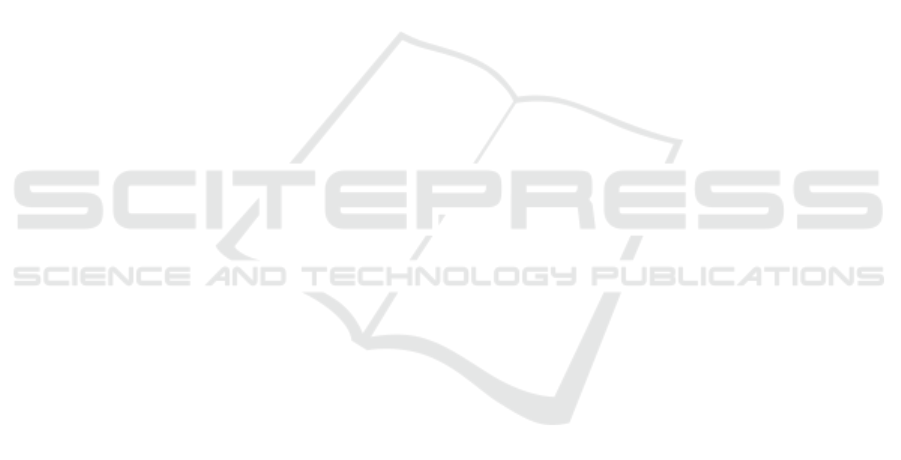
6 CONCLUSIONS
In this paper, we presented DTW
seg
, a modified
DTW algorithm based on a segment-to-point cost
function dedicated to online handwriting matching.
We showed that classical matching approaches such
as RMSE and DTW distance overstate the sam-
pling rate’s importance. DTW
seg
, on the other hand,
matches more closely signals differing in sampling
rates. We also benchmark SoTA for offline to on-
line conversion with DTW
seg
. In future work, we
will study the definition of a loss function (Cuturi and
Blondel, 2018) based on DTW
seg
to train a neural net-
work for the offline to online conversion online task.
We hypothesize that DTW
seg
provides more meaning-
ful information as its gradient pushes the network’s
predictions to be closer to the signal as a whole rather
than a single point from the signal.
REFERENCES
Aksan, E., Pece, F., and Hilliges, O. (2018). DeepWrit-
ing: Making Digital Ink Editable via Deep Generative
Modeling. In Proceedings of the 2018 CHI Confer-
ence on Human Factors in Computing Systems, CHI
’18, pages 1–14, New York, NY, USA. Association
for Computing Machinery.
Archibald, T., Poggemann, M., Chan, A., and Martinez, T.
(2021). TRACE: A Differentiable Approach to Line-
Level Stroke Recovery for Offline Handwritten Text.
In Llad
´
os, J., Lopresti, D., and Uchida, S., editors,
Document Analysis and Recognition – ICDAR 2021,
volume 12823, pages 414–429. Springer International
Publishing, Cham.
Awal, A.-M., Feng, G., Mouch
`
ere, H., and Viard-Gaudin,
C. (2011). First experiments on a new online hand-
written flowchart database. In Agam, G. and Viard-
Gaudin, C., editors, IS&T/SPIE Electronic Imag-
ing, page 78740A, San Francisco Airport, California,
USA.
Bahlmann, C., Haasdonk, B., and Burkhardt, H. (2002).
Online handwriting recognition with support vector
machines - a kernel approach. In Proceedings Eighth
International Workshop on Frontiers in Handwriting
Recognition, pages 49–54.
Chan, C. (2020). Stroke Extraction for Offline Handwritten
Mathematical Expression Recognition. IEEE Access,
8:61565–61575.
Cuturi, M. and Blondel, M. (2018). Soft-DTW: A Differen-
tiable Loss Function for Time-Series.
Diaz, M., Crispo, G., Parziale, A., Marcelli, A., and Ferrer,
M. A. (2022). Writing Order Recovery in Complex
and Long Static Handwriting. International Journal
of Interactive Multimedia and Artificial Intelligence,
7(4):171.
Dinh, M., Yang, H.-J., Lee, G.-S., Kim, S.-H., and Do, L.-
N. (2016). Recovery of drawing order from multi-
stroke English handwritten images based on graph
models and ambiguous zone analysis. Expert Systems
with Applications, 64:352–364.
Graves, A. (2014). Generating Sequences With Recurrent
Neural Networks.
Hassa
¨
ıne, A., Al Maadeed, S., and Bouridane, A. (2013).
ICDAR 2013 Competition on Handwriting Stroke Re-
covery from Offline Data. In 2013 12th International
Conference on Document Analysis and Recognition,
pages 1412–1416.
Ji, B. and Chen, T. (2020). Generative Adversarial Network
for Handwritten Text.
Kato, Y. and Yasuhara, M. (2000). Recovery of drawing
order from single-stroke handwriting images. IEEE
Transactions on Pattern Analysis and Machine Intel-
ligence, 22(9):938–949.
Li, J., Mouchere, H., Viard-Gaudin, C., and Chen, Z.
(2013). A Multi-stroke Dynamic Time Warping Dis-
tance Based on A* Optimization. In 2013 12th In-
ternational Conference on Document Analysis and
Recognition, pages 1330–1334.
Marti, U.-V. and Bunke, H. (2002). The IAM-database:
An English sentence database for offline handwrit-
ing recognition. International Journal on Document
Analysis and Recognition, 5(1):39–46.
Mohamed Moussa, E., Lelore, T., and Mouch
`
ere, H. (2021).
Applying End-to-End Trainable Approach on Stroke
Extraction in Handwritten Math Expressions Images.
In Llad
´
os, J., Lopresti, D., and Uchida, S., editors,
Document Analysis and Recognition – ICDAR 2021,
Lecture Notes in Computer Science, pages 445–458,
Cham. Springer International Publishing.
Phan, D., Na, I.-S., Kim, S.-H., Lee, G.-S., and Yang, H.-J.
(2015). Triangulation Based Skeletonization and Tra-
jectory Recovery for Handwritten Character Patterns.
KSII Transactions on Internet and Information Sys-
tems (TIIS), 9(1):358–377.
Sakoe, H. and Chiba, S. (1978). Dynamic programming
algorithm optimization for spoken word recognition.
IEEE Transactions on Acoustics, Speech, and Signal
Processing, 26(1):43–49.
Sharma, A. and Sundaram, S. (2018). On the Exploration
of Information From the DTW Cost Matrix for Online
Signature Verification. IEEE Transactions on Cyber-
netics, 48(2):611–624.
Szoke, I., Schwarz, P., Matejka, P., Burget, L., Karafiat, M.,
Fapso, M., and Cernocky, J. (2005). Comparison of
keyword spotting approaches for informal continuous
speech. In Interspeech 2005, pages 633–636. ISCA.
Viard-Gaudin, C., Lallican, P. M., Knerr, S., and Binter, P.
(1999). The IRESTE On/Off (IRONOFF) dual hand-
writing database. In Proceedings of the Fifth Interna-
tional Conference on Document Analysis and Recog-
nition. ICDAR ’99 (Cat. No.PR00318), pages 455–
458.
Wehbi, M., Luge, D., Hamann, T., Barth, J., Kaempf, P.,
Zanca, D., and Eskofier, B. M. (2022). Surface-Free
Multi-Stroke Trajectory Reconstruction and Word
Recognition Using an IMU-Enhanced Digital Pen.
Sensors, 22(14):5347.
Point to Segment Distance DTW for Online Handwriting Signals Matching
855