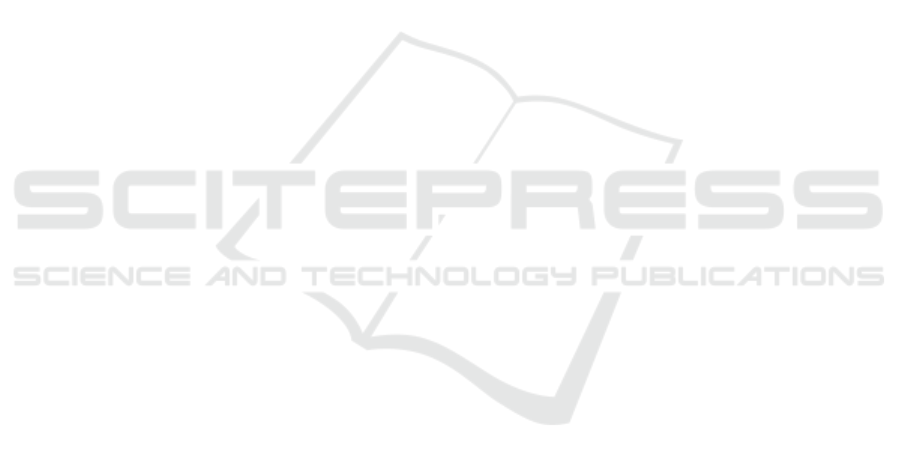
40th Chinese Control Conference (CCC), pages 8181–
8186.
Gerum, R. C., Richter, S., Winterl, A., Mark, C., Fabry, B.,
Le Bohec, C., and Zitterbart, D. P. (2019). Camera-
Transform: A Python package for perspective correc-
tions and image mapping. SoftwareX, 10:1–6.
Harre, I. (2000). Ais adding new quality to vts systems.
Journal of Navigation, 53(3):527–539.
Hu, W.-C., Yang, C.-Y., and Huang, D.-Y. (2011). Ro-
bust real-time ship detection and tracking for vi-
sual surveillance of cage aquaculture. Journal of
Visual Communication and Image Representation,
22(6):543–556.
Kaluza, P., K
¨
olzsch, A., Gastner, M. T., and Blasius, B.
(2010). The complex network of global cargo ship
movements. Journal of the Royal Society Interface,
7(48):1093–1103.
Kang, M., Ji, K., Leng, X., and Lin, Z. (2017). Con-
textual Region-Based Convolutional Neural Network
with Multilayer Fusion for SAR Ship Detection. Re-
mote Sensing, 9(8):1–14.
Larson, K. M., Shand, L., Staid, A., Gray, S., Roesler, E. L.,
and Lyons, D. (2022). An optical flow approach to
tracking ship track behavior using goes-r satellite im-
agery. IEEE Journal of Selected Topics in Applied
Earth Observations and Remote Sensing, 15:6272–
6282.
Last, P., Bahlke, C., Hering-Bertram, M., and Linsen, L.
(2014). Comprehensive Analysis of Automatic Identi-
fication System (AIS) Data in Regard to Vessel Move-
ment Prediction. Journal of Navigation, 67(5):791—-
809.
Li, H. and Man, Y. (2016). Moving ship detection based on
visual saliency for video satellite. In 2016 IEEE Inter-
national Geoscience and Remote Sensing Symposium
(IGARSS), pages 1248–1250.
Lin, T.-Y., Maire, M., Belongie, S., Hays, J., Perona, P., Ra-
manan, D., Doll
´
ar, P., and Zitnick, C. L. (2014). Mi-
crosoft COCO: Common Objects in Context. In Euro-
pean Conference on Computer Vision – ECCV 2014,
pages 740–755. Springer, Springer International Pub-
lishing.
Moosbauer, S., K
¨
onig, D., J
¨
akel, J., and Teutsch, M. (2019).
A Benchmark for Deep Learning Based Object Detec-
tion in Maritime Environments. In Proceedings of the
IEEE/CVF Conference on Computer Vision and Pat-
tern Recognition (CVPR) Workshops, pages 916–925.
Prasad, D. K., Dong, H., Rajan, D., and Quek, C. (2020).
Are object detection assessment criteria ready for mar-
itime computer vision? IEEE Transactions on Intelli-
gent Transportation Systems, 21(12):5295–5304.
Satyanarayanan, M. (2017). The Emergence of Edge Com-
puting. Computer, 50(1):30–39.
Shao, Z., Wu, W., Wang, Z., Du, W., and Li, C. (2018). Sea-
Ships: A Large-Scale Precisely Annotated Dataset for
Ship Detection. IEEE Transactions on Multimedia,
20(10):2593–2604.
Simonsen, C., Theisson, F., Holtskog, Ø., and Gade, R.
(2020). Detecting and locating boats using a ptz
camera with both optical and thermal sensors. In
Farinella, G., Radeva, P., and Braz, J., editors, Pro-
ceedings of the 15th International Joint Conference
on Computer Vision, Imaging and Computer Graph-
ics Theory and Applications, volume 5, pages 395–
403. SCITEPRESS Digital Library. 15th International
Joint Conference on Computer Vision, Imaging and
Computer Graphics Theory and Applications, VISI-
GRAPP 2020 ; Conference date: 27-02-2020 Through
29-02-2020.
Sirimanne, S. N., Hoffmann, J., Asariotis, R., Ayala, G.,
Assaf, M., Bacrot, C., Benamara, H., Chantrel, D.,
Cournoyer, A., Fugazza, M., Hansen, P., Kulaga,
T., Premti, A., Rodr
´
ıguez, L., Salo, B., Tahiri, K.,
Tokuda, H., Ugaz, P., and Youssef, F. (2021). Review
of Maritime Transport 2021. United Nations Con-
ference on Trade and Development (UNCTAD), New
York: United Nations.
Tu, E., Zhang, G., Rachmawati, L., Rajabally, E., and
Huang, G.-B. (2018). Exploiting AIS Data for Intelli-
gent Maritime Navigation: A Comprehensive Survey
From Data to Methodology. IEEE Transactions on In-
telligent Transportation Systems, 19(5):1559–1582.
Wang, N., Wang, Y., and Er, M. J. (2022). Review on
deep learning techniques for marine object recogni-
tion: Architectures and algorithms. Control Engineer-
ing Practice, 118:104458.
Wang, Y., Wang, C., Zhang, H., Dong, Y., and Wei, S.
(2019). Automatic ship detection based on retinanet
using multi-resolution gaofen-3 imagery. Remote
Sensing, 11(5).
Xiaorui, H. and Changchuan, L. (2011). A Preliminary
Study on Targets Association Algorithm of Radar and
AIS Using BP Neural Network. Procedia Engineer-
ing, 15:1441–1445.
Yang, X., Sun, H., Fu, K., Yang, J., Sun, X., Yan, M.,
and Guo, Z. (2018). Automatic Ship Detection in Re-
mote Sensing Images from Google Earth of Complex
Scenes Based on Multiscale Rotation Dense Feature
Pyramid Networks. Remote Sensing, 10(1):1–14.
Zhang, R., Li, S., Ji, G., Zhao, X., Li, J., and Pan, M. (2021).
Survey on deep learning-based marine object detec-
tion. Journal of Advanced Transportation.
Zhao, H., Zhang, W., Sun, H., and Xue, B. (2019). Embed-
ded deep learning for ship detection and recognition.
Future Internet, 11(2).
Maritime Surveillance by Multiple Data Fusion: An Application Based on Deep Learning Object Detection, AIS Data and Geofencing
855