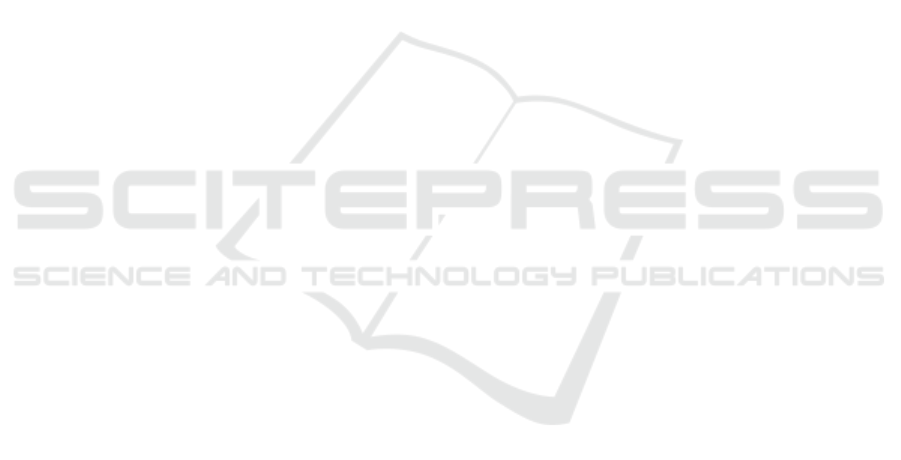
nent failure time distributions, and the scale of the
economy. We use a genetic algorithm to derive the
optimal maintenance plan by minimizing the long-run
operational cost considering both maintenance and
potential penalty costs due to failure in the future. The
model recommends additional preventive operations
in cases with high failure risk. A sensitivity analysis
shows that the optimal decision is mainly affected by
the interest rate and the planning horizon. We evalu-
ated the model’s performance using several real case
problems based on real data from General electric
Healthcare MR machines.
An opportunistic replacement policy (OM) is a
particular type of preventive replacement in which
working components of complex equipment are re-
placed simultaneously with a failed one when a down-
time opportunity has been created (Haque et al.,
2003). Different criteria are developed for compo-
nents’ selection. The age replacement policy is based
on replacing parts when it has achieved their esti-
mated lifetime (Jiang and Ji, 2002). In (Wang et al.,
2021), the authors propose an imperfect opportunistic
maintenance model for two unit series system consid-
ering random repair time. When a unit fails, the other
is maintained when it meets the required age. The ob-
jective is to minimize the average maintenance cost
by optimizing the thresholds of opportunistic mainte-
nance. (Li et al., 2021) studies a maintenance strategy
for wind farms by considering degradation failures
and random incidents. An operating part will only
be replaced if it reaches its critical age. However,
these strategies become complicated if implemented
in a multi-component system, even if it maximizes the
usage of a component. As the number of components
increases, more maintenance activities are conducted,
which may disturb production. Another issue is that
components may fail before their lifetime because of
environmental and external conditions.
Different approaches are developed to consider
the dependency between components for maintenance
planning. The block replacement strategy (BRP) sug-
gests that maintenance activities are conducted on a
block or a group of components. In (Nakagawa and
Zhao, 2013), the authors compared between different
modified BRP models using renewal process where
replacements are executed at constant and random
variable times to minimize the expected cost rates.
They analyze when the random replacements would
be better than the standard BRP. In (Rebaiaia and
Ait-Kadi, 2022), the authors build a model to group
parts based on their mean remaining useful life. In
(Laggoune et al., 2009), the authors focus on main-
tenance cost and suggest that deterioration-based de-
cisions can be included to solve the cost issue. The
model considers economic dependency between com-
ponents by replacing multiple units to minimize sys-
tem downtime. The solution is found by analyzing
the cost or benefit balance of the component that can
be preventively replaced during CM activities. In
(Satow et al., 2000), a model is developed to maxi-
mize the global reliability of n subsystem of k parallel
components that follow Weibull distributions while
considering age conditions and time spent for repa-
ration. Each k parallel items are replaced simulta-
neously. Nevertheless, the BRP policy increases the
wasted components due to the early replacement.
Other researchers evaluate the decision based on
the operation costs. Saranga estimates how cost-
effective opportunistic maintenance is compared to
a later grounding (Saranga, 2004). Using a genetic
algorithm, the model computes the remaining useful
life cost, the down cost, and the cost of risk for each
component individually. In (Nilsson et al., 2009),
the authors study maintenance plan for power plants.
Their results prove the impact of discount interest on
maintenance plan. However, to simplify the model,
they use a discrete-time space to calculate the cost
of failure. In (Haque et al., 2003), the authors de-
velop an optimization model to maximize the net ben-
efit gained from an opportunistic replacement. The
model calculates the system’s residual life with and
without opportunistic operations. The benefit is cal-
culated from savings made in replacement, residual
life, labor time, and the profit of increasing lifetime.
A genetic algorithm was used to solve the model.
It may be realized from the above review that
most of the mathematical models developed so far
are either limited in scope or burdened with exces-
sive computation, especially if the part to repair con-
sists of many deteriorating components. In addition,
few models consider the time needed to disassemble
a component. In addition, because of inflation, the
real value of failure cost may be discounted in the
future, especially for systems with a relatively long
mean useful lifetime.
The formulation of the model is described in Sec-
tion 2. In Section 3, we present a case problem based
on real data. The description of the genetic opera-
tors and the optimization technique is also described
in Section 3. We discuss the results in Section 4. Fi-
nally, Section 5 concludes the paper and highlights
some future research.
Opportunistic Maintenance of Multi-Component Systems Under Structure and Economic Dependencies: A Healthcare System Case Study
159