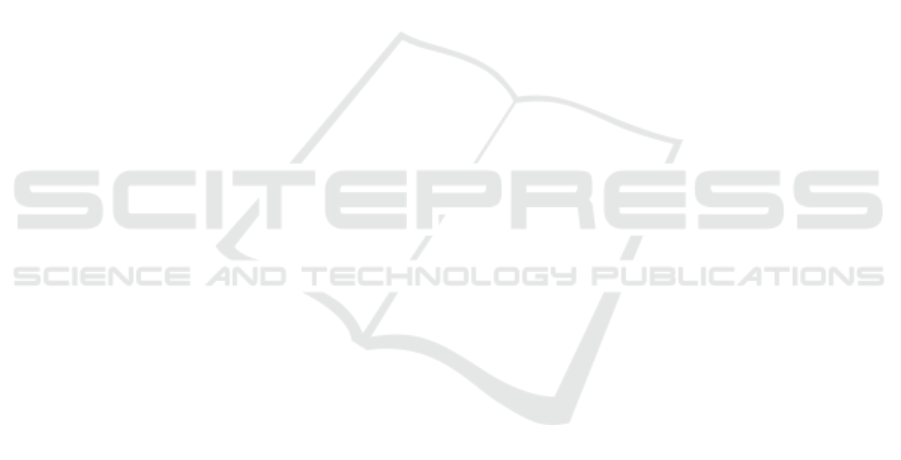
A. (2004). Thyroid nodule shape and prediction of
malignancy. Thyroid, 14(11):953–958.
Chattopadhay, A., Sarkar, A., Howlader, P., and Balasub-
ramanian, V. N. (2018). Grad-cam++: Generalized
gradient-based visual explanations for deep convolu-
tional networks. In 2018 IEEE Winter Conference on
Applications of Computer Vision (WACV). IEEE.
Chi, J., Walia, E., Babyn, P., Wang, J., Groot, G., and
Eramian, M. (2017). Thyroid nodule classification in
ultrasound images by fine-tuning deep convolutional
neural network. Journal of Digital Imaging, 30.
Desai, S. and Ramaswamy, H. G. (2020). Ablation-cam: Vi-
sual explanations for deep convolutional network via
gradient-free localization. In 2020 IEEE Winter Con-
ference on Applications of Computer Vision (WACV),
pages 972–980.
He, K., Zhang, X., Ren, S., and Sun, J. (2015). Deep
residual learning for image recognition. CoRR,
abs/1512.03385.
Koh, J., Lee, E., Han, K., Kim, E.-K., Son, E., Sohn, Y.-
M., Seo, M., Kwon, M.-R., Yoon, J. H., Lee, J., Park,
Y. M., Kim, S., Shin, J., and Kwak, J. (2020a). Di-
agnosis of thyroid nodules on ultrasonography by a
deep convolutional neural network. Scientific reports,
10:15245.
Koh, P. W., Nguyen, T., Tang, Y. S., Mussmann, S., Pierson,
E., Kim, B., and Liang, P. (2020b). Concept bottle-
neck models.
Kwon, S., Choi, I., Kang, J., Jang, W., Lee, G., and Lee, M.
(2020). Ultrasonographic thyroid nodule classification
using a deep convolutional neural network with surgi-
cal pathology (bmvc2020 oral). In Journal of digital
imaging, volume 33, pages 1202–1208.
Lee, E., Ha, H., Kim, H., Moon, H., Byon, J., Huh, S., Son,
J., Yoon, J., Han, K., and Kwak, J. (2019). Differen-
tiation of thyroid nodules on us using features learned
and extracted from various convolutional neural net-
works. Scientific Reports, 9:19854.
Liang, X., Yu, J., Liao, J., and Chen, Z. (2020). Convolu-
tional neural network for breast and thyroid nodules
diagnosis in ultrasound imaging. BioMed Research
International, 2020:1–9.
Lin, M., Chen, Q., and Yan, S. (2013). Network in network.
10.48550/ARXIV.1312.4400.
