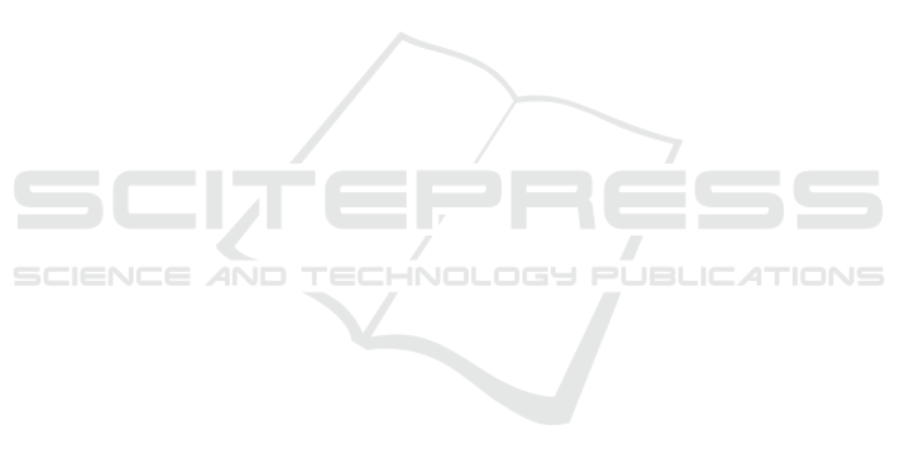
of the IEEE/CVF conference on computer vision and
pattern recognition, pages 9592–9600.
Chalapathy, R. and Chawla, S. (2019). Deep learning
for anomaly detection: A survey. arXiv preprint
arXiv:1901.03407.
Cohen, N. and Hoshen, Y. (2020). Sub-image anomaly
detection with deep pyramid correspondences. arXiv
preprint arXiv:2005.02357.
d’Ascoli, S., Touvron, H., Leavitt, M. L., Morcos, A. S.,
Biroli, G., and Sagun, L. (2021). Convit: Improv-
ing vision transformers with soft convolutional induc-
tive biases. In International Conference on Machine
Learning, pages 2286–2296. PMLR.
Defard, T., Setkov, A., Loesch, A., and Audigier, R. (2021).
Padim: a patch distribution modeling framework for
anomaly detection and localization. In International
Conference on Pattern Recognition, pages 475–489.
Springer.
Deng, J., Dong, W., Socher, R., Li, L.-J., Li, K., and Fei-
Fei, L. (2009). Imagenet: A large-scale hierarchical
image database. In 2009 IEEE conference on com-
puter vision and pattern recognition, pages 248–255.
Ieee.
DeVries, T. and Taylor, G. W. (2017). Improved regular-
ization of convolutional neural networks with cutout.
arXiv preprint arXiv:1708.04552.
Di Mattia, F., Galeone, P., De Simoni, M., and Ghelfi, E.
(2019). A survey on gans for anomaly detection. arXiv
preprint arXiv:1906.11632.
Dosovitskiy, A., Beyer, L., Kolesnikov, A., Weissenborn,
D., Zhai, X., Unterthiner, T., Dehghani, M., Minderer,
M., Heigold, G., Gelly, S., et al. (2020). An image is
worth 16x16 words: Transformers for image recogni-
tion at scale. arXiv preprint arXiv:2010.11929.
Fernando, T., Gammulle, H., Denman, S., Sridharan, S.,
and Fookes, C. (2021). Deep learning for medical
anomaly detection–a survey. ACM Computing Sur-
veys (CSUR), 54(7):1–37.
Hassani, A., Walton, S., Shah, N., Abuduweili, A., Li,
J., and Shi, H. (2021). Escaping the big data
paradigm with compact transformers. arXiv preprint
arXiv:2104.05704.
Kong, Y., Huang, J., Huang, S., Wei, Z., and Wang, S.
(2019). Learning spatiotemporal representations for
human fall detection in surveillance video. Journal
of Visual Communication and Image Representation,
59:215–230.
Li, C.-L., Sohn, K., Yoon, J., and Pfister, T. (2021). Cut-
paste: Self-supervised learning for anomaly detection
and localization. In Proceedings of the IEEE/CVF
Conference on Computer Vision and Pattern Recog-
nition, pages 9664–9674.
