinto, M., Li, M., Parodi, K., Belka, C., and
Van den Berg, C. A. (2019). Cbct correction us-
ing a cycle-consistent generative adversarial network
and unpaired training to enable photon and proton
dose calculation. Physics in Medicine & Biology,
64(22):225004.
LeCun, Y., Bengio, Y., and Hinton, G. (2015). Deep learn-
ing. nature, 521(7553):436–444.
Liang, X., Chen, L., Nguyen, D., Zhou, Z., Gu, X., Yang,
M., Wang, J., and Jiang, S. (2019). Generating syn-
thesized computed tomography (ct) from cone-beam
computed tomography (cbct) using cyclegan for adap-
tive radiation therapy. Physics in Medicine & Biology,
64(12):125002.
Lu, J.,
¨
Ofverstedt, J., Lindblad, J., and Sladoje, N. (2021).
Is image-to-image translation the panacea for multi-
modal image registration? a comparative study. arXiv
preprint arXiv:2103.16262.
Mahapatra, D., Antony, B., Sedai, S., and Garnavi, R.
(2018). Deformable medical image registration us-
ing generative adversarial networks. In 2018 IEEE
15th International Symposium on Biomedical Imaging
(ISBI 2018), pages 1449–1453. IEEE.
McKenzie, E., Santhanam, A., Ruan, D., O’Connor, D.,
Cao, M., and Sheng, K. (2019). Multimodality image
registration in the head-and-neck using a deep learn-
ing derived synthetic ct as a bridge. Medical Physics,
47.
Modersitzki, J. (2009). Fair: Flexible Algorithms for Im-
age Registration. Society for Industrial and Applied
Mathematics, USA.
Qi, X. S. (2017). Image-Guided Radiation Therapy, pages
131–173. Springer International Publishing, Cham.
Reed, S., Akata, Z., Yan, X., Logeswaran, L., Schiele, B.,
and Lee, H. (2016). Generative adversarial text to
image synthesis. In Balcan, M. F. and Weinberger,
K. Q., editors, Proceedings of The 33rd International
Conference on Machine Learning, volume 48 of Pro-
ceedings of Machine Learning Research, pages 1060–
1069, New York, New York, USA. PMLR.
Roy, S., Carass, A., Jog, A., Prince, J., and Lee, J. (2014).
Mr to ct registration of brains using image synthesis.
Proceedings of SPIE, 9034.
Saiti, E. and Theoharis, T. (2020). An application inde-
pendent review of multimodal 3d registration meth-
ods. Computers & Graphics, 91:153–178.
Shi, Z., Mettes, P., Zheng, G., and Snoek, C. (2021).
Frequency-supervised mr-to-ct image synthesis.
Sørensen, T. J. (1948). A method of establishing groups of
equal amplitude in plant sociology based on similarity
of species content and its application to analyses of
the vegetation on Danish commons, volume 5.
Tanner, C., Ozdemir, F., Profanter, R., Vishnevsky, V.,
Konukoglu, E., and Goksel, O. (2018). Generative
adversarial networks for mr-ct deformable image reg-
istration.
Wei, D., Ahmad, S., Huo, J., Peng, W., Ge, Y., Xue, Z.,
Yap, P.-T., Li, W., Shen, D., and Wang, Q. (2019).
Synthesis and inpainting-based mr-ct registration for
image-guided thermal ablation of liver tumors. In In-
ternational Conference on Medical Image Computing
and Computer-Assisted Intervention, pages 512–520.
Springer.
Wolterink, J. M., Dinkla, A. M., Savenije, M. H. F.,
Seevinck, P. R., van den Berg, C. A. T., and Isgum,
I. (2017). Deep MR to CT synthesis using unpaired
data. CoRR, abs/1708.01155.
Yi, Z., Zhang, H., Tan, P., and Gong, M. (2017). Dualgan:
Unsupervised dual learning for image-to-image trans-
lation.
Zhu, J.-Y., Park, T., Isola, P., and Efros, A. A. (2017).
Unpaired image-to-image translation using cycle-
consistent adversarial networks. In Computer Vision
(ICCV), 2017 IEEE International Conference on.
Evaluating the Effects of a Priori Deep Learning Image Synthesis on Multi-Modal MR-to-CT Image Registration Performance
329