Liu, S. and Deng, W. (2015). Very deep convolutional
neural network based image classification using small
training sample size. In 2015 3rd IAPR Asian Confer-
ence on Pattern Recognition (ACPR), pages 730–734.
Marcos, D., Fong, R., Lobry, S., Flamary, R., Courty,
N., and Tuia, D. (2020). Contextual semantic inter-
pretability. In Proceedings of the Asian Conference
on Computer Vision.
Morris, L. G., Sikora, A. G., Tosteson, T. D., and Davies, L.
(2013). The increasing incidence of thyroid cancer:
the influence of access to care. Thyroid, 23(7):885–
891.
Naidu, R., Ghosh, A., Maurya, Y., K, S. R. N., and
Kundu, S. S. (2020). Is-cam: Integrated score-cam
for axiomatic-based explanations.
Pedraza, L., Vargas, C., Narv
´
aez, F., Dur
´
an, O., Mu
˜
noz, E.,
and Romero, E. (2015). An open access thyroid ultra-
sound image database. In 10th International Sympo-
sium on Medical Information Processing and Analy-
sis, volume 9287, page 92870W. International Society
for Optics and Photonics.
Rudin, C. (2019). Stop explaining black box machine learn-
ing models for high stakes decisions and use inter-
pretable models instead. Nature Machine Intelligence,
1(5):206–215.
Schaffer, J. (2015). What not to multiply without necessity.
Australasian Journal of Philosophy, 93(4):644–664.
Selvaraju, R. R., Cogswell, M., Das, A., Vedantam, R.,
Parikh, D., and Batra, D. (2019). Grad-CAM: Visual
explanations from deep networks via gradient-based
localization. International Journal of Computer Vi-
sion, 128(2):336–359.
Siegel, R. L., Miller, K. D., and Jemal, A. (2019). Cancer
statistics, 2019. CA: a cancer journal for clinicians,
69(1):7–34.
Simonyan, K., Vedaldi, A., and Zisserman, A. (2013).
Deep inside convolutional networks: Visualising im-
age classification models and saliency maps. arXiv
preprint arXiv:1312.6034.
Speith, T. (2022). A review of taxonomies of explainable
artificial intelligence (xai) methods. In 2022 ACM
Conference on Fairness, Accountability, and Trans-
parency, pages 2239–2250.
Tessler, F. N., Middleton, W. D., Grant, E. G., Hoang, J. K.,
Berland, L. L., Teefey, S. A., Cronan, J. J., Beland,
M. D., Desser, T. S., Frates, M. C., et al. (2017). Acr
thyroid imaging, reporting and data system (ti-rads):
white paper of the acr ti-rads committee. Journal of
the American college of radiology, 14(5):587–595.
Wang, H., Naidu, R., Michael, J., and Kundu, S. S. (2020).
Ss-cam: Smoothed score-cam for sharper visual fea-
ture localization.
Wang, H., Wang, Z., Du, M., Yang, F., Zhang, Z., Ding, S.,
Mardziel, P., and Hu, X. (2019). Score-cam: Score-
weighted visual explanations for convolutional neural
networks.
Wang, L., Zhou, X., Nie, X., Lin, X., Li, J., Zheng, H., Xue,
E., Chen, S., Chen, C., Du, M., Tong, T., Gao, Q., and
Zheng, M. (2022). A multi-scale densely connected
convolutional neural network for automated thyroid
nodule classification. Frontiers in Neuroscience, 16.
Wu, M.-H., Chen, C.-N., Chen, K.-Y., Ho, M.-C., Tai, H.-
C., Wang, Y.-H., Chen, A., and Chang, K.-J. (2016).
Quantitative analysis of echogenicity for patients with
thyroid nodules. Scientific reports, 6:35632.
Zhou, B., Khosla, A., Lapedriza,
`
A., Oliva, A., and Tor-
ralba, A. (2015). Learning deep features for discrimi-
native localization. CoRR, abs/1512.04150.
Prediction of Thyroid Malignancy Using Contextual Semantic Interpretability from Sonograms
101