Liu, W., Li, R., Zheng, M., Karanam, S., Wu, Z., Bhanu, B.,
Radke, R. J., and Camps, O. (2020). Towards visually
explaining variational autoencoders. In Proceedings
of the IEEE/CVF Conference on Computer Vision and
Pattern Recognition, pages 8642–8651.
Liu, Y., Li, C.-L., and P
´
oczos, B. (2018). Classifier two
sample test for video anomaly detections. In BMVC,
page 71.
Liznerski, P., Ruff, L., Vandermeulen, R. A., Franks,
B. J., Kloft, M., and M
¨
uller, K.-R. (2020). Ex-
plainable deep one-class classification. arXiv preprint
arXiv:2007.01760.
Mahalanobis, P. C. (1936). On the generalized distance in
statistics. National Institute of Science of India.
Masci, J., Meier, U., Cires¸an, D., and Schmidhuber, J.
(2011). Stacked convolutional auto-encoders for hi-
erarchical feature extraction. In International con-
ference on artificial neural networks, pages 52–59.
Springer.
Mishra, P., Verk, R., Fornasier, D., Piciarelli, C., and
Foresti, G. L. (2021). Vt-adl: A vision transformer
network for image anomaly detection and localization.
In 2021 IEEE 30th International Symposium on In-
dustrial Electronics (ISIE), pages 01–06. IEEE.
Mohammadi, B., Fathy, M., and Sabokrou, M. (2021). Im-
age/video deep anomaly detection: A survey. arXiv
preprint arXiv:2103.01739.
Ouardini, K., Yang, H., Unnikrishnan, B., Romain, M.,
Garcin, C., Zenati, H., Campbell, J. P., Chiang, M. F.,
Kalpathy-Cramer, J., Chandrasekhar, V., et al. (2019).
Towards practical unsupervised anomaly detection on
retinal images. In Domain Adaptation and Represen-
tation Transfer and Medical Image Learning with Less
Labels and Imperfect Data, pages 225–234. Springer.
Pang, G., Shen, C., Cao, L., and Hengel, A. V. D. (2021).
Deep learning for anomaly detection: A review. ACM
Computing Surveys (CSUR), 54(2):1–38.
Pirnay, J. and Chai, K. (2022). Inpainting transformer
for anomaly detection. In International Conference
on Image Analysis and Processing, pages 394–406.
Springer.
Rippel, O., Mertens, P., and Merhof, D. (2021). Model-
ing the distribution of normal data in pre-trained deep
features for anomaly detection. In 2020 25th Inter-
national Conference on Pattern Recognition (ICPR),
pages 6726–6733. IEEE.
Schlegl, T., Seeb
¨
ock, P., Waldstein, S. M., Schmidt-Erfurth,
U., and Langs, G. (2017). Unsupervised anomaly de-
tection with generative adversarial networks to guide
marker discovery. In International conference on in-
formation processing in medical imaging, pages 146–
157. Springer.
Tschuchnig, M. E. and Gadermayr, M. (2022). Anomaly
detection in medical imaging-a mini review. Data
Science–Analytics and Applications, pages 33–38.
Wu, H., Xiao, B., Codella, N., Liu, M., Dai, X., Yuan,
L., and Zhang, L. (2021). Cvt: Introducing convo-
lutions to vision transformers. In Proceedings of the
IEEE/CVF International Conference on Computer Vi-
sion, pages 22–31.
Yan, H., Li, Z., Li, W., Wang, C., Wu, M., and Zhang,
C. (2021). Contnet: Why not use convolution and
transformer at the same time? arXiv preprint
arXiv:2104.13497.
Yang, J., Xu, R., Qi, Z., and Shi, Y. (2021). Visual
anomaly detection for images: A survey. arXiv
preprint arXiv:2109.13157.
Yi, J. and Yoon, S. (2020). Patch svdd: Patch-level svdd for
anomaly detection and segmentation. In Proceedings
of the Asian Conference on Computer Vision.
VISAPP 2023 - 18th International Conference on Computer Vision Theory and Applications
152