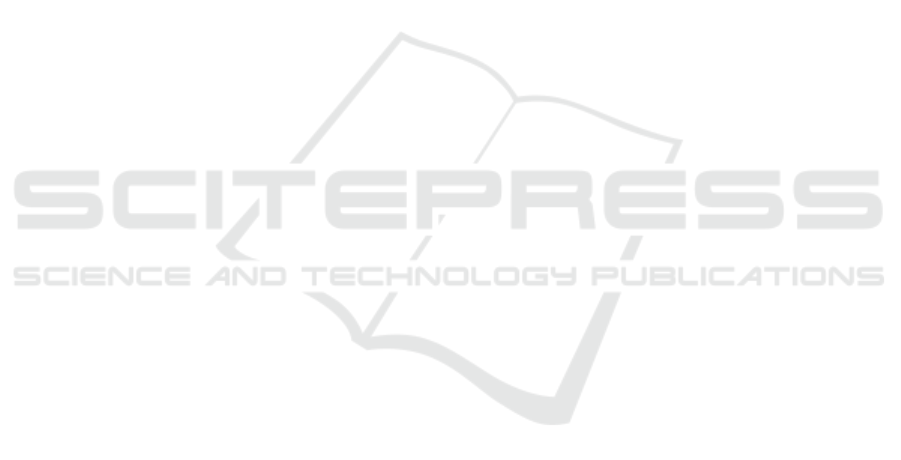
Deep, Y. (2020). Radar cross-sections of pedestrians at au-
tomotive radar frequencies using ray tracing and point
scatterer modelling. IET Radar, Sonar & Navigation,
14:833–844(11).
Doherty, H. G., Cifola, L., Harmanny, R. I. A., and Fio-
ranelli, F. (2019). Unsupervised learning using gen-
erative adversarial networks on micro-doppler spec-
trograms. In 2019 16th European Radar Conference
(EuRAD), pages 197–200.
Dosovitskiy, A., Ros, G., Codevilla, F., Lopez, A., and
Koltun, V. (2017). CARLA: An open urban driving
simulator. In Proceedings of the 1st Annual Confer-
ence on Robot Learning, pages 1–16.
Eder, T., Hachicha, R., Sellami, H., van Driesten, C., and
Biebl, E. (2019). Data driven radar detection models:
A comparison of artificial neural networks and non
parametric density estimators on synthetically gener-
ated radar data. In 2019 Kleinheubach Conference,
pages 1–4.
Erol, B., Gurbuz, S. Z., and Amin, M. G. (2019). Gan-based
synthetic radar micro-doppler augmentations for im-
proved human activity recognition. In 2019 IEEE
Radar Conference (RadarConf), pages 1–5.
Goodfellow, I., Pouget-Abadie, J., Mirza, M., Xu, B.,
Warde-Farley, D., Ozair, S., Courville, A., and Ben-
gio, Y. (2014). Generative adversarial nets. Advances
in neural information processing systems, 27.
Hinton, G. E., Srivastava, N., Krizhevsky, A., Sutskever, I.,
and Salakhutdinov, R. R. (2012). Improving neural
networks by preventing co-adaptation of feature de-
tectors.
Iberle, J., Mutschler, M. A., Scharf, P. A., and Walter, T.
(2019). A radar target simulator concept for close-
range targets with micro-doppler signatures. In 2019
12th German Microwave Conference (GeMiC), pages
198–201.
Javadi, S. H. and Farina, A. (2020). Radar networks: A re-
view of features and challenges. Information Fusion,
61:48–55.
Jiao, L. and Zhao, J. (2019). A survey on the new generation
of deep learning in image processing. IEEE Access,
7:172231–172263.
Kern, N. and Waldschmidt, C. (2022). Data augmentation
in time and doppler frequency domain for radar-based
gesture recognition. In 2021 18th European Radar
Conference (EuRAD), pages 33–36.
Lee, H., Ra, M., and Kim, W.-Y. (2020). Nighttime data
augmentation using gan for improving blind-spot de-
tection. IEEE Access, 8:48049–48059.
