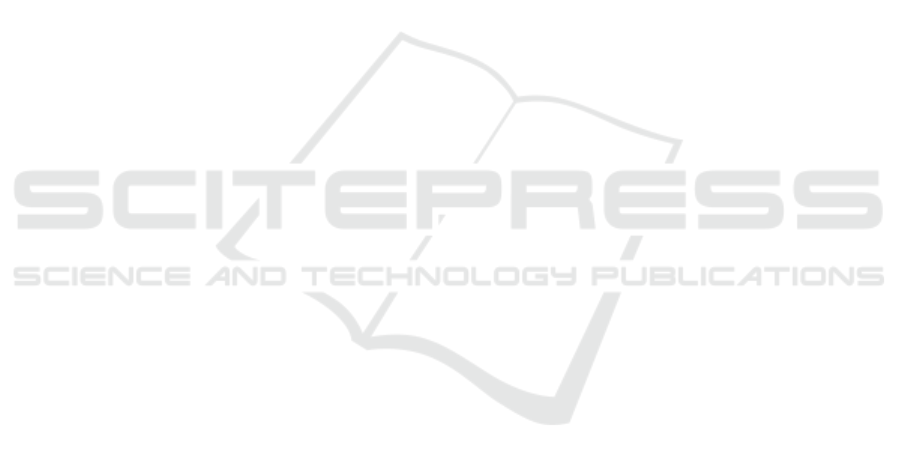
De Jong, J. D., Westert, G. P., Lagoe, R., and Groenewe-
gen, P. P. (2006). Variation in hospital length of stay:
do physicians adapt their length of stay decisions to
what is usual in the hospital where they work? Health
Services Research, 41(2):374–394.
Dorogush, A. V., Ershov, V., and Gulin, A. (2018). Cat-
boost: gradient boosting with categorical features sup-
port. ArXiv, abs/1810.11363.
Feng, C., Hongyue, W., Lu, N., Chen, T., He, H., Lu, Y.,
and Tu, X. (2014). Log-transformation and its impli-
cations for data analysis. Shanghai archives of psychi-
atry, 26:105–9.
Gentimis, T., Alnaser, A. J., Durante, A., Cook,
K., and Steele, R. (2017). Predicting hospital
length of stay using neural networks on mimic
iii data. 2017 IEEE 15th Intl Conf on De-
pendable, Autonomic and Secure Computing, 15th
Intl Conf on Pervasive Intelligence and Comput-
ing, 3rd Intl Conf on Big Data Intelligence and
Computing and Cyber Science and Technology
Congress(DASC/PiCom/DataCom/CyberSciTech).
Johnsen, A., Bulgarelli, L., Horng, S., Celi, L. A.,
and Mark, R. (2021). Mimic-iv (version 1.0).
https://doi.org/10.13026/s6n6-xd98.
Launay, C., Rivi
`
ere, H., Kabeshova, A., and Beauchet, O.
(2015). Predicting prolonged length of hospital stay
in older emergency department users: use of a novel
analysis method, the artificial neural network. Euro-
pean journal of internal medicine, 26(7):478–482.
Rengasamy, D., Rothwell, B., and Figueredo, G. P. (2020).
Asymmetric loss functions for deep learning early
predictions of remaining useful life in aerospace gas
turbine engines. 2020 International Joint Conference
on Neural Networks (IJCNN), page 1–7.
Rocheteau, E., Li
`
o, P., and Hyland, S. (2021). Temporal
pointwise convolutional networks for length of stay
prediction in the intensive care unit. Proceedings of
the Conference on Health, Inference, and Learning.
Sadler, B. L., Berry, L. L., Guenther, R., Hamilton, D. K.,
Hessler, F. A., Merritt, C., and Parker, D. (2011). Fa-
ble hospital 2.0: the business case for building bet-
ter health care facilities. Hastings Center Report,
41(1):13–23.
Safaei, N., Safaei, B., Seyedekrami, S., Talafidaryani, M.,
Masoud, A., Wang, S., Li, Q., and Moqri, M. (2022).
E-catboost: An efficient machine learning framework
for predicting icu mortality using the eicu collabora-
tive research database. PLOS ONE, 17(5).
Stone, K., Zwiggelaar, R., Jones, P., and Mac Parthal
´
ain,
N. (2022). A systematic review of the prediction of
hospital length of stay: Towards a unified framework.
PLOS Digital Health, 1(4):e0000017.
Stone, K., Zwiggelaar, R., Jones, P., and Parthal
´
ain, N. M.
(2019). Predicting hospital length of stay for accident
and emergency admissions. In UK Workshop on Com-
putational Intelligence, pages 283–295. Springer.
Yu, L., Halalau, A., Dalal, B., Abbas, A. E., Ivascu, F.,
Amin, M., and Nair, G. B. (2021). Machine learning
methods to predict mechanical ventilation and mortal-
ity in patients with covid-19. PLOS ONE, 16(4).
Zebin, T., Rezvy, S., and Chaussalet, T. J. (2019). A deep
learning approach for length of stay prediction in clin-
ical settings from medical records. In 2019 IEEE
Conference on Computational Intelligence in Bioin-
formatics and Computational Biology (CIBCB), pages
1–5. IEEE.
Zolbanin, H. M., Davazdahemami, B., Delen, D., and
Zadeh, A. H. (2020). Data analytics for the sustainable
use of resources in hospitals: predicting the length of
stay for patients with chronic diseases. Information &
Management, page 103282.
Predicting Hospital Length of Stay of Patients Leaving the Emergency Department
131