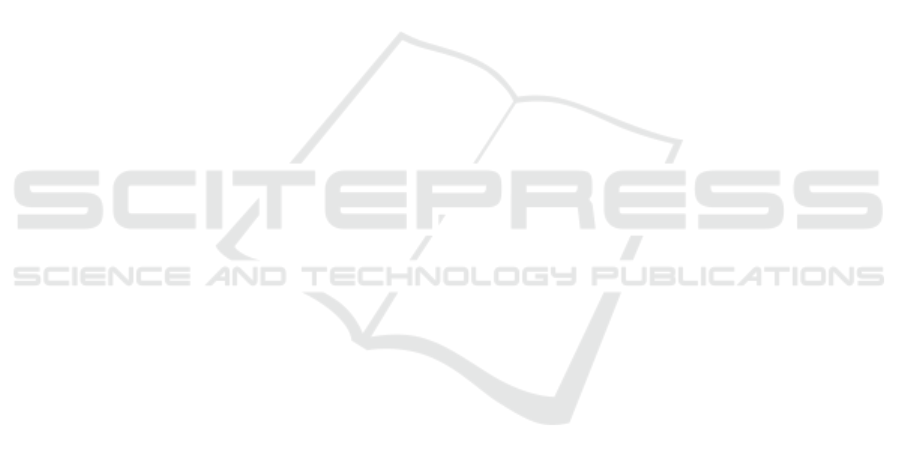
volve a more in-depth sensitive analysis on the param-
eters chosen in the model, and the possibility to ex-
tend the experiments to other different urban contexts
(larger size cities with more transportation modes).
In this case, the possibility of implementing heuris-
tic algorithms cannot be excluded a priori. Under the
mathematical perspective, other variants of the opti-
mization model can be investigated by considering
multitrip, time-dependent travel times and the vari-
ability of the visit duration (dependent of the crowd-
ing level of the visited POIs). Under the managerial
perspective, further studies can be devoted to evalu-
ate the scalability of the proposed model in a regional
context, in which a tourist likes moving between POIs
in different cities using long-distance transportation
modes (bus, train, private car) and where discour-
aging the use of private cars has a stronger impact
on the CO
2
emission levels. Finally, specific studies
can be addressed to the tourist trip design for VRUs
with particular disabilities (for example, people in
wheelchairs or with low vision) who need to access to
POIs, and to transportation modes with specific fea-
tures.
REFERENCES
Abbaspour, R. and Samadzadegan, F. (2009). Itinerary
planning in multimodal urban transportation network.
J Appl Sci, 9(10):1898–1906.
Alonso-Mu
˜
noz, S., Torrej
´
on-Ramos, M., Medina-Salgado,
M., and Gonz
´
alez-S
´
anchez, R. (2022). Sustainabil-
ity as a building block for tourism – future research:
tourism agenda 2030. Tourism Review.
Archetti, C., Speranza, M., and Vigo, D. (2014). Vehicle
routing problems with profits. Vehicle Routing: Prob-
lems, Methods and Applications. SIAM, pages 273–
296.
Ardito, L., Cerchione, R., Del Vecchio, P., and Raguseo, E.
(2019). Big data in smart tourism: challenges, issues
and opportunities. Curr. Issues Tour., 22.
Awerbuch, B., Azar, Y., Blum, A., and Vempala, S. (1998).
New approximation guarantees for minimum-weight
k-trees and prize-collecting salesmen. SIAM Journal
on Computing, 28:254–262.
Butt, S. and Cavalier, T. (1994). A heuristic for the multi-
ple tour maximum collection problem,. Computers &
Operations Research, 21:101–111.
Castillo, L., Armengol, E., Onaind
´
ıa, E., Sebastia, L.,
Gonzalez-Boticario, J., Rodr
´
ıguez, A., Fernandez, S.,
Arias, J., and Borrajo, D. (2008). Samap: an user-
oriented adaptive system for planning tourist visits.
Expert Syst Appl, 34(2):1318–1322.
Collette, Y. and Siarry, P. (2003). Multiobjective Optimiza-
tion. Principles and Case Studies.
Darko, J., Folsom, L., Pugh, N., Park, H., Shirzad, K.,
Owens, J., and Miller, A. (2022). Adaptive personal-
ized routing for vulnerable road users. IET Intelligent
Transport Systems, WILEY., 16.
De Maio, A., Fersini, E., Messina, E., Santoro, F., and Anto-
nio Violi, A. (2020). Exploiting social data for tourism
management: the smartcal project. Quality and Quan-
tity.
Divsalar, G., Ali Divsalar, A., Jabbarzadeh, A., and Sahebi,
H. (2022). An optimization approach for green tourist
trip design. Soft Computing, 26:303–4332.
Fakfare, P. and Wattanacharoensil, W. (2022). Low-
carbon tourism: determining domestic tourist percep-
tion from thailand: Tourism agenda 2030. Tourism
Review.
Gavalas, D., Konstantopoulos, C., Mastakas, K., and
Pantziou, G. (2014). A survey on algorithmic ap-
proaches for solving tourist trip design problems. J
Heuristics., 20(3):291–328.
Geem, Z. W., Tseng, C. L., and Park, Y. (2005). Harmony
search for generalized ori- enteering problem: best
touring in china. In L. Wang, K. Chen and Y.S.Ong
(Eds.), Advances in natural computation. Lecture
Notes in Computer Science, Springer., 3612:741–750.
Golden, B., Levy, L., and Vohra, R. (1987). The orienteer-
ing problem. Naval Research Logistics, 34:307–318.
Gunawan, A., Lau, H., and Vansteenwegen, P. (2016). Ori-
enteering problem: A survey of recent variants, solu-
tion approaches and applications. European Journal
of Operational Research, 255:315–332.
Huang, T., Gong, Y., Zhang, Y., Zhan, Z., and Zhang, J.
(2019). Automatic planning of multiple itineraries: a
niching genetic evolution approach. IEEE Trans Intell
Transp Syst, 21(10):4225–4240.
Ilhan, T., Iravani, S. M. R., and Daskin, M. (2008). The ori-
enteering problem with stochastic profits. IIE Trans-
actions, 40(4):406–421.
Kontogianni, A., Kabassi, K., Virvou, M., and Alepis, E.
(2018). Smart tourism through social network user
modeling: a literature review. 2018 9th International
Conference on Information, Intelligence, Systems and
Applications (IISA). IEEE.
Labadie, N., Mansini, R., Melechovsky, J., and
Wolfler Calvo, R. (2012). The team orienteer-
ing problem with time windows: an lp-based granular
variable neighborhood search. European Journal of
Operational Research, 220(1):15–17.
Laporte, G. and Martello, S. (1990). The selective travel-
ling salesman problem. Discrete Applied Mathemat-
ics, 26:193–207.
Lim, K., Chan, J., Leckie, C., and Karunasekera, S. (2017).
Personalized trip recommendation for tourists based
on user interests, points of interest visit durations and
visit recency. Knowl Inf Syst, 54(2):375–406.
Massidda, C. and Mattana, P. (2013). A svecm analysis
of the relationship between international tourism ar-
rivals, gdp and trade in italy. Journal of Travel Re-
search, 52(1).
Methorst, R. (2002). Vulnerable road users: new ap-
proaches needed. In: 15th ICTCT Workshop, Trans-
port Research Centre, Rotterdam.
The Green Tourist Trip Design Problem with Time Windows: A Model Application on a Urban Scale
69