Lekic, V. and Babic, Z. (2019). Automotive radar and
camera fusion using generative adversarial networks.
Computer Vision and Image Understanding, 184:1–8.
Liu, G., Wang, L., and Zou, S. (2017). A radar-based
blind spot detection and warning system for driver as-
sistance. In 2017 IEEE 2nd Advanced Information
Technology, Electronic and Automation Control Con-
ference (IAEAC), pages 2204–2208.
Magosi, Z. F., Li, H., Rosenberger, P., Wan, L., and Eich-
berger, A. (2022). A survey on modelling of auto-
motive radar sensors for virtual test and validation of
automated driving. Sensors, 22(15).
MathWorks (2022). Use experiment manager to
train generative adversarial networks (gans). url-
https://www.mathworks.com/help/deeplearning.
Mirza, M. and Osindero, S. (2014a). Conditional generative
adversarial nets.
Mirza, M. and Osindero, S. (2014b). Conditional generative
adversarial nets.
Ngo, A., Bauer, M. P., and Resch, M. (2021). A multi-
layered approach for measuring the simulation-to-
reality gap of radar perception for autonomous driv-
ing.
Qu, L., Wang, Y., Yang, T., Zhang, L., and Sun, Y. (2021).
Wgan-gp-based synthetic radar spectrogram augmen-
tation in human activity recognition. In 2021 IEEE
International Geoscience and Remote Sensing Sym-
posium IGARSS, pages 2532–2535.
Radford, A., Metz, L., and Chintala, S. (2015). Unsuper-
vised representation learning with deep convolutional
generative adversarial networks.
Radford, A., Metz, L., and Chintala, S. (2016). Unsuper-
vised representation learning with deep convolutional
generative adversarial networks.
Rahman, H. (2019). Fundamental Principles of Radar.
Cambridge: Cambridge University Press.
Rahman, M. M., Gurbuz, S. Z., and Amin, M. G. (2021).
Physics-aware design of multi-branch gan for human
rf micro-doppler signature synthesis. In 2021 IEEE
Radar Conference (RadarConf21), pages 1–6.
Rahman, M. M., Malaia, E. A., Gurbuz, A. C., Griffin,
D. J., Crawford, C., and Gurbuz, S. Z. (2022). Ef-
fect of kinematics and fluency in adversarial synthetic
data generation for ASL recognition with RF sensors.
IEEE Transactions on Aerospace and Electronic Sys-
tems, 58(4):2732–2745.
Schuler, K., Becker, D., and Wiesbeck, W. (2008). Extrac-
tion of virtual scattering centers of vehicles by ray-
tracing simulations. IEEE Transactions on Antennas
and Propagation, 56(11):3543–3551.
Skeika, E. L., Luz, M. R. D., Fernandes, B. J. T., Siqueira,
H. V., and De Andrade, M. L. S. C. (2020). Convolu-
tional neural network to detect and measure fetal skull
circumference in ultrasound imaging. IEEE Access,
8:191519–191529.
Song, Y., Wang, Y., and Li, Y. (2019). Radar data simula-
tion using deep generative networks. The Journal of
Engineering, 2019(20):6699–6702.
Vishwakarma, S., Tang, C., Li, W., Woodbridge, K., Adve,
R., and Chetty, K. (2021). Gan based noise generation
to aid activity recognition when augmenting measured
wifi radar data with simulations. In 2021 IEEE Inter-
national Conference on Communications Workshops
(ICC Workshops), pages 1–6.
Wald, S. O. and Weinmann, F. (2019). Ray tracing for
range-doppler simulation of 77 ghz automotive sce-
narios. In 2019 13th European Conference on Anten-
nas and Propagation (EuCAP), pages 1–4.
Wheeler, T. A., Holder, M., Winner, H., and Kochenderfer,
M. (2017). Deep stochastic radar models.
Yun, Z. and Iskander, M. F. (2015). Ray tracing for radio
propagation modeling: Principles and applications.
IEEE Access, 3:1089–1100.
Exploiting GAN Capacity to Generate Synthetic Automotive Radar Data
271